Gradient Reactivation Enhanced Causal Attention for Out-Of-Distribution Generalizable Graph Classification
ICASSP 2024 - 2024 IEEE International Conference on Acoustics, Speech and Signal Processing (ICASSP)(2024)
摘要
Seeking for generalizable graph representations becomes hot spot in the area of graph learning. Recently, causality theory has been applied for extracting the causal relations between graph data and labels, which are generalizable under distribution shift and result in better OOD generalization. In this paper, for more accurately capturing causal representation of graph data, we propose a gradient reactivation enhanced causal subgraph extraction method. The proposed model utilizes attention mechanism to extract the causal features and attenuates the confounding effect of shortcut features. For ensuring stability of extracted causal features, we propose a novel gradient reactivation method to filter features with greater effect on making prediction. Extensively experimental result proves the effectiveness of the proposed model.
更多查看译文
关键词
Graph classification,out of distribution,causal models,graph neural networks,gradient reactivation
AI 理解论文
溯源树
样例
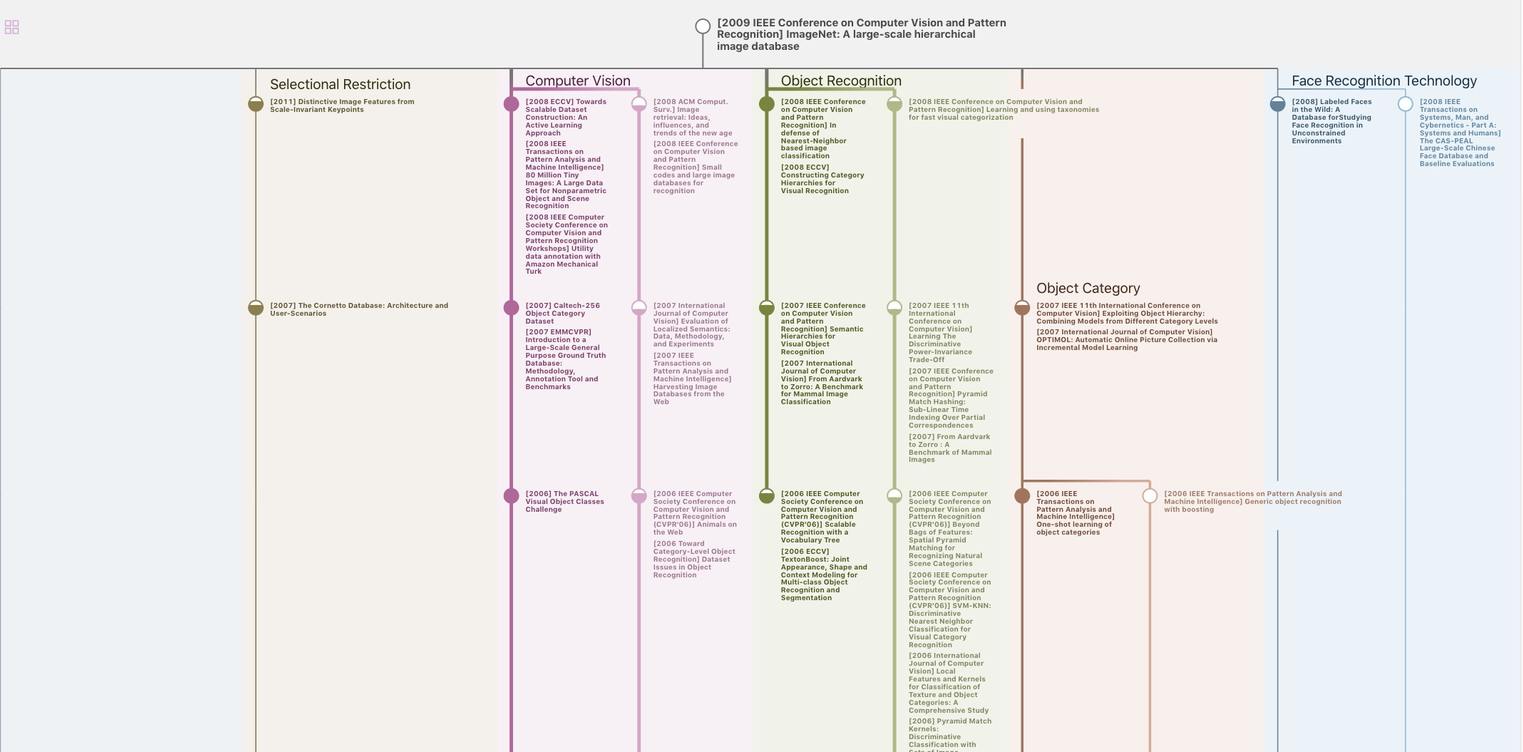
生成溯源树,研究论文发展脉络
Chat Paper
正在生成论文摘要