Be Careful With Writing Perturbations: This Could Be a Trigger of Textual Backdoor Attacks
2023 IEEE Smart World Congress (SWC)(2023)
摘要
Recent research has shown that large natural language processing (NLP) models have been vulnerable to a security threat called backdoor attacks. The current privately-triggered backdoor attack achieves the attack target by modifying the label of the poisoned data to a specified label. Still, the result often ignores the consistency of the sample semantics and the label, thus failing to achieve stealthy attacks on system users and system deployers. To address this issue, we use human-written text perturbations, which are less likely to be detected as machine-manipulation and removed by defense systems, as a backdoor trigger and apply a mild adversarial perturbation to the poisoned samples before implanting the backdoor, thereby addressing the semantic inconsistency caused by modifying the labels of the poisoned data. Furthermore, we use learnable backdoor triggers to improve the stealth of backdoor attacks while avoiding the conflict between textual adversarial perturbations and backdoor implantation. Experiments show that our attack achieves close to 100% attack success rates on SST-2, OLID and AG’s News datasets without affecting the utility of existing NLP models.
更多查看译文
关键词
textual backdoor attacks,natural language processing (NLP) models
AI 理解论文
溯源树
样例
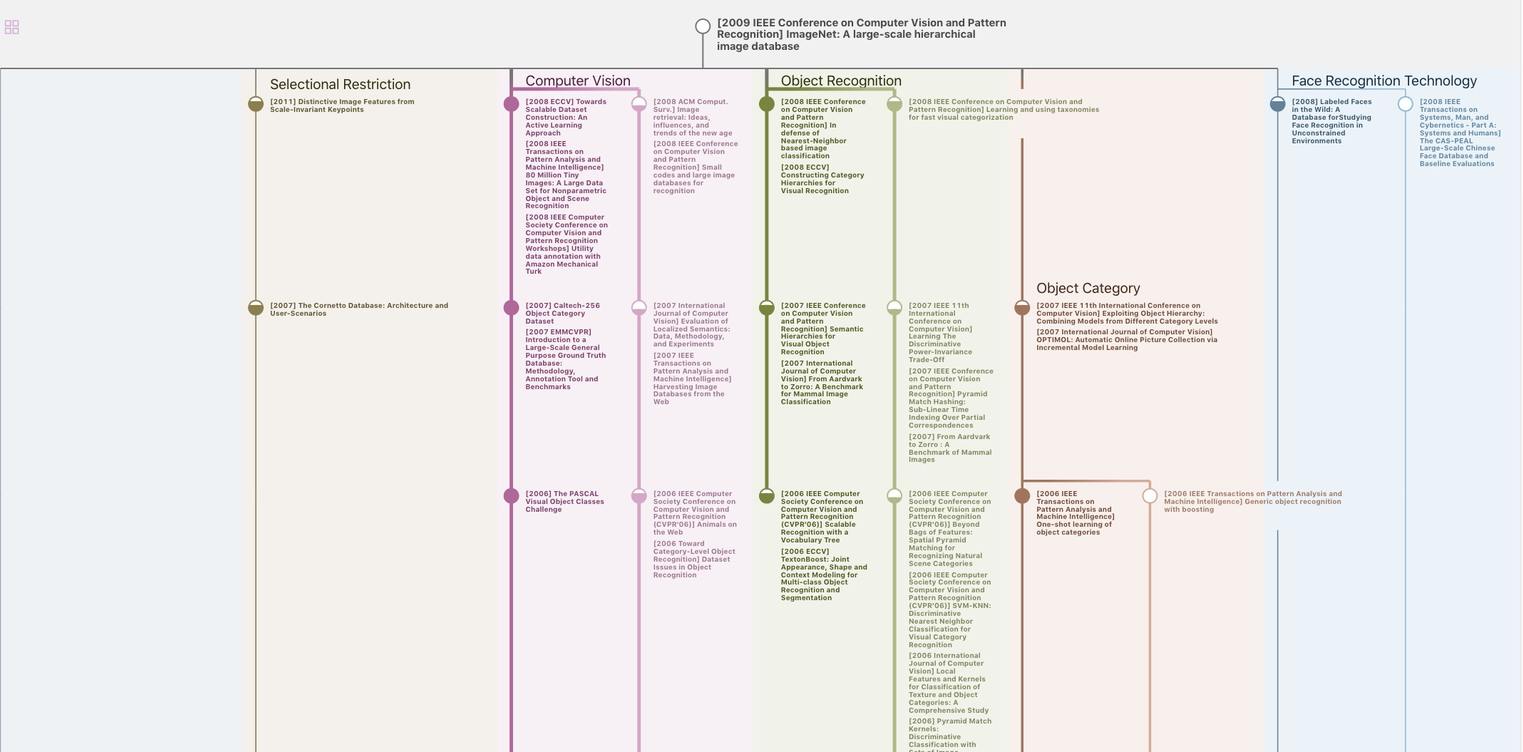
生成溯源树,研究论文发展脉络
Chat Paper
正在生成论文摘要