Robust sparse graph regularized nonnegative matrix factorization for automatic depression diagnosis
BIOMEDICAL SIGNAL PROCESSING AND CONTROL(2024)
摘要
Multichannel electroencephalogram (EEG) signals, which directly reflects the brain's inner workings state, is an powerful tool to diagnosis depression. For EEG -based depression diagnosis, appropriate features are important to get a good classification result. Considering the multichannel nature of the EEG signals, the extracted features are generally high -dimensional and contain many redundant features, which impairs the performance of the classifier. Therefore, it is necessary to perform dimensionality reduction. However, existing dimensionality reduction methods are not well suited for EEG data analysis. For this reason, a novel dimensionality reduction algorithm termed correntropy based sparse graph regularized nonnegative matrix factorization (RSGNMF) is proposed. Our RSGNMF solution finds the discriminative low -dimensional representations via joint optimization for better classification. Specifically, RSGNMF adopts correntropy replace the squared Euclidean distance (SED) into nonnegative matrix factorization (NMF) as similarity measure to increase the robustness for noise and outliers, and simultaneously integrating graph regularization and sparse constraints. In order to solve the optimization problem of RSGNMF, the half -quadratic technique was used and subsequently the multiplicative update rule was obtained. Furthermore, convergence and computational complexity of RSGNMF is analyzed. Experimental results reveal the effectiveness of RSGNMF in EEG depression diagnosis in comparison with other state-of-the-art NMF methods. It also shows the practical application value of our method in detecting depression.
更多查看译文
关键词
Depression diagnosis,Dimensionality reduction,EEG,Nonnegative matrix factorization
AI 理解论文
溯源树
样例
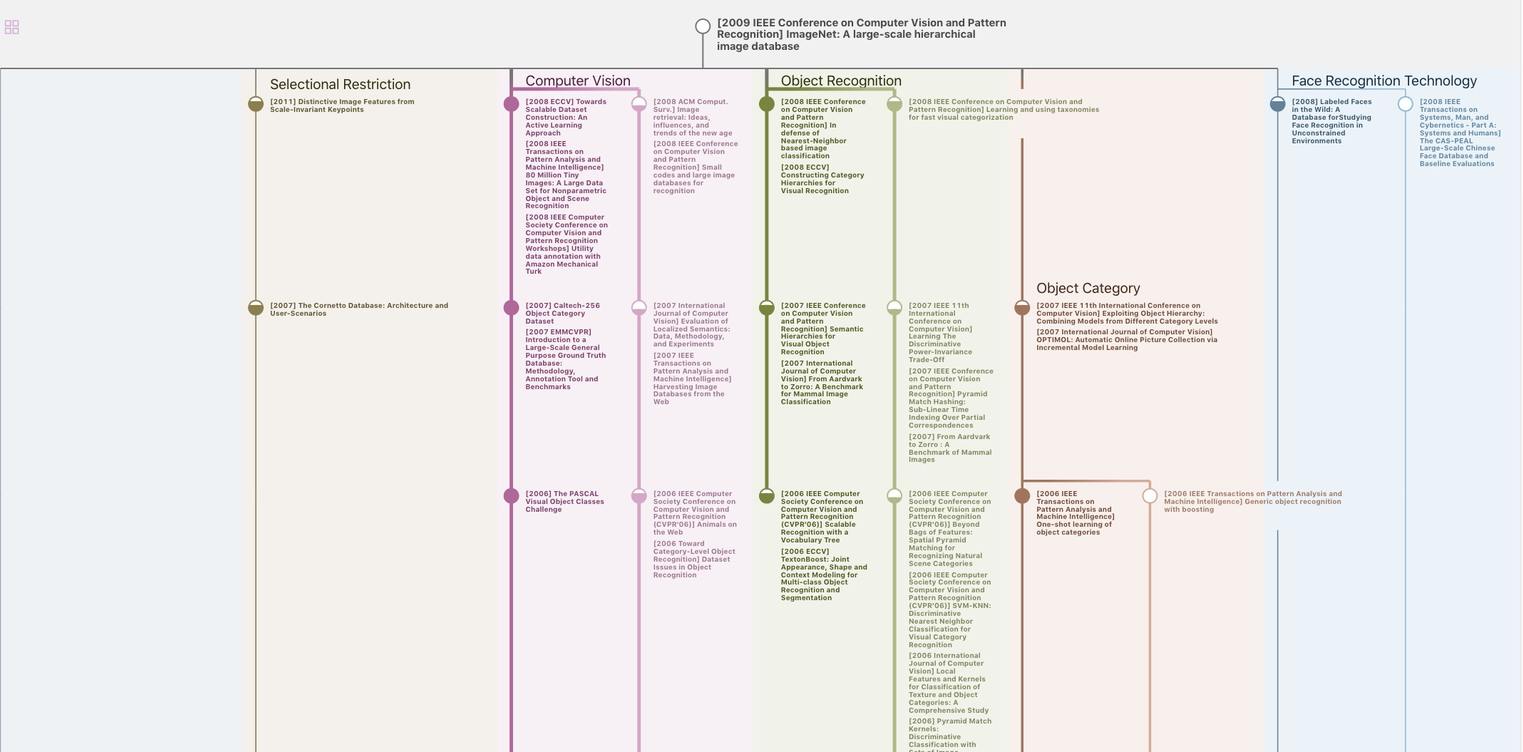
生成溯源树,研究论文发展脉络
Chat Paper
正在生成论文摘要