Learning Hybrid Negative Probability Model for Weakly-Supervised Whole Slide Image Recognition
ICASSP 2024 - 2024 IEEE International Conference on Acoustics, Speech and Signal Processing (ICASSP)(2024)
摘要
Classifying an entire Whole Slide Image (WSI) in a single forward pass is challenging due to its vast resolution. Consequently, current effort on WSI classification resorts to multiple instance learning (MIL), using patch-wise instances to predict categories under image-wise supervision. However, recent MIL methods usually follow implicit instance selection strategy and ignore the effect from inherent patch category imbalances. In a statistical sense, negative patches dominate in WSIs and provide sufficient samples for accurate density estimation. Therefore, in this paper, we learn from anomaly detection and propose a deep MIL framework which learns a hybrid negative probability model to bootstrap discovery of potential positive lesion. We associate attention-based MIL approach with a regularization loss function to explicitly improve patch selection process in positive images. Experiments conducted on benchmarks of WSI recognition demonstrate that our method brings significant improvement to classic attention-based MIL baseline and achieves state-of-the-art performance.
更多查看译文
关键词
multiple instance learning,weakly-supervised learning,whole slide image
AI 理解论文
溯源树
样例
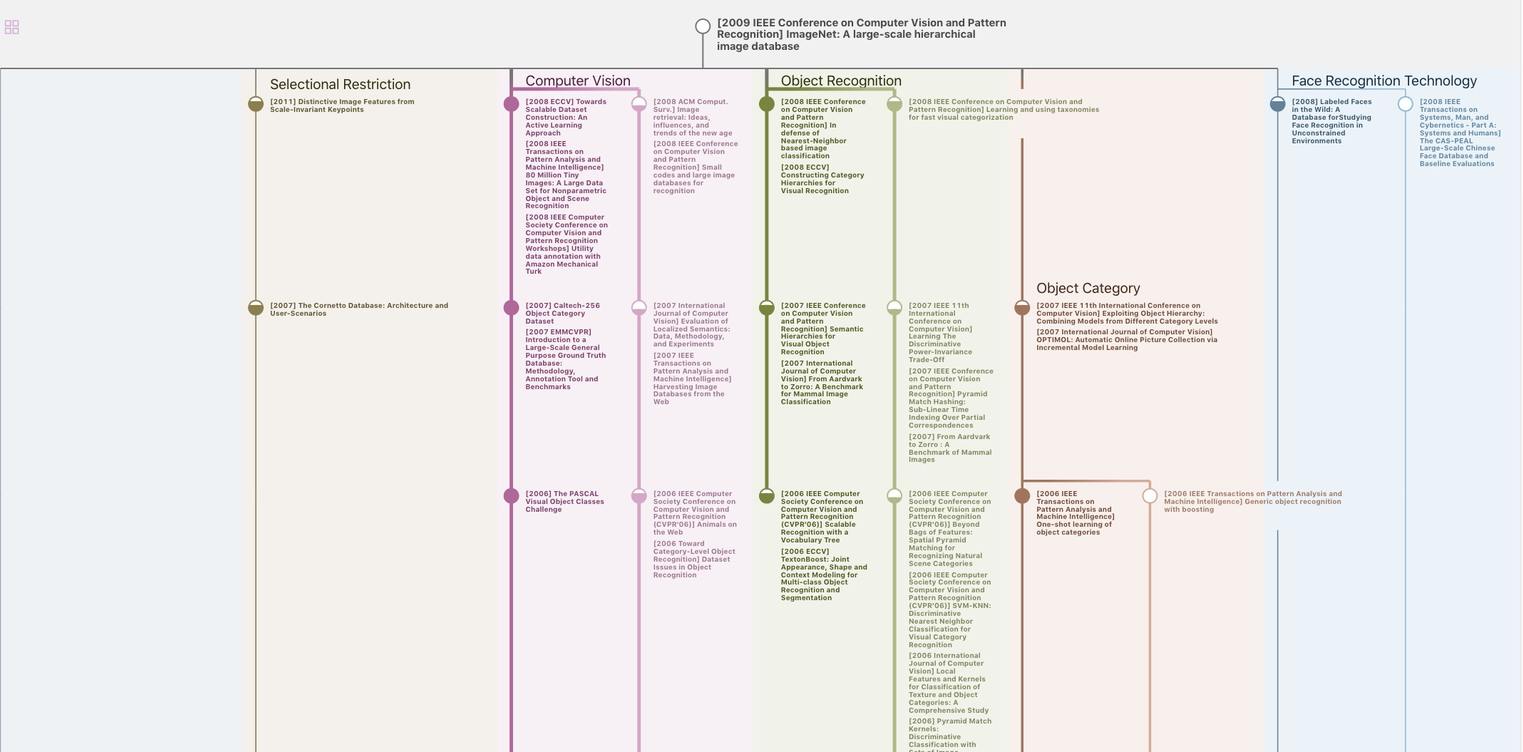
生成溯源树,研究论文发展脉络
Chat Paper
正在生成论文摘要