Dynamic Sparse Learning: A Novel Paradigm for Efficient Recommendation
PROCEEDINGS OF THE 17TH ACM INTERNATIONAL CONFERENCE ON WEB SEARCH AND DATA MINING, WSDM 2024(2024)
摘要
In the realm of deep learning-based recommendation systems, the increasing computational demands, driven by the growing number of users and items, pose a significant challenge to practical deployment. This challenge is primarily twofold: reducing the model size while effectively learning user and item representations for efficient recommendations. Despite considerable advancements in model compression and architecture search, prevalent approaches face notable constraints. These include substantial additional computational costs from pre-training/re-training in model compression and an extensive search space in architecture design. Additionally, managing complexity and adhering to memory constraints is problematic, especially in scenarios with strict time or space limitations. Addressing these issues, this paper introduces a novel learning paradigm, Dynamic Sparse Learning (DSL), tailored for recommendation models. DSL innovatively trains a lightweight sparse model from scratch, periodically evaluating and dynamically adjusting each weight's significance and the model's sparsity distribution during the training. This approach ensures a consistent and minimal parameter budget throughout the full learning lifecycle, paving the way for "end-to-end" efficiency from training to inference. Our extensive experimental results underline DSL's effectiveness, significantly reducing training and inference costs while delivering comparable recommendation performance. We give an code link of our work: https://github.com/shuyao-wang/DSL.
更多查看译文
关键词
Efficient Recommendation,Sparse Learning
AI 理解论文
溯源树
样例
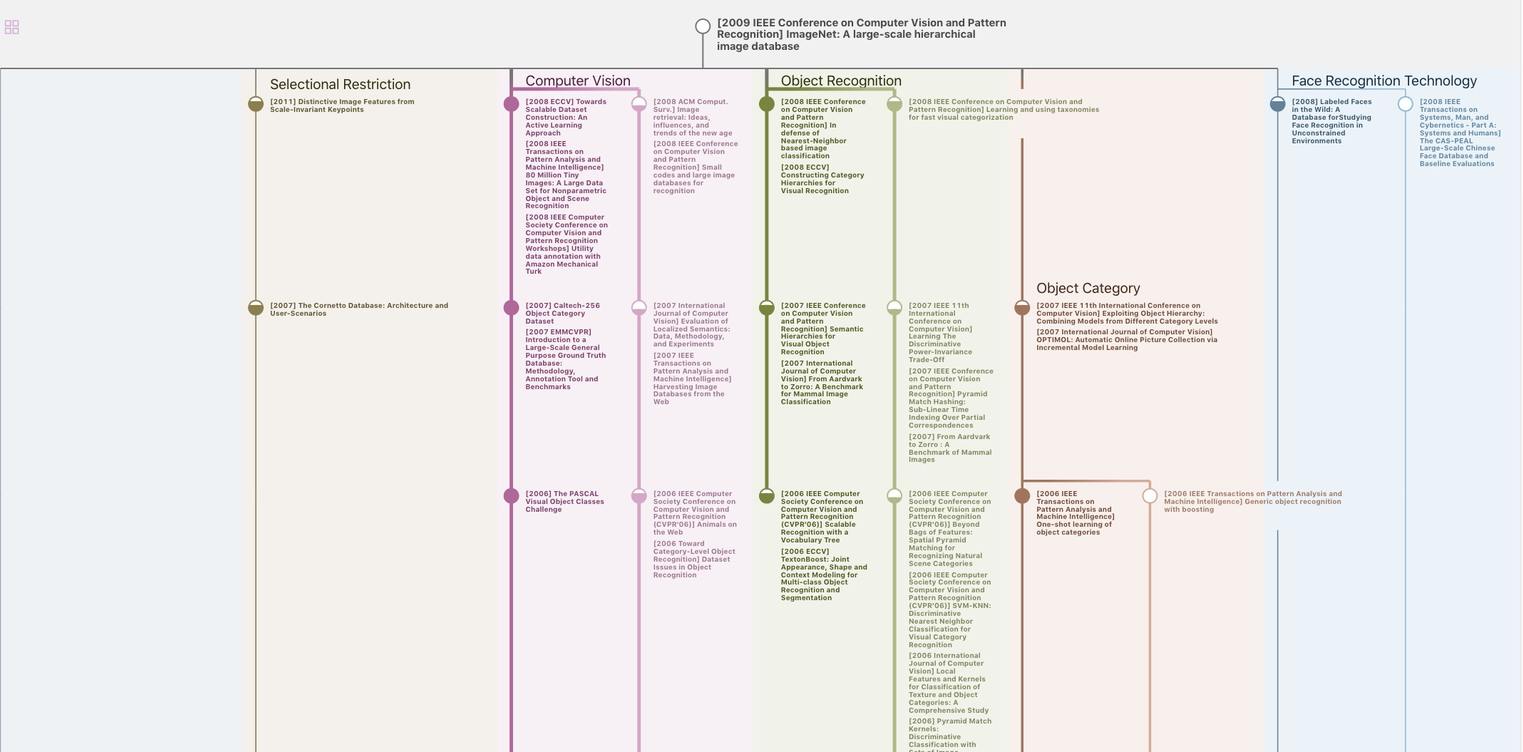
生成溯源树,研究论文发展脉络
Chat Paper
正在生成论文摘要