Learning with Noisy Labels by Semantic and Feature Space Collaboration
IEEE Transactions on Circuits and Systems for Video Technology(2024)
摘要
Learning with noisy labels has become more and more popular because of the expensive costs of collecting high-quality labels. To avoid the decrease in model performance caused by incorrect annotations, some existing methods try to select reliable samples based on the local structure of nearest neighbors in the feature space. However, the information from local neighbors is unreliable when encountering extremely noisy cases, and selecting samples only using the feature space may result in clear noise accumulation. To this end, we propose a Dual-Space Collaborative Learning (DSCL) framework to boost classification accuracy by jointly using the complementarity information from both semantic and feature spaces. Specifically, a collaborative selection module is designed by constructing a set of global prototypes and high-confidence semantic predictions, which enhances the robustness of the sample selection process. Moreover, a collaborative regularization module is constructed by the bidirectional adjustment between the semantic and feature spaces, which effectively alleviates the noise accumulation issue caused by sample selection bias in a single space. By simultaneously utilizing the two modules, our method improves the accuracy of sample selection and mitigates the degradation caused by noisy labels. Extensive experimental results indicate the superior performance of DSCL compared with various baselines.
更多查看译文
关键词
Label noise,collaborative learning,deep learning,image classification
AI 理解论文
溯源树
样例
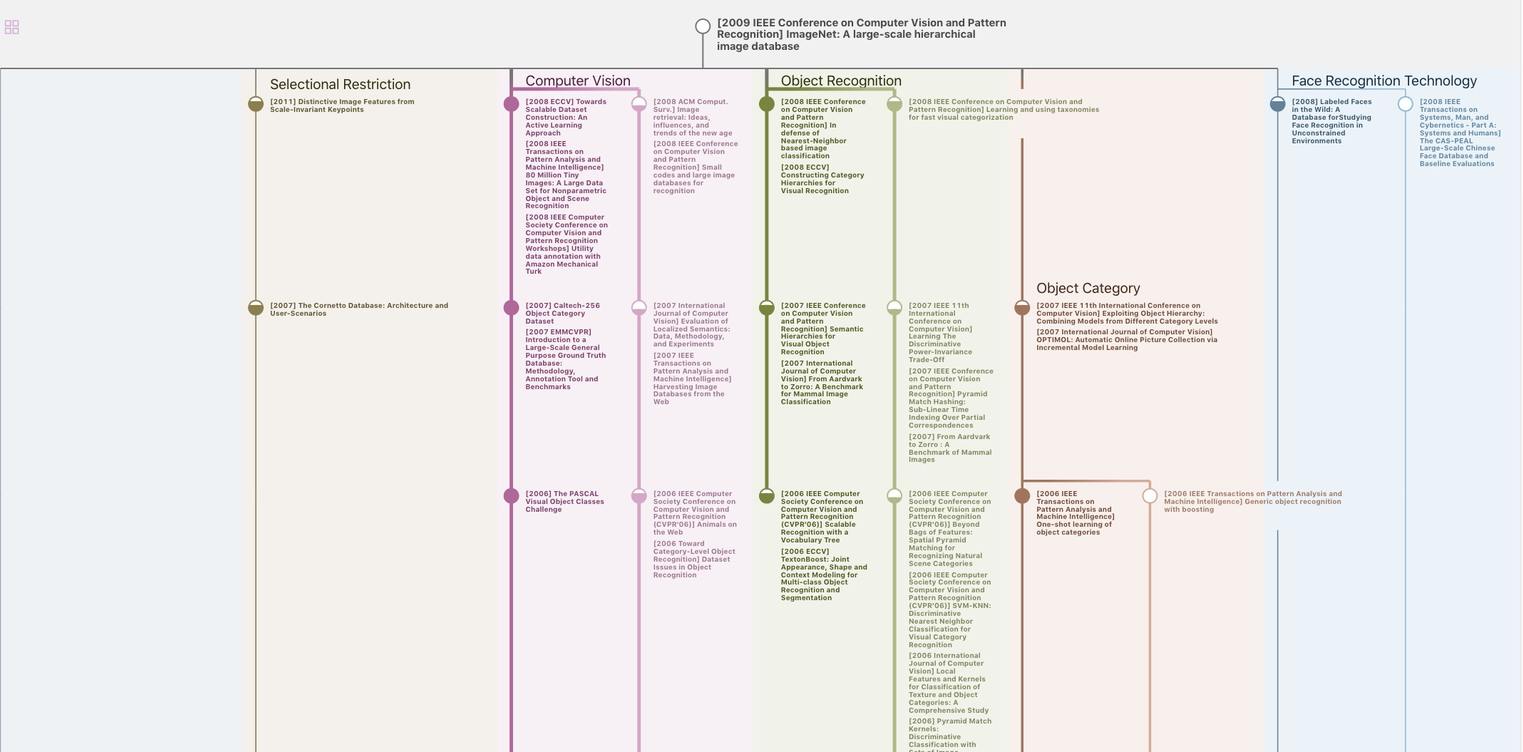
生成溯源树,研究论文发展脉络
Chat Paper
正在生成论文摘要