NeRF-Det++: Incorporating Semantic Cues and Perspective-aware Depth Supervision for Indoor Multi-View 3D Detection
arXiv (Cornell University)(2024)
摘要
NeRF-Det has achieved impressive performance in indoor multi-view 3Ddetection by innovatively utilizing NeRF to enhance representation learning.Despite its notable performance, we uncover three decisive shortcomings in itscurrent design, including semantic ambiguity, inappropriate sampling, andinsufficient utilization of depth supervision. To combat the aforementionedproblems, we present three corresponding solutions: 1) Semantic Enhancement. Weproject the freely available 3D segmentation annotations onto the 2D plane andleverage the corresponding 2D semantic maps as the supervision signal,significantly enhancing the semantic awareness of multi-view detectors. 2)Perspective-aware Sampling. Instead of employing the uniform sampling strategy,we put forward the perspective-aware sampling policy that samples densely nearthe camera while sparsely in the distance, more effectively collecting thevaluable geometric clues. 3)Ordinal Residual Depth Supervision. As opposed todirectly regressing the depth values that are difficult to optimize, we dividethe depth range of each scene into a fixed number of ordinal bins andreformulate the depth prediction as the combination of the classification ofdepth bins as well as the regression of the residual depth values, therebybenefiting the depth learning process. The resulting algorithm, NeRF-Det++, hasexhibited appealing performance in the ScanNetV2 and ARKITScenes datasets.Notably, in ScanNetV2, NeRF-Det++ outperforms the competitive NeRF-Det by +1.9in mAP@0.25 and +3.5https://github.com/mrsempress/NeRF-Detplusplus.
更多查看译文
关键词
Multi-View Stereo,Interest Point Detectors
AI 理解论文
溯源树
样例
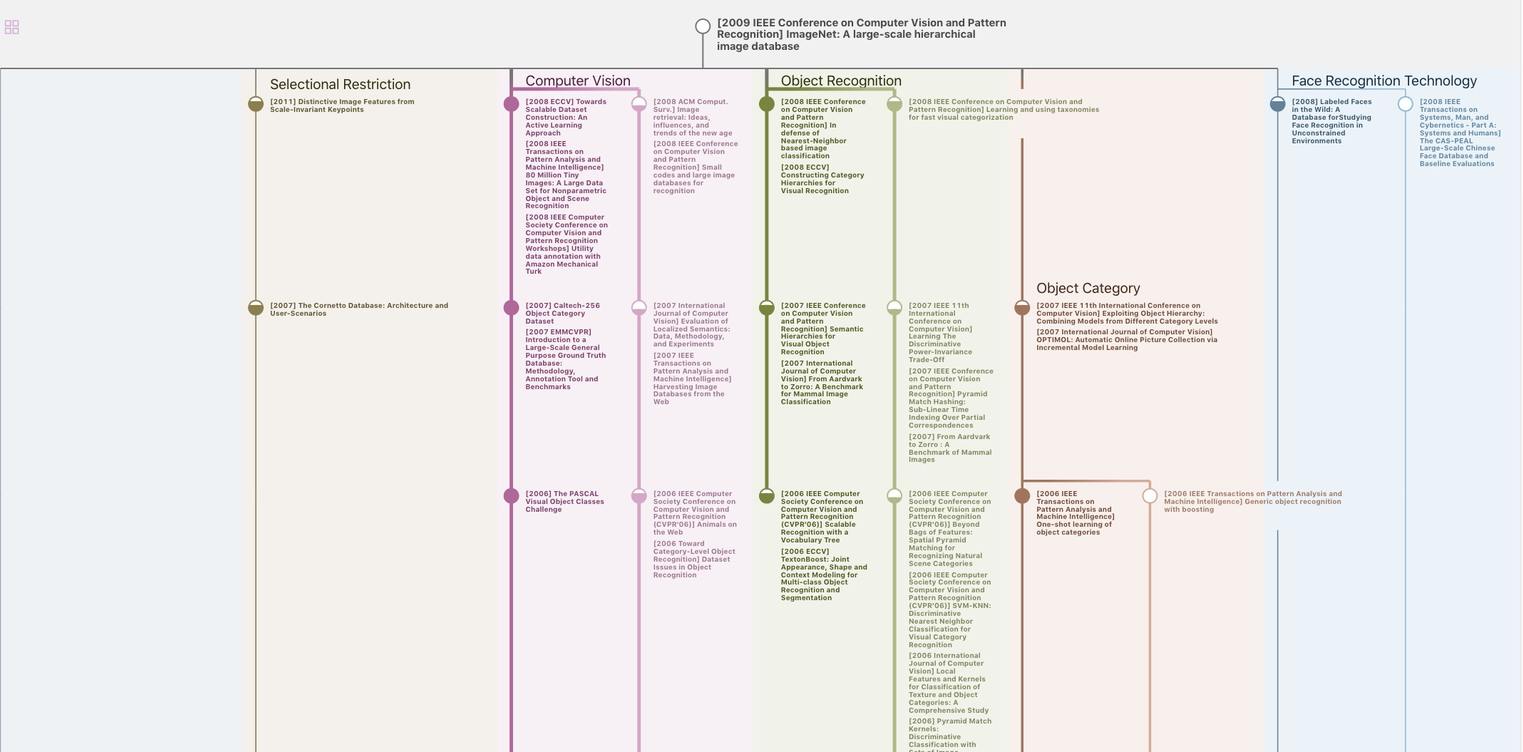
生成溯源树,研究论文发展脉络
Chat Paper
正在生成论文摘要