Learning Invariant Inter-pixel Correlations for Superpixel Generation
AAAI 2024(2024)
摘要
Deep superpixel algorithms have made remarkable strides by substituting hand-crafted features with learnable ones. Nevertheless, we observe that existing deep superpixel methods, serving as mid-level representation operations, remain sensitive to the statistical properties (e.g., color distribution, high-level semantics) embedded within the training dataset. Consequently, learnable features exhibit constrained discriminative capability, resulting in unsatisfactory pixel grouping performance, particularly in untrainable application scenarios. To address this issue, we propose the Content Disentangle Superpixel (CDS) algorithm to selectively separate the invariant inter-pixel correlations and statistical properties, i.e., style noise. Specifically, We first construct auxiliary modalities that are homologous to the original RGB image but have substantial stylistic variations. Then, driven by mutual information, we propose the local-grid correlation alignment across modalities to reduce the distribution discrepancy of adaptively selected features and learn invariant inter-pixel correlations. Afterwards, we perform global-style mutual information minimization to enforce the separation of invariant content and train data styles. The experimental results on four benchmark datasets demonstrate the superiority of our approach to existing state-of-the-art methods, regarding boundary adherence, generalization, and efficiency. Code and pre-trained model are available at https://github.com/rookiie/CDSpixel.
更多查看译文
关键词
CV: Representation Learning for Vision,CV: Applications,CV: Scene Analysis & Understanding,CV: Segmentation,ML: Applications,ML: Deep Learning Algorithms,ML: Deep Learning Theory,ML: Representation Learning
AI 理解论文
溯源树
样例
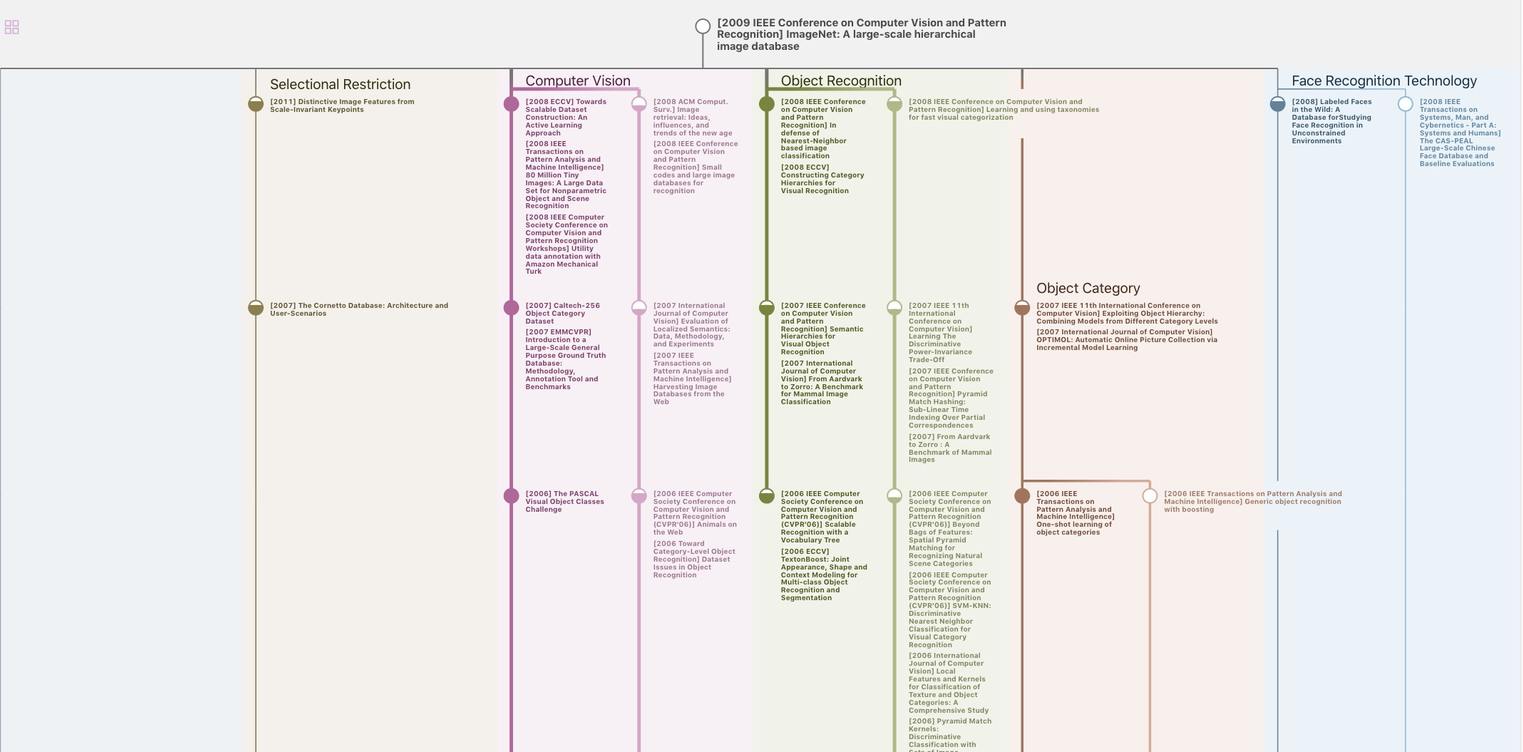
生成溯源树,研究论文发展脉络
Chat Paper
正在生成论文摘要