Deep Homography Estimation for Visual Place Recognition
AAAI 2024(2024)
摘要
Visual place recognition (VPR) is a fundamental task for many applications such as robot localization and augmented reality. Recently, the hierarchical VPR methods have received considerable attention due to the trade-off between accuracy and efficiency. They usually first use global features to retrieve the candidate images, then verify the spatial consistency of matched local features for re-ranking. However, the latter typically relies on the RANSAC algorithm for fitting homography, which is time-consuming and non-differentiable. This makes existing methods compromise to train the network only in global feature extraction. Here, we propose a transformer-based deep homography estimation (DHE) network that takes the dense feature map extracted by a backbone network as input and fits homography for fast and learnable geometric verification. Moreover, we design a re-projection error of inliers loss to train the DHE network without additional homography labels, which can also be jointly trained with the backbone network to help it extract the features that are more suitable for local matching. Extensive experiments on benchmark datasets show that our method can outperform several state-of-the-art methods. And it is more than one order of magnitude faster than the mainstream hierarchical VPR methods using RANSAC. The code is released at https://github.com/Lu-Feng/DHE-VPR.
更多查看译文
关键词
ROB: Localization, Mapping, and Navigation,CV: Applications,CV: Image and Video Retrieval
AI 理解论文
溯源树
样例
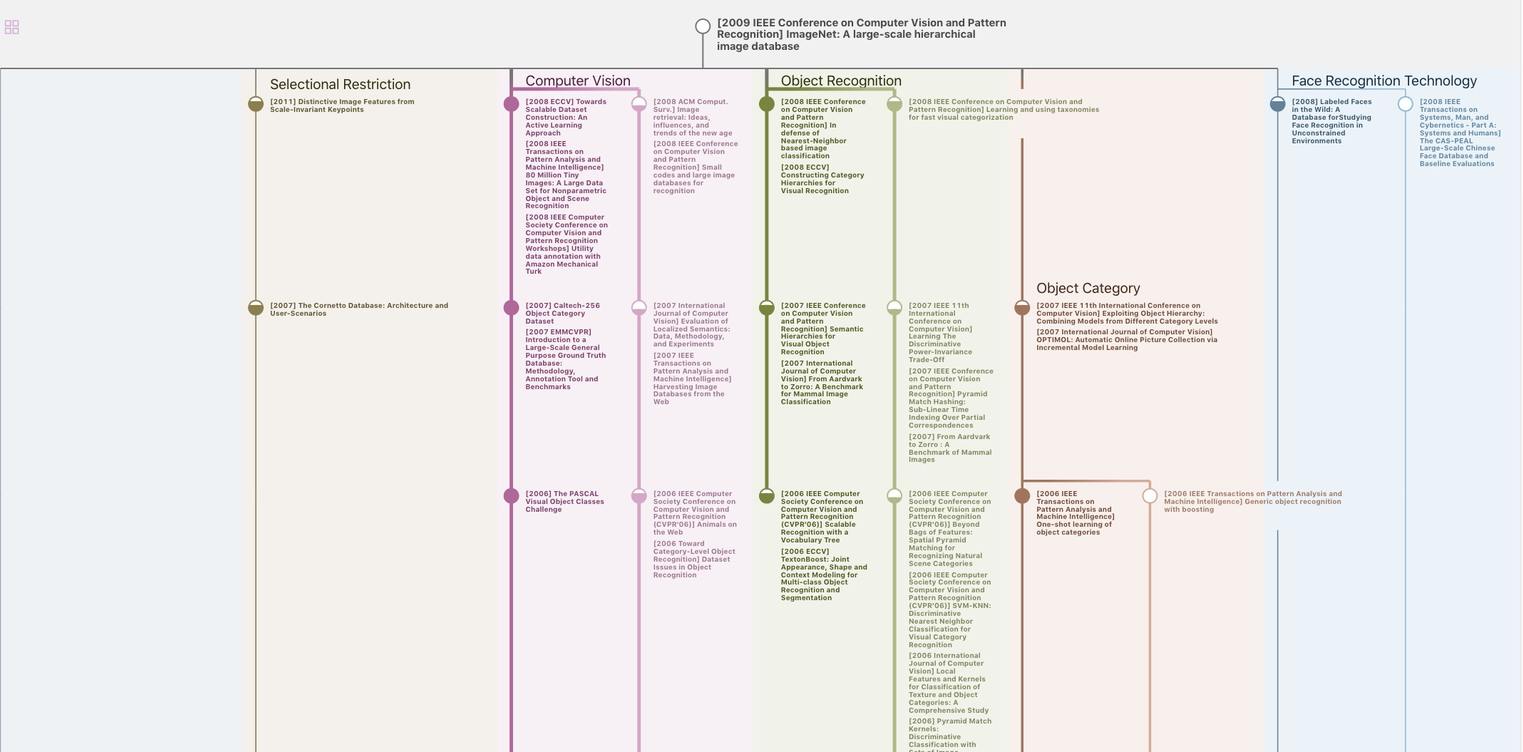
生成溯源树,研究论文发展脉络
Chat Paper
正在生成论文摘要