Rethinking The Uniformity Metric in Self-Supervised Learning
ICLR 2024(2024)
摘要
Uniformity plays a crucial role in the assessment of learned representations,
contributing to a deeper comprehension of self-supervised learning. The seminal
work by introduced a uniformity metric that
quantitatively measures the collapse degree of learned representations.
Directly optimizing this metric together with alignment proves to be effective
in preventing constant collapse. However, we present both theoretical and
empirical evidence revealing that this metric lacks sensitivity to dimensional
collapse, highlighting its limitations. To address this limitation and design a
more effective uniformity metric, this paper identifies five fundamental
properties, some of which the existing uniformity metric fails to meet. We
subsequently introduce a novel uniformity metric that satisfies all of these
desiderata and exhibits sensitivity to dimensional collapse. When applied as an
auxiliary loss in various established self-supervised methods, our proposed
uniformity metric consistently enhances their performance in downstream
tasks.Our code was released at
https://github.com/sunset-clouds/WassersteinUniformityMetric.
更多查看译文
关键词
Desiderata of Ideal Uniformity Metric,Dimensional Collapse,Wasserstein Distance,Self-Supervised Learning
AI 理解论文
溯源树
样例
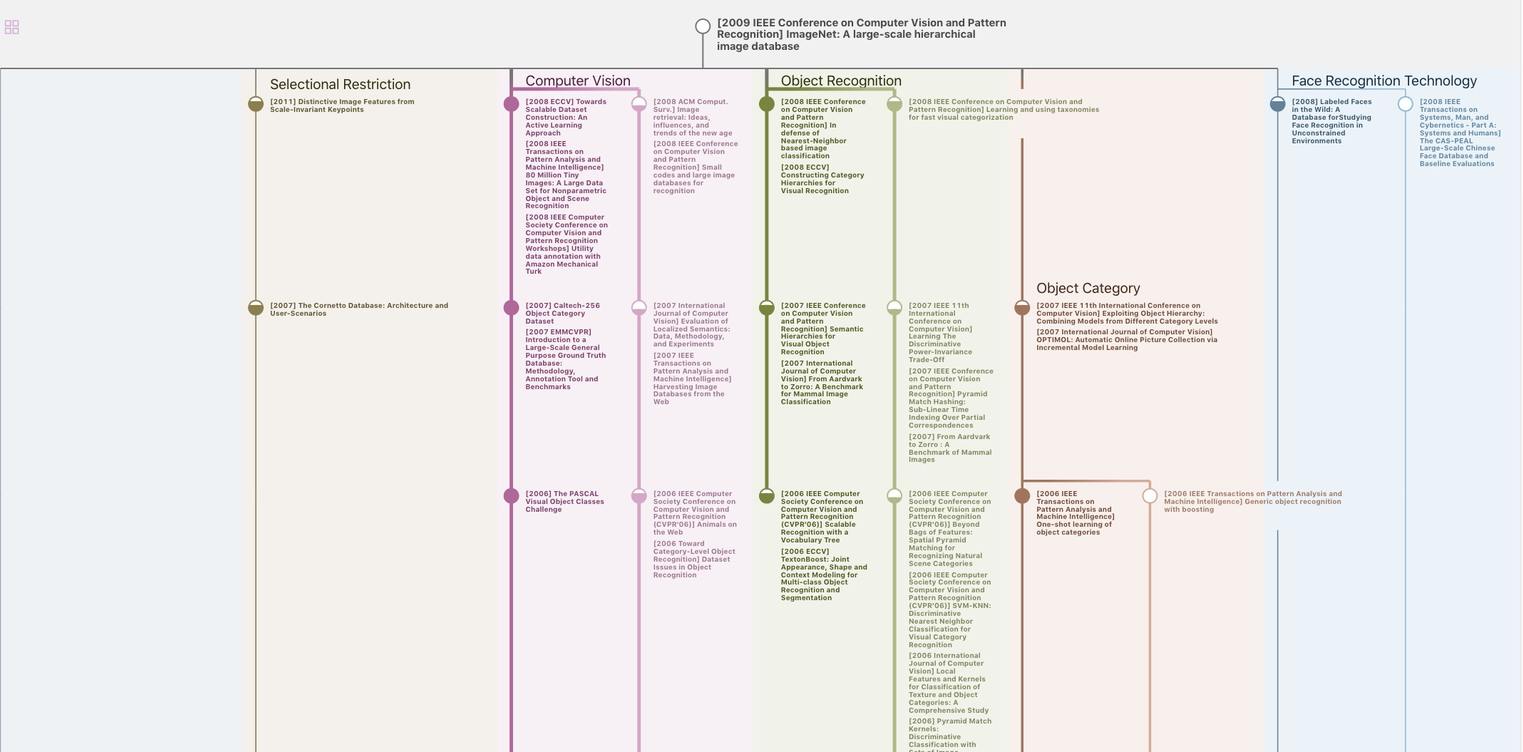
生成溯源树,研究论文发展脉络
Chat Paper
正在生成论文摘要