State space representations of the Roesser type for convolutional layers
CoRR(2024)
摘要
From the perspective of control theory, convolutional layers (of neural
networks) are 2-D (or N-D) linear time-invariant dynamical systems. The usual
representation of convolutional layers by the convolution kernel corresponds to
the representation of a dynamical system by its impulse response. However, many
analysis tools from control theory, e.g., involving linear matrix inequalities,
require a state space representation. For this reason, we explicitly provide a
state space representation of the Roesser type for 2-D convolutional layers
with c_inr_1 + c_outr_2 states, where
c_in/c_out is the number of input/output channels of the
layer and r_1/r_2 characterizes the width/length of the convolution kernel.
This representation is shown to be minimal for c_in =
c_out. We further construct state space representations for dilated,
strided, and N-D convolutions.
更多查看译文
AI 理解论文
溯源树
样例
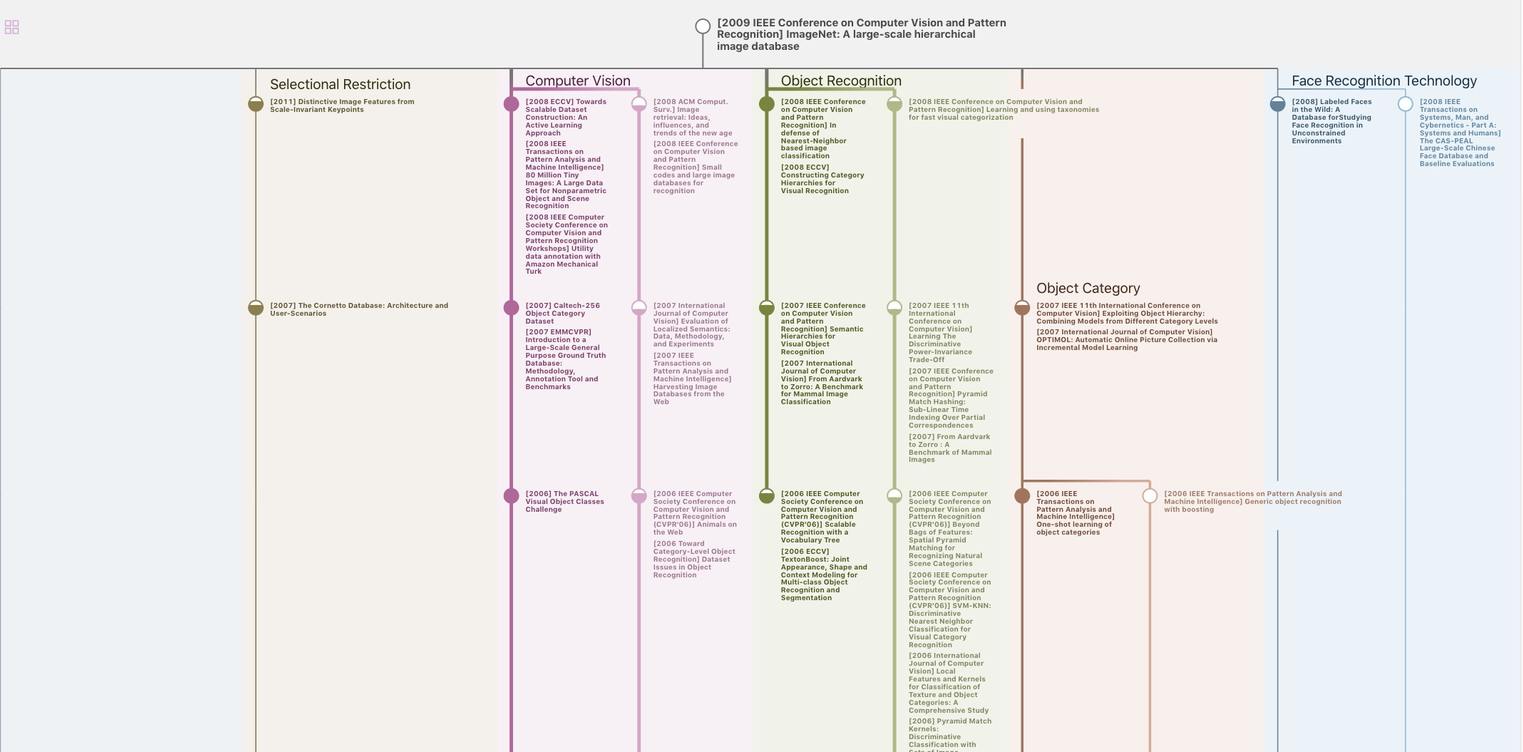
生成溯源树,研究论文发展脉络
Chat Paper
正在生成论文摘要