Gradient Based Feature Attribution in Explainable AI: A Technical Review
arXiv (Cornell University)(2024)
摘要
The surge in black-box AI models has prompted the need to explain theinternal mechanism and justify their reliability, especially in high-stakesapplications, such as healthcare and autonomous driving. Due to the lack of arigorous definition of explainable AI (XAI), a plethora of research related toexplainability, interpretability, and transparency has been developed toexplain and analyze the model from various perspectives. Consequently, with anexhaustive list of papers, it becomes challenging to have a comprehensiveoverview of XAI research from all aspects. Considering the popularity of neuralnetworks in AI research, we narrow our focus to a specific area of XAIresearch: gradient based explanations, which can be directly adopted for neuralnetwork models. In this review, we systematically explore gradient basedexplanation methods to date and introduce a novel taxonomy to categorize theminto four distinct classes. Then, we present the essence of technique detailsin chronological order and underscore the evolution of algorithms. Next, weintroduce both human and quantitative evaluations to measure algorithmperformance. More importantly, we demonstrate the general challenges in XAI andspecific challenges in gradient based explanations. We hope that this surveycan help researchers understand state-of-the-art progress and theircorresponding disadvantages, which could spark their interest in addressingthese issues in future work.
更多查看译文
关键词
XAI Concepts,Machine Learning Interpretability,Interpretable Models,Responsibility in AI,Model Interpretability
AI 理解论文
溯源树
样例
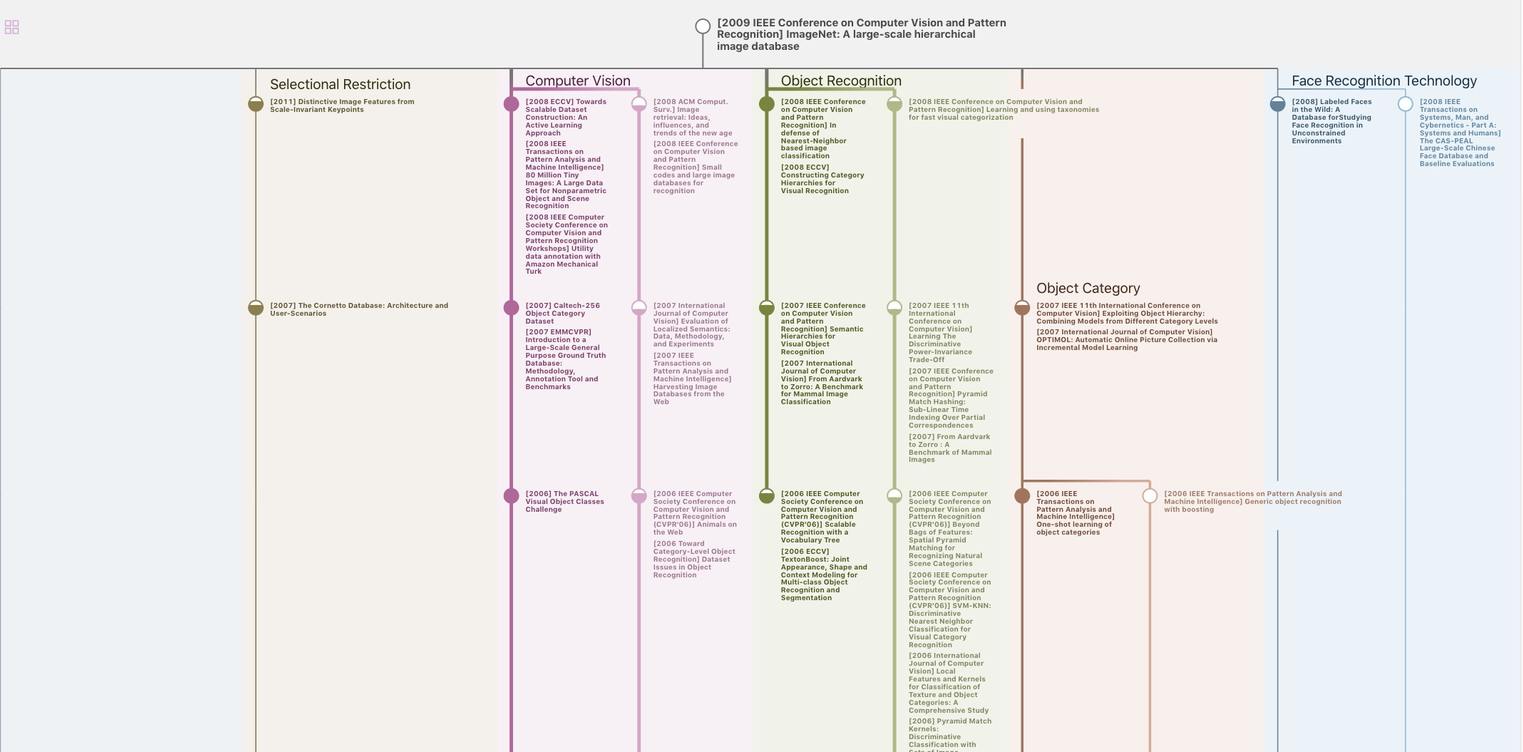
生成溯源树,研究论文发展脉络
Chat Paper
正在生成论文摘要