Data-Efficient Learning via Clustering-Based Sensitivity Sampling: Foundation Models and Beyond
CoRR(2024)
摘要
We study the data selection problem, whose aim is to select a small
representative subset of data that can be used to efficiently train a machine
learning model. We present a new data selection approach based on k-means
clustering and sensitivity sampling. Assuming access to an embedding
representation of the data with respect to which the model loss is Hölder
continuous, our approach provably allows selecting a set of “typical” k +
1/ε^2 elements whose average loss corresponds to the average loss of
the whole dataset, up to a multiplicative (1±ε) factor and an
additive ελΦ_k, where Φ_k represents the k-means
cost for the input embeddings and λ is the Hölder constant.
We furthermore demonstrate the performance and scalability of our approach on
fine-tuning foundation models and show that it outperforms state-of-the-art
methods. We also show how it can be applied on linear regression, leading to a
new sampling strategy that surprisingly matches the performances of leverage
score sampling, while being conceptually simpler and more scalable.
更多查看译文
AI 理解论文
溯源树
样例
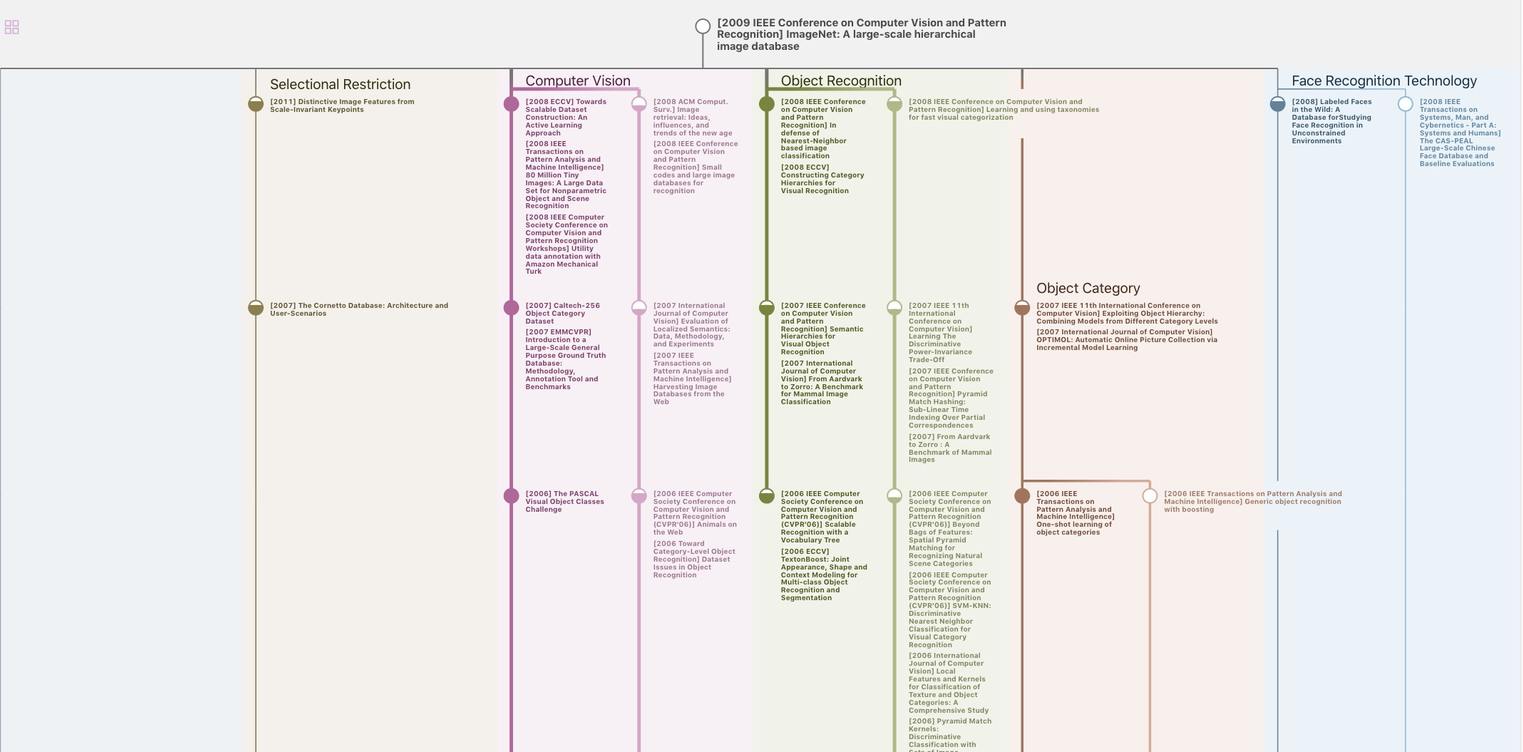
生成溯源树,研究论文发展脉络
Chat Paper
正在生成论文摘要