Improved Algorithm for Adversarial Linear Mixture MDPs with Bandit Feedback and Unknown Transition
arXiv (Cornell University)(2024)
摘要
We study reinforcement learning with linear function approximation, unknowntransition, and adversarial losses in the bandit feedback setting.Specifically, we focus on linear mixture MDPs whose transition kernel is alinear mixture model. We propose a new algorithm that attains anO(d√(HS^3K) + √(HSAK)) regret with high probability,where d is the dimension of feature mappings, S is the size of state space,A is the size of action space, H is the episode length and K is thenumber of episodes. Our result strictly improves the previous best-knownO(dS^2 √(K) + √(HSAK)) result in Zhao et al. (2023a)since H ≤ S holds by the layered MDP structure. Our advancements areprimarily attributed to (i) a new least square estimator for the transitionparameter that leverages the visit information of all states, as opposed toonly one state in prior work, and (ii) a new self-normalized concentrationtailored specifically to handle non-independent noises, originally proposed inthe dynamic assortment area and firstly applied in reinforcement learning tohandle correlations between different states.
更多查看译文
关键词
Support Vector Machines
AI 理解论文
溯源树
样例
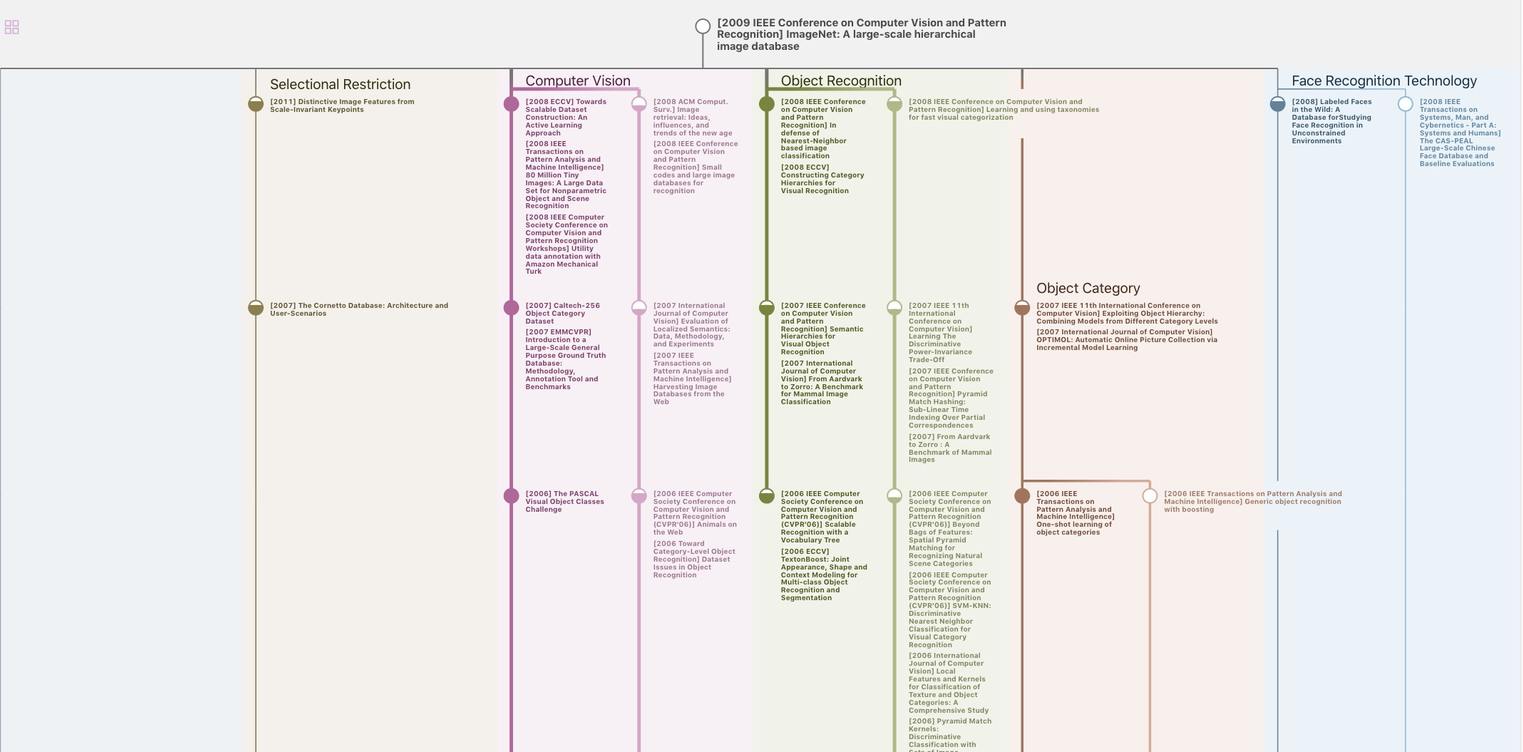
生成溯源树,研究论文发展脉络
Chat Paper
正在生成论文摘要