Inter-Intra Modality Knowledge Learning and Clustering Noise Alleviation for Unsupervised Visible-Infrared Person Re-Identification
IEEE Transactions on Knowledge and Data Engineering(2024)
摘要
Unsupervised visible-infrared person re-identification (USVI-ReID) is a valuable yet under-explored task that addresses the challenge of person retrieval from different modalities without annotations. USVI-ReID is faced with large intra and inter modality discrepancy as well as pseudo label noises. In this paper, we propose a novel learning approach termed Spectrum-Cam Aware and Refined Cross-Pair learning strategy (SCA-RCP), crafted to concurrently tackle these issues. The Dual-Spectrum Augmentation (DSA) method is first presented to mine inter-modality knowledge between the visible and infrared modalities by expanding the diversity of spectra for both modalities. Concurrently, to learn intra-modality knowledge, we divide the clusters into camera-based proxies and introduce the Cross-modal Cam-Aware Proxy learning method (CCAP) to pull together camera-based proxies that correspond to the same identity across modalities. Finally, to mitigate clustering noise, the innovative Refined Cross-Pair learning strategy (RCP) is devised comprising the Intra-modality Label Refinement (ILR) and Bi-directional Cross Pairing (BCP) method. ILR calculates maximum-likely label for each instance by employing the intra-modal cluster memories as a classifier, while BCP establish dependable cross-paired labels in a bidirectional manner. Extensive experiments on the cross-modality datasets demonstrate the superior performance of our model over the state-of-art method.
更多查看译文
关键词
Unsupervised Learning,Visible-Infrared Person Re-Identification,Label Refinement
AI 理解论文
溯源树
样例
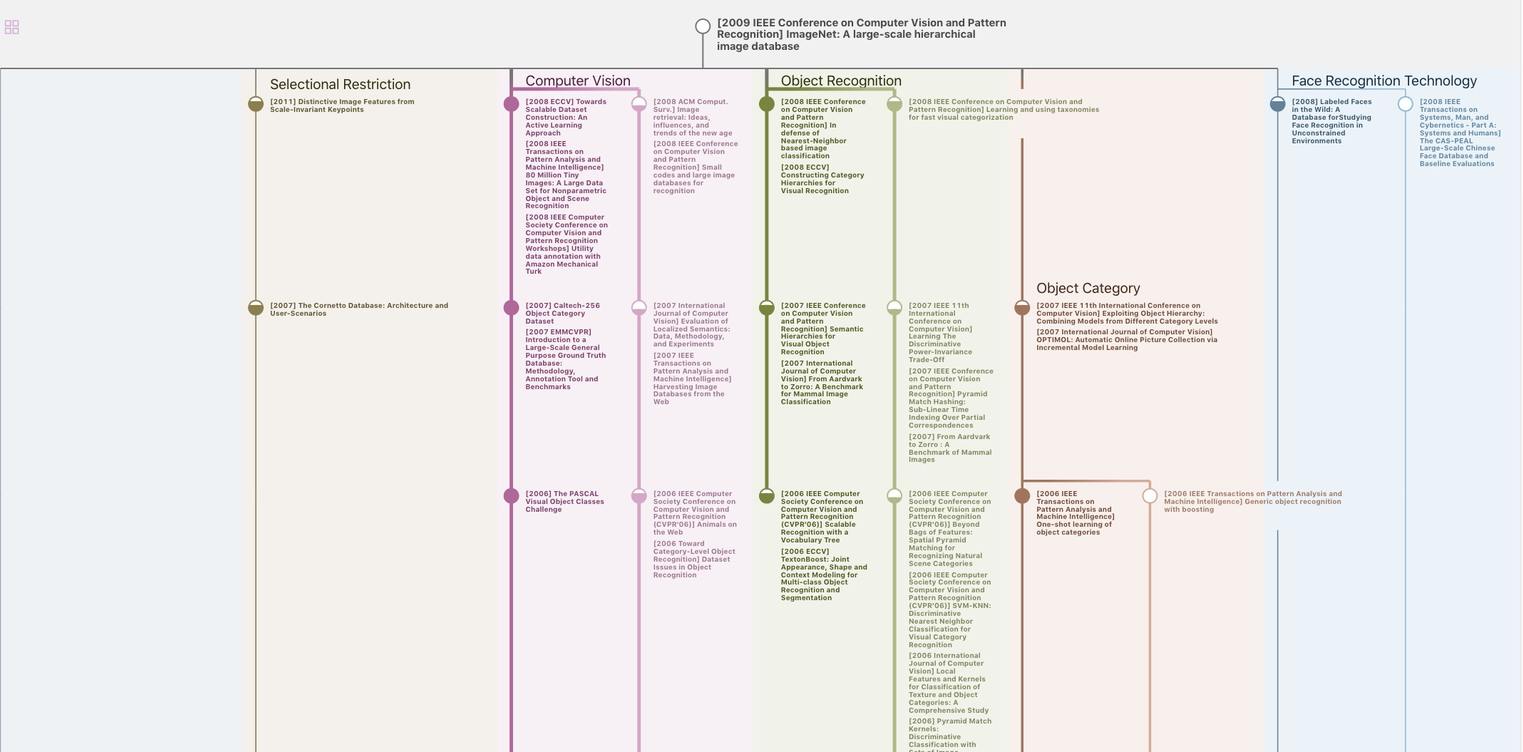
生成溯源树,研究论文发展脉络
Chat Paper
正在生成论文摘要