LLM3:Large Language Model-based Task and Motion Planning with Motion Failure Reasoning
CoRR(2024)
摘要
Conventional Task and Motion Planning (TAMP) approaches rely on manually
crafted interfaces connecting symbolic task planning with continuous motion
generation. These domain-specific and labor-intensive modules are limited in
addressing emerging tasks in real-world settings. Here, we present LLM^3, a
novel Large Language Model (LLM)-based TAMP framework featuring a
domain-independent interface. Specifically, we leverage the powerful reasoning
and planning capabilities of pre-trained LLMs to propose symbolic action
sequences and select continuous action parameters for motion planning.
Crucially, LLM^3 incorporates motion planning feedback through prompting,
allowing the LLM to iteratively refine its proposals by reasoning about motion
failure. Consequently, LLM^3 interfaces between task planning and motion
planning, alleviating the intricate design process of handling domain-specific
messages between them. Through a series of simulations in a box-packing domain,
we quantitatively demonstrate the effectiveness of LLM^3 in solving TAMP
problems and the efficiency in selecting action parameters. Ablation studies
underscore the significant contribution of motion failure reasoning to the
success of LLM^3. Furthermore, we conduct qualitative experiments on a physical
manipulator, demonstrating the practical applicability of our approach in
real-world settings.
更多查看译文
AI 理解论文
溯源树
样例
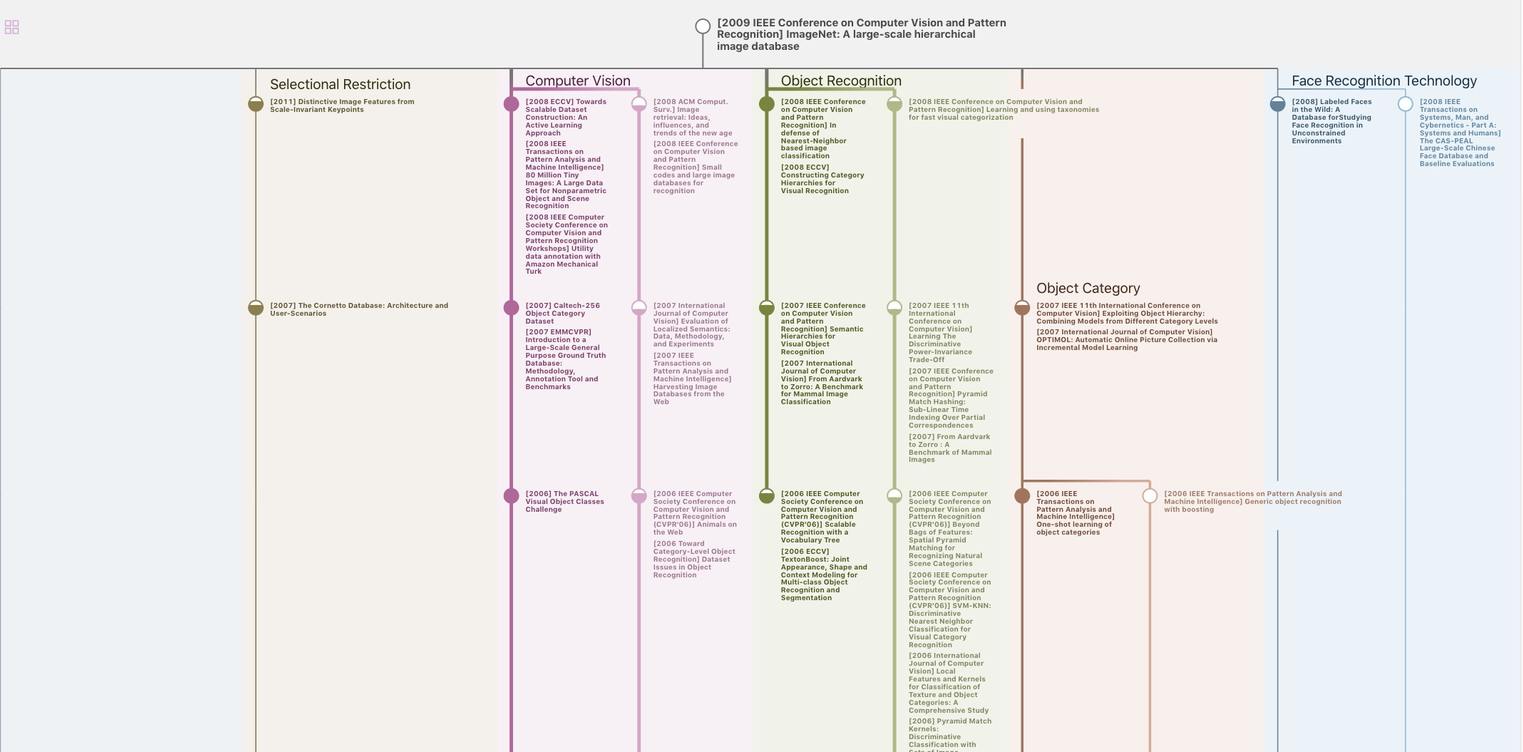
生成溯源树,研究论文发展脉络
Chat Paper
正在生成论文摘要