Prompt Tuning in Biomedical Relation Extraction
Journal of Healthcare Informatics Research(2024)
摘要
Biomedical relation extraction (RE) is critical in constructing high-quality knowledge graphs and databases as well as supporting many downstream text mining applications. This paper explores prompt tuning on biomedical RE and its few-shot scenarios, aiming to propose a simple yet effective model for this specific task. Prompt tuning reformulates natural language processing (NLP) downstream tasks into masked language problems by embedding specific text prompts into the original input, facilitating the adaption of pre-trained language models (PLMs) to better address these tasks. This study presents a customized prompt tuning model designed explicitly for biomedical RE, including its applicability in few-shot learning contexts. The model’s performance was rigorously assessed using the chemical-protein relation (CHEMPROT) dataset from BioCreative VI and the drug-drug interaction (DDI) dataset from SemEval-2013, showcasing its superior performance over conventional fine-tuned PLMs across both datasets, encompassing few-shot scenarios. This observation underscores the effectiveness of prompt tuning in enhancing the capabilities of conventional PLMs, though the extent of enhancement may vary by specific model. Additionally, the model demonstrated a harmonious balance between simplicity and efficiency, matching state-of-the-art performance without needing external knowledge or extra computational resources. The pivotal contribution of our study is the development of a suitably designed prompt tuning model, highlighting prompt tuning’s effectiveness in biomedical RE. It offers a robust, efficient approach to the field’s challenges and represents a significant advancement in extracting complex relations from biomedical texts.
更多查看译文
关键词
Biomedical relation extraction,Prompt tuning,Pre-trained language models,Few-shot learning
AI 理解论文
溯源树
样例
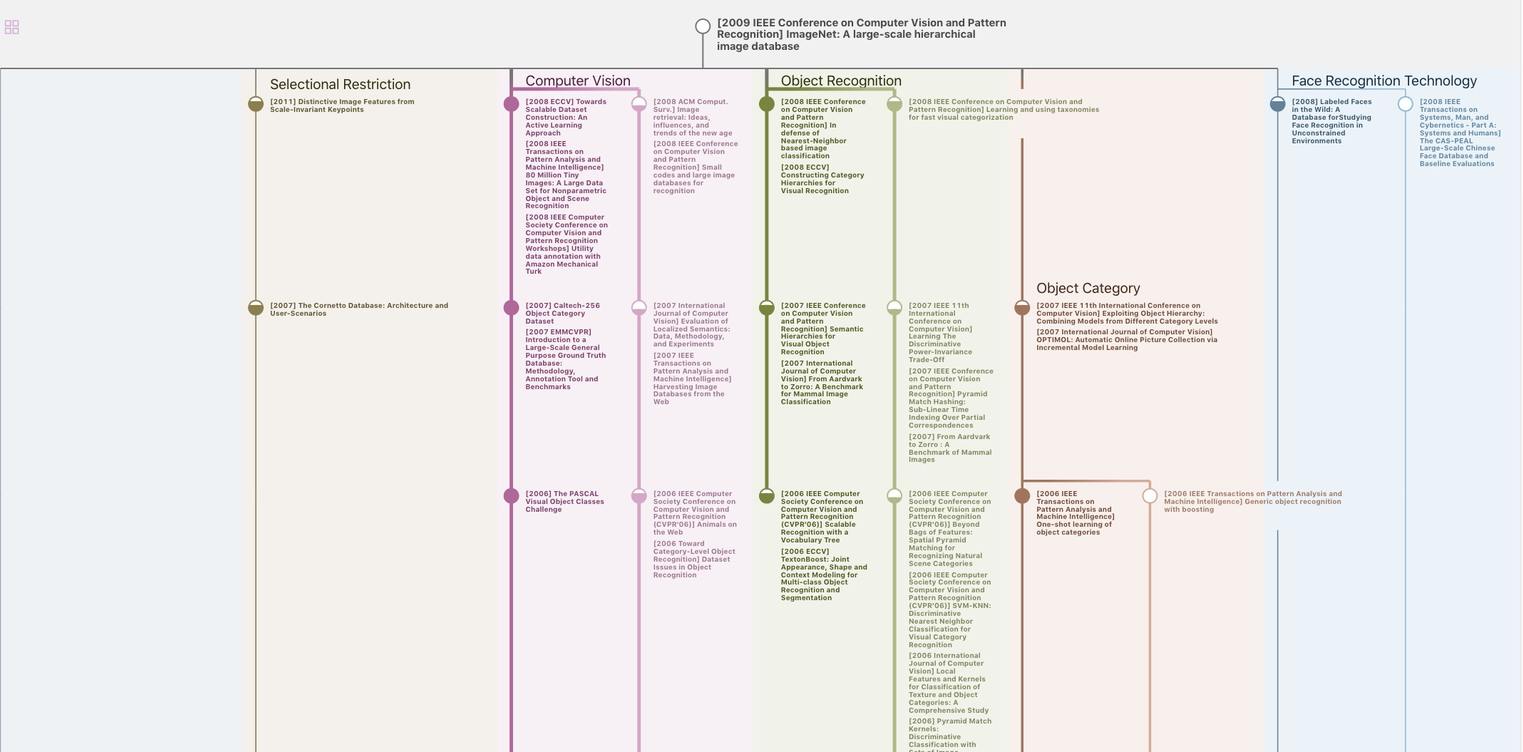
生成溯源树,研究论文发展脉络
Chat Paper
正在生成论文摘要