ROG_PL: Robust Open-Set Graph Learning via Region-Based Prototype Learning
AAAI 2024(2024)
摘要
Open-set graph learning is a practical task that aims to classify the known class nodes and to identify unknown class samples as unknowns. Conventional node classification methods usually perform unsatisfactorily in open-set scenarios due to the complex data they encounter, such as out-of-distribution (OOD) data and in-distribution (IND) noise. OOD data are samples that do not belong to any known classes. They are outliers if they occur in training (OOD noise), and open-set samples if they occur in testing. IND noise are training samples which are assigned incorrect labels. The existence of IND noise and OOD noise is prevalent, which usually cause the ambiguity problem, including the intra-class variety problem and the inter-class confusion problem. Thus, to explore robust open-set learning methods is necessary and difficult, and it becomes even more difficult for non-IID graph data. To this end, we propose a unified framework named ROG_PL to achieve robust open-set learning on complex noisy graph data, by introducing prototype learning. In specific, ROG_PL consists of two modules, i.e., denoising via label propagation and open-set prototype learning via regions. The first module corrects noisy labels through similarity-based label propagation and removes low-confidence samples, to solve the intra-class variety problem caused by noise. The second module learns open-set prototypes for each known class via non-overlapped regions and remains both interior and border prototypes to remedy the inter-class confusion problem. The two modules are iteratively updated under the constraints of classification loss and prototype diversity loss. To the best of our knowledge, the proposed ROG_PL is the first robust open-set node classification method for graph data with complex noise. Experimental evaluations of ROG_PL on several benchmark graph datasets demonstrate that it has good performance.
更多查看译文
关键词
DMKM: Graph Mining, Social Network Analysis & Community,ML: Classification and Regression
AI 理解论文
溯源树
样例
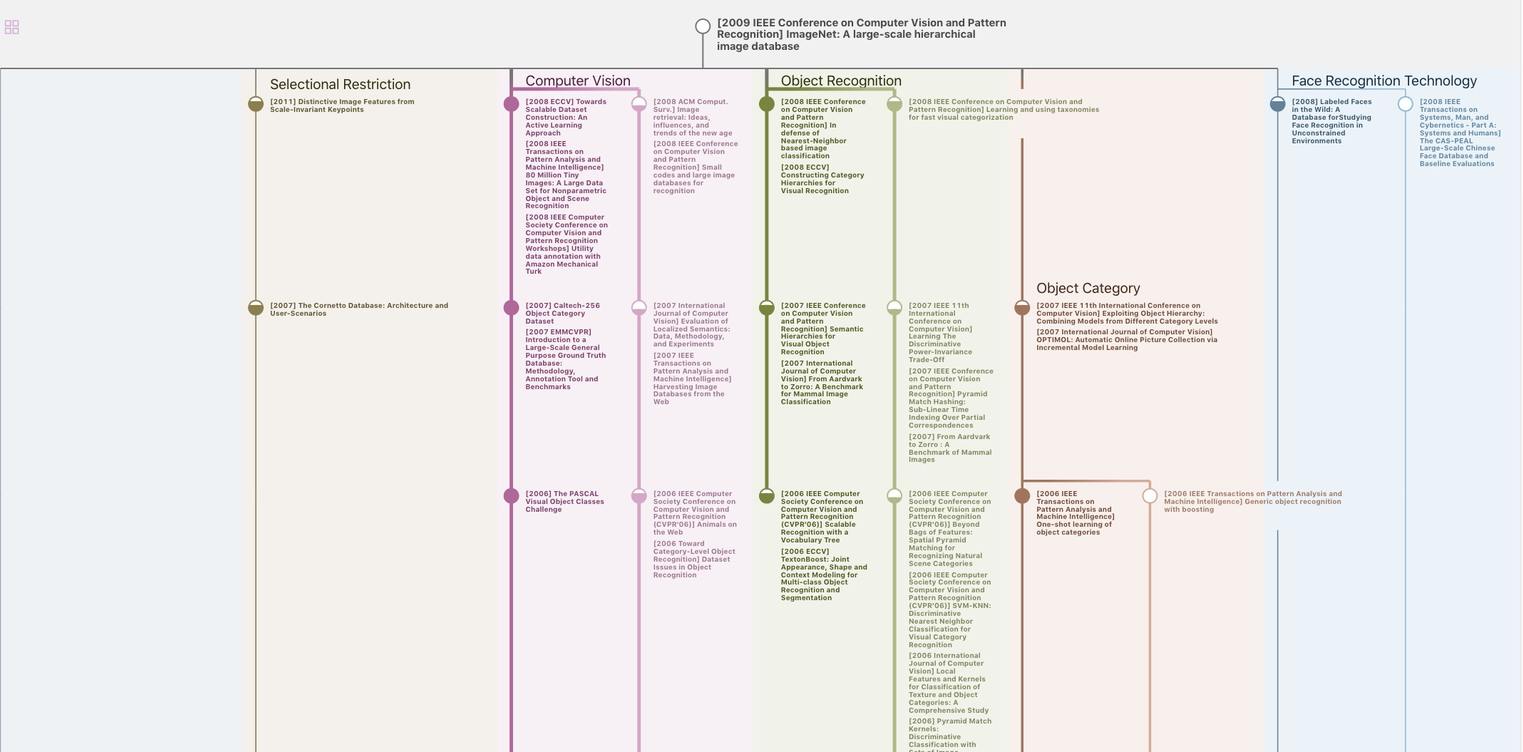
生成溯源树,研究论文发展脉络
Chat Paper
正在生成论文摘要