Bitrate Adaptation and Guidance with Meta Reinforcement Learning
IEEE Transactions on Mobile Computing(2024)
摘要
Adaptive bitrate (ABR) schemes enable streaming clients to adapt to time-varying network/device conditions for a stall-free viewing experience. Most ABR schemes use manually tuned heuristics or learning-based methods. Heuristics are easy to implement but do not always perform well, whereas learning-based methods generally perform well but are difficult to deploy on low-resource devices. To make the most out of both worlds, we earlier developed
Ahaggar
, a learning-based scheme executing on the server side that provides quality-aware bitrate guidance to streaming clients running their own heuristics.
Ahaggar
's novelty is the meta reinforcement learning approach taking network conditions, clients' statuses and device resolutions, and streamed content as input features to perform bitrate guidance.
Ahaggar
uses the new Common Media Client/Server Data (CMCD/SD) protocols to exchange the necessary metadata between the servers and clients. While
Ahaggar
was a significant step forward, in this study, we focus on three open areas, namely, (
$i$
) exploring the performance of
Ahaggar
in a heterogeneous environment including both
Ahaggar
and non-
Ahaggar
clients with varied network conditions and device resolutions, and (
$ii$
) quantifying the impact of device resolutions on QoE with
Ahaggar
. We thoroughly investigate these areas and report our findings. We also (
$iii$
) discuss the
Ahaggar
design choices. Experiments on an open-source system show that
Ahaggar
adapts to unseen conditions fast and outperforms its competitors in several viewer experience metrics.
更多查看译文
关键词
Adaptive streaming,meta-RL,ABR,CMCD,CMSD,bitrate guidance,quality awareness
AI 理解论文
溯源树
样例
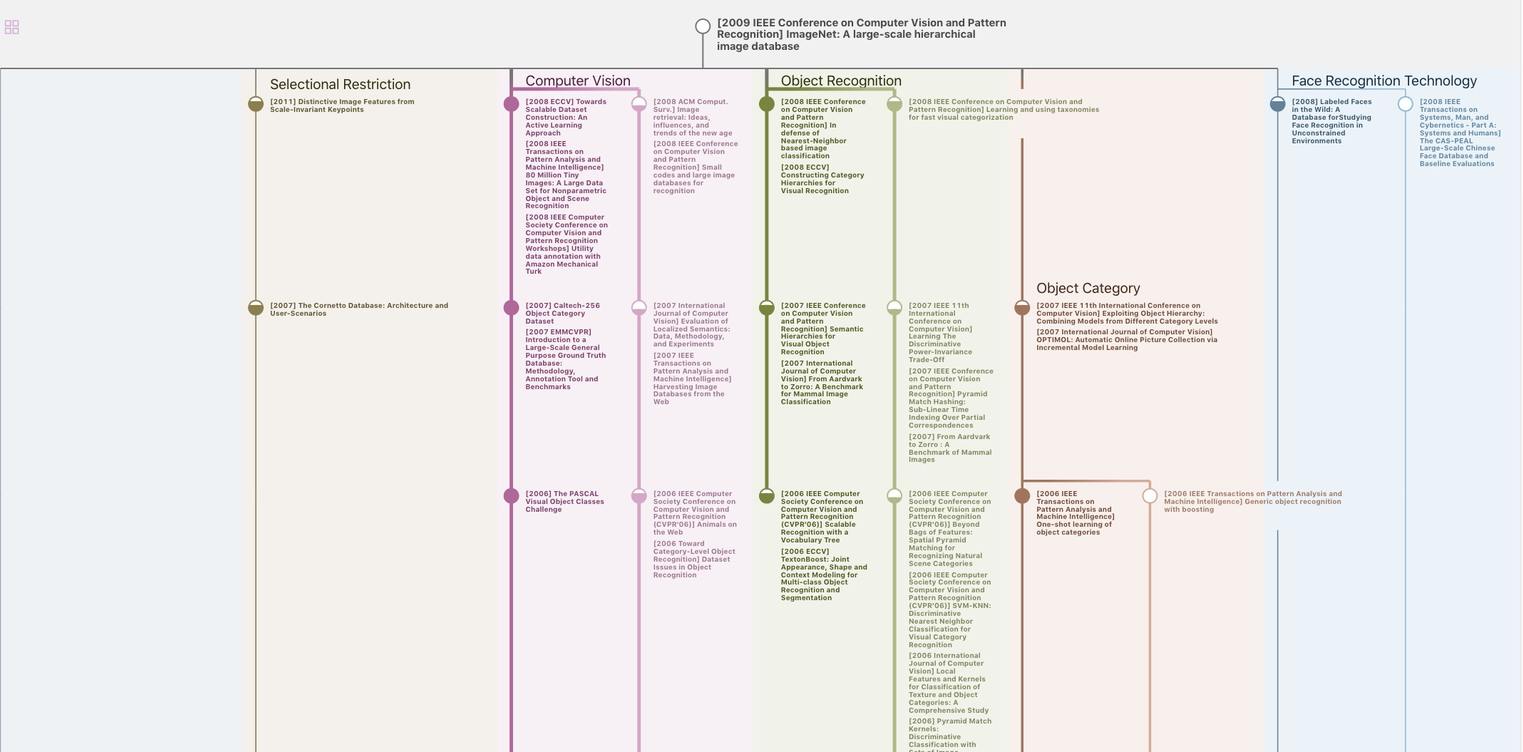
生成溯源树,研究论文发展脉络
Chat Paper
正在生成论文摘要