SCott: Accelerating Diffusion Models with Stochastic Consistency Distillation
arXiv (Cornell University)(2024)
摘要
The iterative sampling procedure employed by diffusion models (DMs) oftenleads to significant inference latency. To address this, we propose StochasticConsistency Distillation (SCott) to enable accelerated text-to-imagegeneration, where high-quality generations can be achieved with just 1-2sampling steps, and further improvements can be obtained by adding additionalsteps. In contrast to vanilla consistency distillation (CD) which distills theordinary differential equation solvers-based sampling process of a pretrainedteacher model into a student, SCott explores the possibility and validates theefficacy of integrating stochastic differential equation (SDE) solvers into CDto fully unleash the potential of the teacher. SCott is augmented withelaborate strategies to control the noise strength and sampling process of theSDE solver. An adversarial loss is further incorporated to strengthen thesample quality with rare sampling steps. Empirically, on the MSCOCO-2017 5Kdataset with a Stable Diffusion-V1.5 teacher, SCott achieves an FID (FrechetInceptio Distance) of 22.1, surpassing that (23.4) of the 1-step InstaFlow (Liuet al., 2023) and matching that of 4-step UFOGen (Xue et al., 2023b). Moreover,SCott can yield more diverse samples than other consistency models forhigh-resolution image generation (Luo et al., 2023a), with up to 16improvement in a qualified metric. The code and checkpoints are coming soon.
更多查看译文
关键词
Robust Optimization,Model Selection
AI 理解论文
溯源树
样例
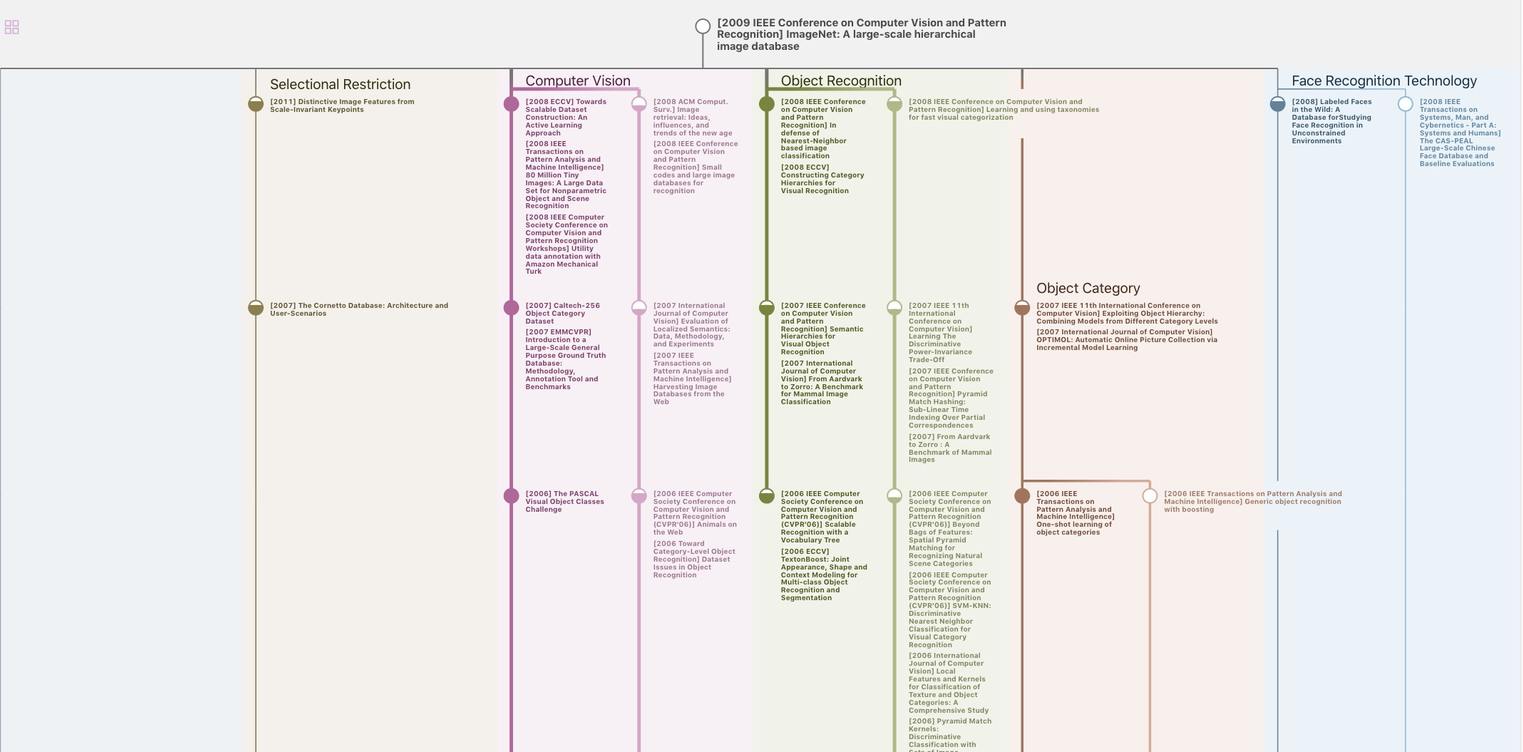
生成溯源树,研究论文发展脉络
Chat Paper
正在生成论文摘要