Intelligent Switching for Reset-Free RL
ICLR 2024(2024)
摘要
In the real world, the strong episode resetting mechanisms that are needed to train
agents in simulation are unavailable. The resetting assumption limits the potential
of reinforcement learning in the real world, as providing resets to an agent usually
requires the creation of additional handcrafted mechanisms or human interventions.
Recent work aims to train agents (forward) with learned resets by constructing
a second (backward) agent that returns the forward agent to the initial state. We
find that the termination and timing of the transitions between these two agents
are crucial for algorithm success. With this in mind, we create a new algorithm,
Reset Free RL with Intelligently Switching Controller (RISC) which intelligently
switches between the two agents based on the agent’s confidence in achieving its
current goal. Our new method achieves state-of-the-art performance on several
challenging environments for reset-free RL.
更多查看译文
关键词
Reset-Free RL
AI 理解论文
溯源树
样例
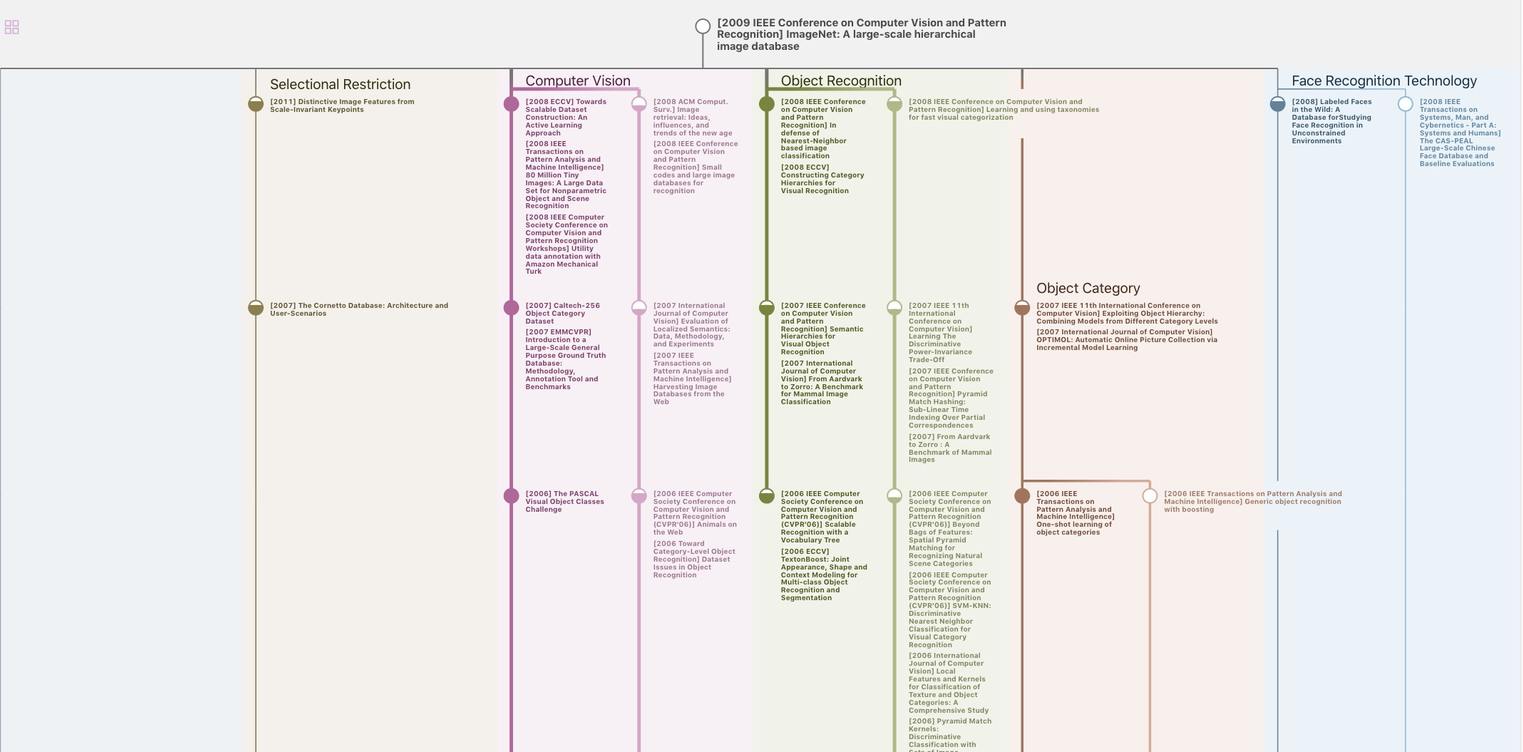
生成溯源树,研究论文发展脉络
Chat Paper
正在生成论文摘要