Toward Generalist Anomaly Detection Via In-context Residual Learning with Few-shot Sample Prompts
2024 IEEE/CVF Conference on Computer Vision and Pattern Recognition (CVPR)(2024)
摘要
This paper explores the problem of Generalist Anomaly Detection (GAD), aimingto train one single detection model that can generalize to detect anomalies indiverse datasets from different application domains without any furthertraining on the target data. Some recent studies have shown that largepre-trained Visual-Language Models (VLMs) like CLIP have strong generalizationcapabilities on detecting industrial defects from various datasets, but theirmethods rely heavily on handcrafted text prompts about defects, making themdifficult to generalize to anomalies in other applications, e.g., medical imageanomalies or semantic anomalies in natural images. In this work, we propose totrain a GAD model with few-shot normal images as sample prompts for AD ondiverse datasets on the fly. To this end, we introduce a novel approach thatlearns an in-context residual learning model for GAD, termed InCTRL. It istrained on an auxiliary dataset to discriminate anomalies from normal samplesbased on a holistic evaluation of the residuals between query images andfew-shot normal sample prompts. Regardless of the datasets, per definition ofanomaly, larger residuals are expected for anomalies than normal samples,thereby enabling InCTRL to generalize across different domains without furthertraining.
更多查看译文
关键词
Anomaly Detection,Vision Language Model,Generalist Anomaly Detection,Few-shot Anomaly Detection
AI 理解论文
溯源树
样例
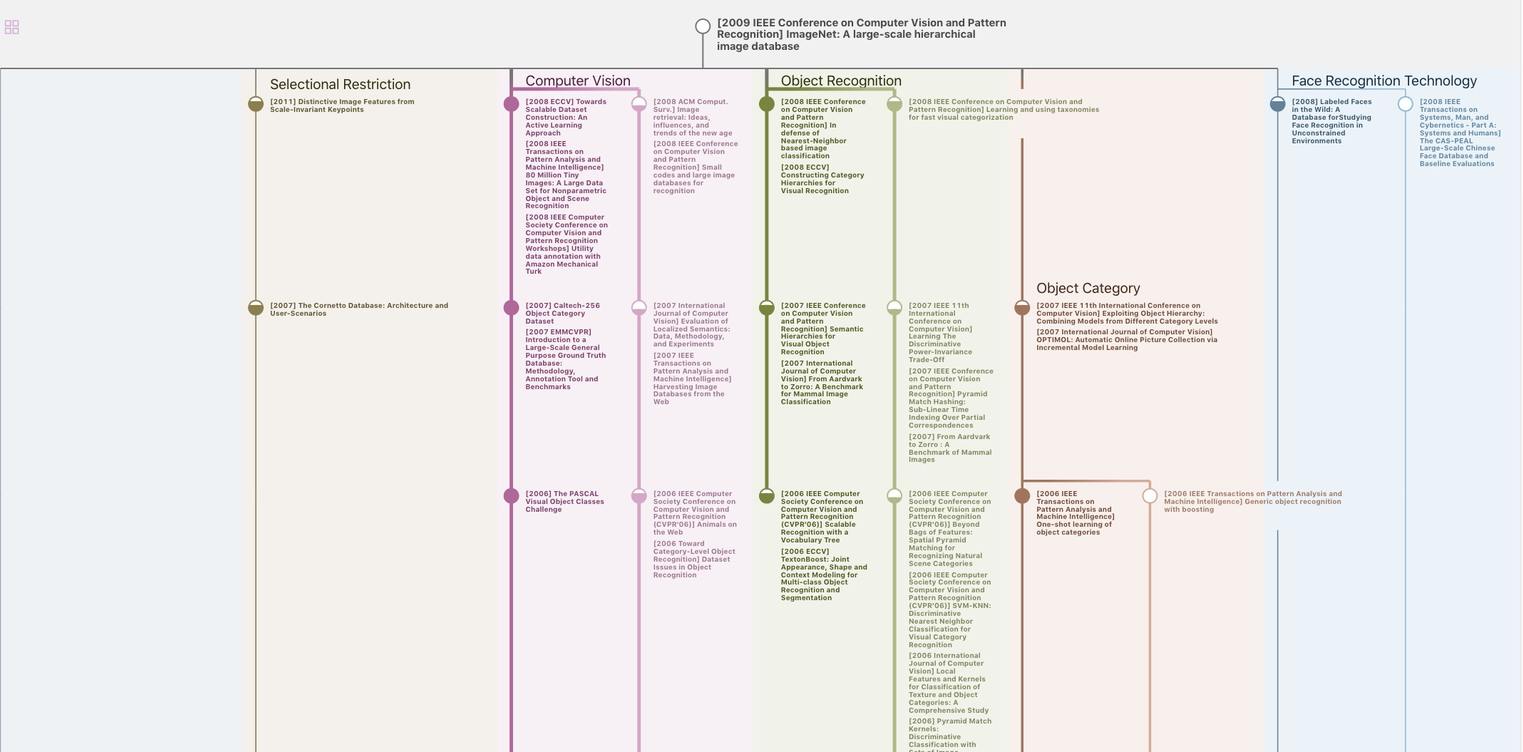
生成溯源树,研究论文发展脉络
Chat Paper
正在生成论文摘要