Abstract: 3D Medical Image Segmentation with Transformer-based Scaling of ConvNets - MedNeXt.
BILDVERARBEITUNG FUR DIE MEDIZIN 2024(2024)
摘要
Transformer-based architectures have seen widespread adoption recently for medical image segmentation. However, achieving performances equivalent to those in natural images are challenging due to the absence of large-scale annotated datasets. In contrast, convolutional networks have higher inductive biases and consequently, are easier to train to high performance. Recently, the ConvNeXt architecture attempted to improve the standard ConvNet by upgrading the popular ResNet blocks to mirror Transformer blocks. In this work, we extend upon this to design a modernized and scalable convolutional architecture customized to challenges of dense segmentation tasks in data-scarce medical settings. In this work, we introduce the MedNeXt architecture which is a Transformer-inspired, scalable large-kernel network for medical image segmentation with 4 key features - 1) Fully ConvNeXt 3D Encoder-Decoder architecture to leverage network-wide benefits of the block design, 2) Residual ConvNeXt blocks for up and downsampling to preserve semantic richness across scales, 3) Upkern, an algorithm to iteratively increase kernel size by upsampling small kernel networks, thus preventing performance saturation on limited data, 4) Compound scaling of depth, width and kernel size to leverage the benefits of large-scale variants of the MedNeXt architecture. With state-of-the-art performance on 4 popular segmentation tasks, across variations in imaging modalities (CT, MRI) and dataset sizes, MedNeXt represents a modernized deep architecture for medical image segmentation. This work was originally published in [1]. Our code is made publicly available at: https://github.com/MIC-DKFZ/MedNeXt.
更多查看译文
AI 理解论文
溯源树
样例
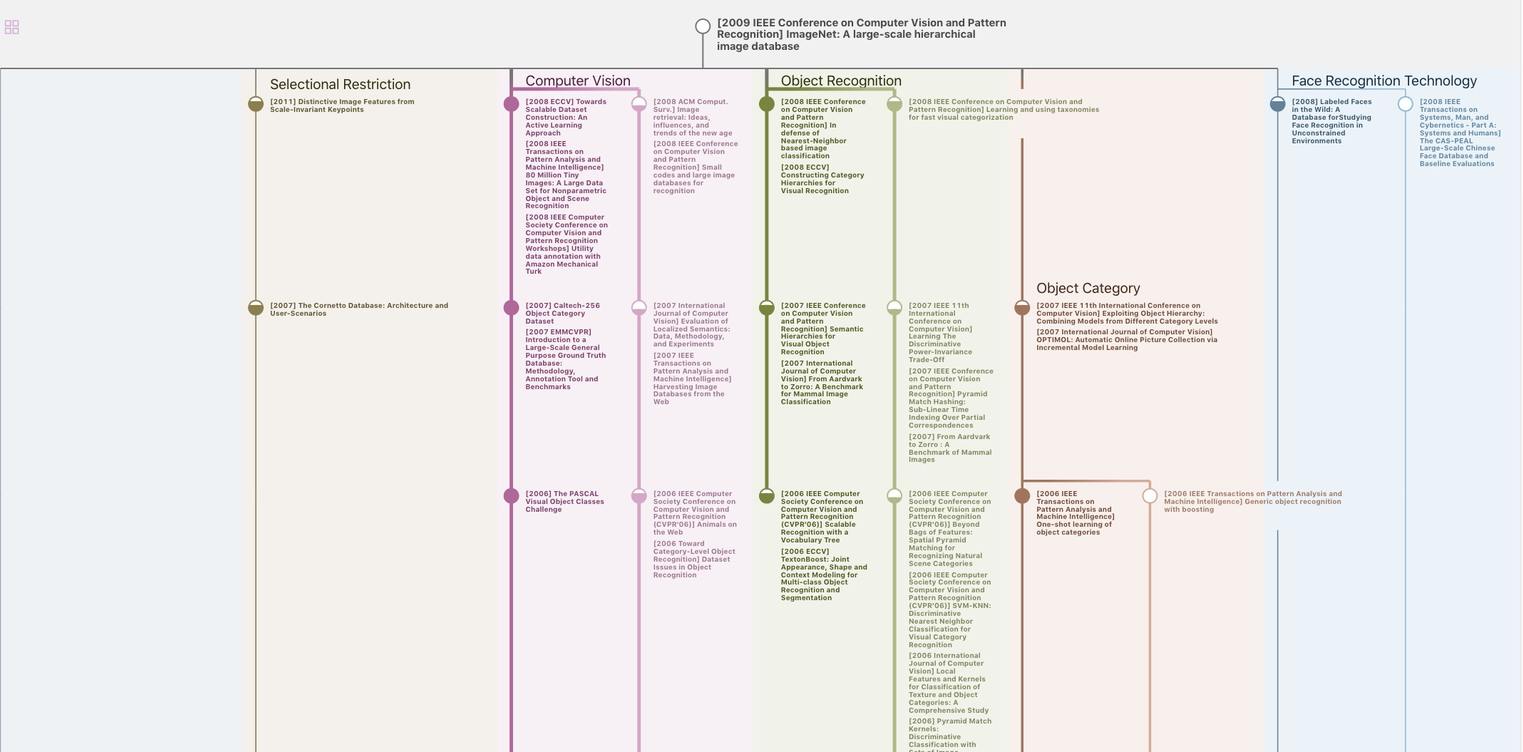
生成溯源树,研究论文发展脉络
Chat Paper
正在生成论文摘要