Causal Imputation for Counterfactual SCMs: Bridging Graphs and Latent Factor Models
arXiv (Cornell University)(2024)
摘要
We consider the task of causal imputation, where we aim to predict theoutcomes of some set of actions across a wide range of possible contexts. As arunning example, we consider predicting how different drugs affect cells fromdifferent cell types. We study the index-only setting, where the actions andcontexts are categorical variables with a finite number of possible values.Even in this simple setting, a practical challenge arises, since often only asmall subset of possible action-context pairs have been studied. Thus, modelsmust extrapolate to novel action-context pairs, which can be framed as a formof matrix completion with rows indexed by actions, columns indexed by contexts,and matrix entries corresponding to outcomes. We introduce a novel SCM-basedmodel class, where the outcome is expressed as a counterfactual, actions areexpressed as interventions on an instrumental variable, and contexts aredefined based on the initial state of the system. We show that, under alinearity assumption, this setup induces a latent factor model over the matrixof outcomes, with an additional fixed effect term. To perform causal predictionbased on this model class, we introduce simple extension to the SyntheticInterventions estimator (Agarwal et al., 2020). We evaluate several matrixcompletion approaches on the PRISM drug repurposing dataset, showing that ourmethod outperforms all other considered matrix completion approaches.
更多查看译文
关键词
Causal imputation,latent factor models,synthetic interventions,matrix completion
AI 理解论文
溯源树
样例
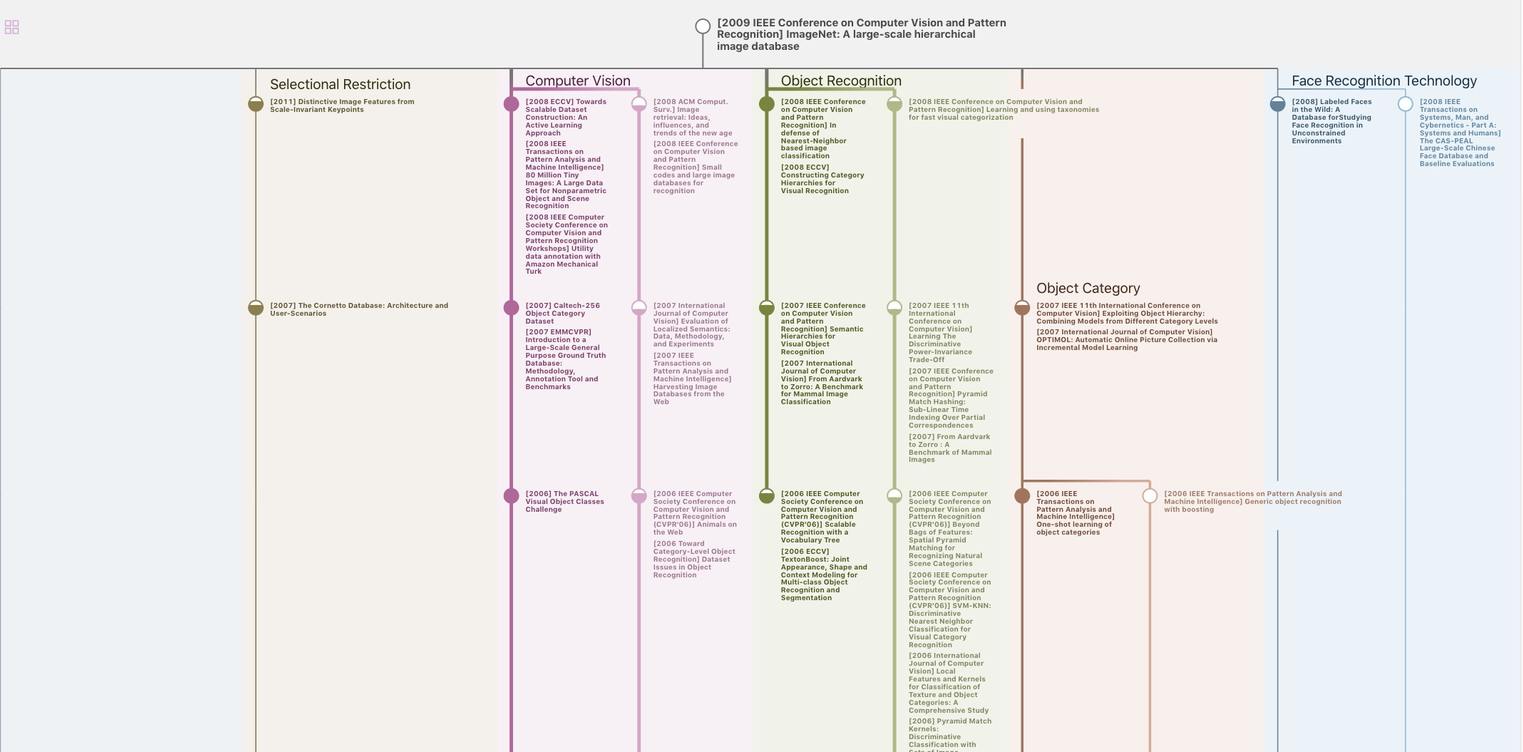
生成溯源树,研究论文发展脉络
Chat Paper
正在生成论文摘要