Be Aware of the Neighborhood Effect: Modeling Selection Bias under Interference for Recommendation
ICLR 2024(2024)
摘要
The interaction between users and recommender systems is not only affected by selection bias but also the neighborhood effect, i.e., the interaction between a user and an item is affected by the interactions between other users and other items, or between the same user and other items, or between other users and the same item. Many previous studies have focused on addressing selection bias to achieve unbiased learning of the prediction model, but the lack of consideration of neighborhood effects can lead to biased estimates and suboptimal performance of the prediction model. In this paper, we formally formulate the neighborhood effect as an interference problem from the perspective of causal inference and introduce a treatment representation to capture the neighborhood effect. On this basis, we propose a novel ideal loss that can be used to deal with selection bias in the presence of neighborhood effects. In addition, we further develop two novel estimators for the ideal loss. We theoretically establish the connection between the proposed methods and previous methods ignoring the neighborhood effect and show that the proposed methods achieve unbiased learning when both selection bias and neighborhood effects are present, while the existing methods are biased. Extensive semi-synthetic and real-world experiments are conducted to demonstrate the effectiveness of the proposed methods.
更多查看译文
关键词
Recommender system,Selection Bias,Neighborhood effect
AI 理解论文
溯源树
样例
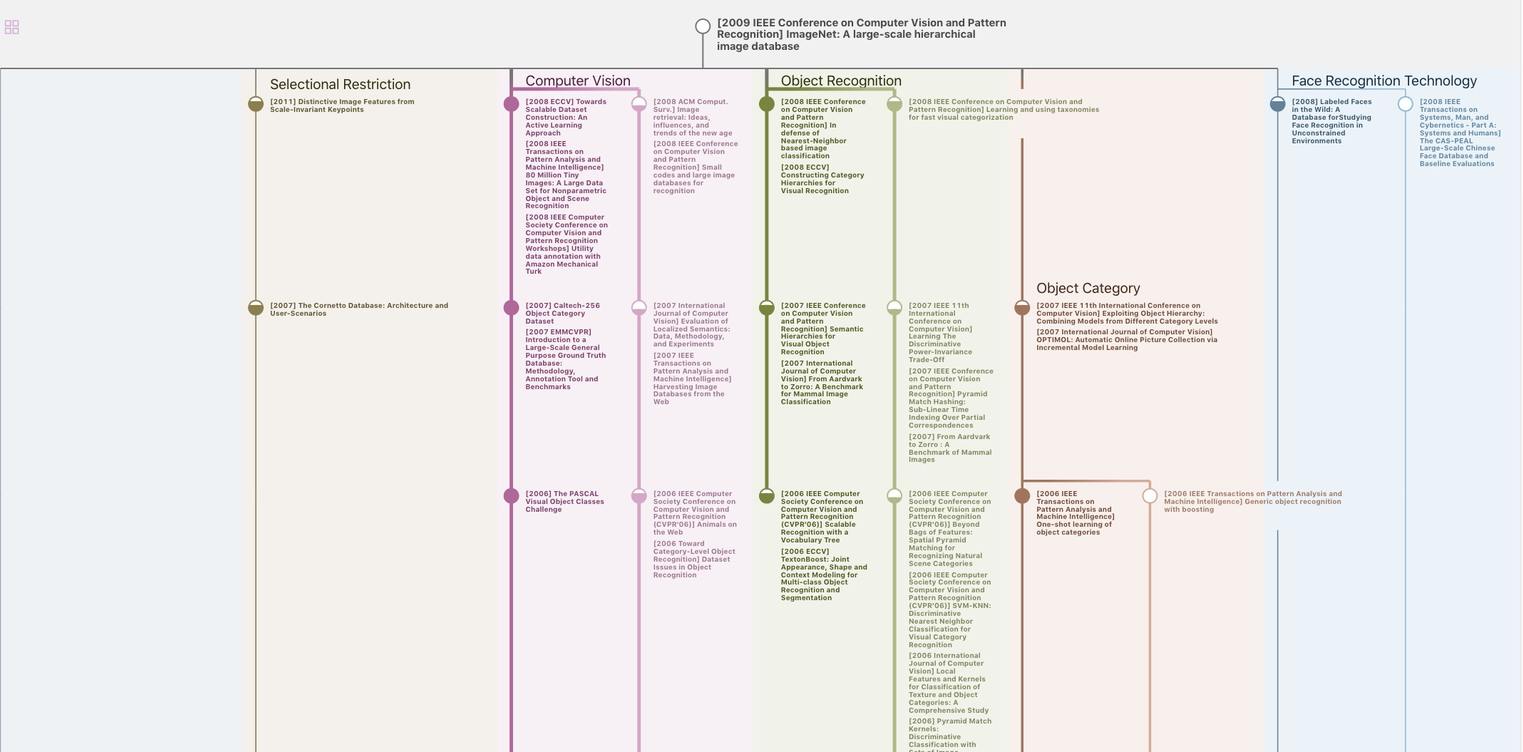
生成溯源树,研究论文发展脉络
Chat Paper
正在生成论文摘要