Graph Networks Stand Strong: Enhancing Robustness via Stability Constraints
ICASSP 2024 - 2024 IEEE International Conference on Acoustics, Speech and Signal Processing (ICASSP)(2024)
摘要
Graph neural networks (GNNs) have achieved great success in graph classification tasks across many domains. However, the varying quality of real-world graph data leads to stability and reliability issues for real-world applications of graph neural networks (GNNs). Improving the robustness of GNNs would help enhance the quality and safety of GNNs in real-world applications. Recently, there have been studies that incorporate insights from information theory, causal theory, etc. into graph classification tasks to improve robustness. However, these strategies rely on extensive task-specific designs that increase model complexity and limit the scope of the methods. In this work, we leverage the interdependence between model stability and robustness by introducing stability constraints to graph neural network models through two different consistency regularization methods. To balance the trade-off between stability constraints and classification performance, we adaptively adjust the strength of the constraints dynamically using multi-objective optimization, making our method applicable to graph classification tasks of varying scales and domains. Extensive experiments on graph datasets from different domains demonstrate the superiority of our proposed method.
更多查看译文
关键词
Graph neural networks,Robustness,Stability
AI 理解论文
溯源树
样例
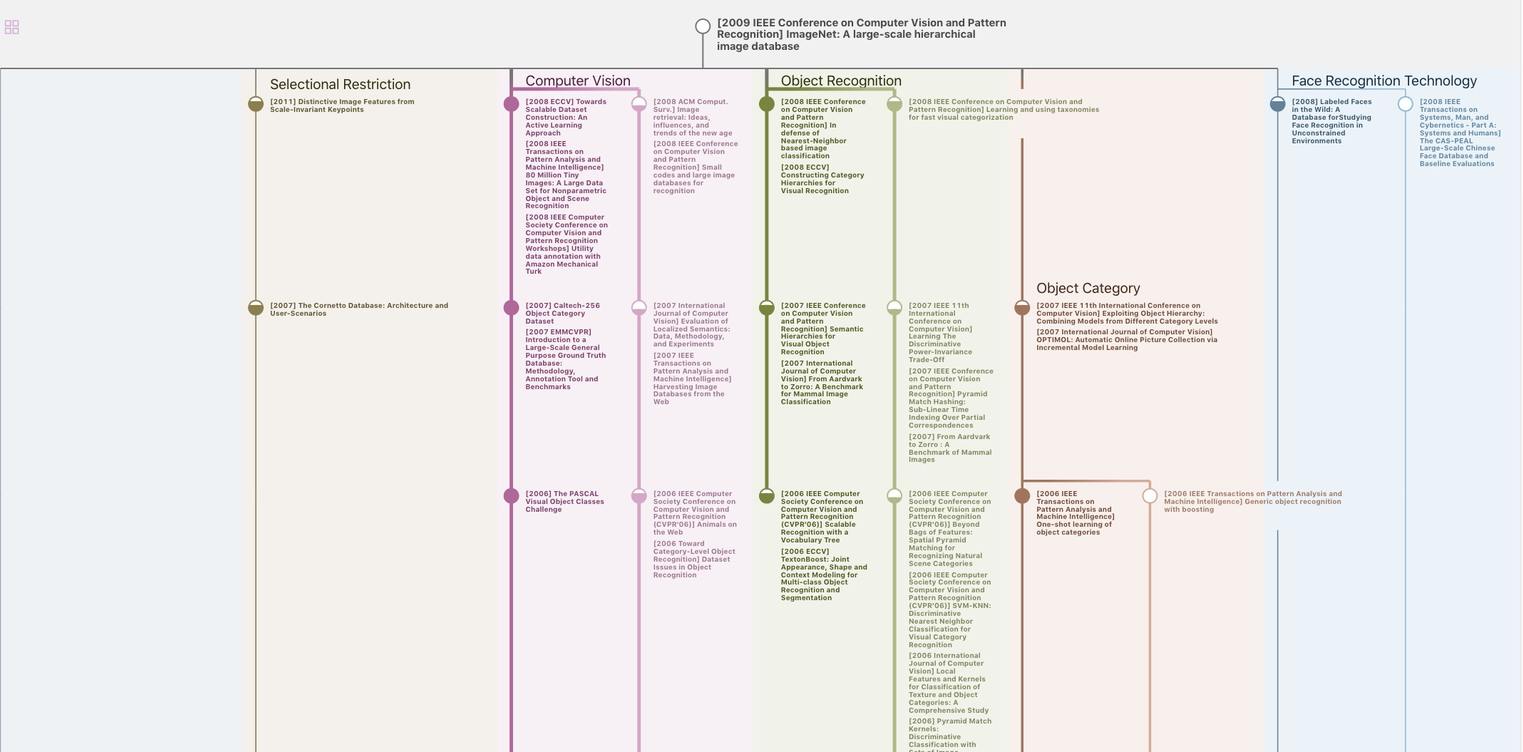
生成溯源树,研究论文发展脉络
Chat Paper
正在生成论文摘要