C^2RV: Cross-Regional and Cross-View Learning for Sparse-View CBCT Reconstruction
CVPR 2024(2024)
摘要
Cone beam computed tomography (CBCT) is an important imaging technologywidely used in medical scenarios, such as diagnosis and preoperative planning.Using fewer projection views to reconstruct CT, also known as sparse-viewreconstruction, can reduce ionizing radiation and further benefitinterventional radiology. Compared with sparse-view reconstruction fortraditional parallel/fan-beam CT, CBCT reconstruction is more challenging dueto the increased dimensionality caused by the measurement process based oncone-shaped X-ray beams. As a 2D-to-3D reconstruction problem, althoughimplicit neural representations have been introduced to enable efficienttraining, only local features are considered and different views are processedequally in previous works, resulting in spatial inconsistency and poorperformance on complicated anatomies. To this end, we propose C^2RV byleveraging explicit multi-scale volumetric representations to enablecross-regional learning in the 3D space. Additionally, the scale-viewcross-attention module is introduced to adaptively aggregate multi-scale andmulti-view features. Extensive experiments demonstrate that our C^2RV achievesconsistent and significant improvement over previous state-of-the-art methodson datasets with diverse anatomy.
更多查看译文
关键词
Cone-Beam CT,Sparse-View Reconstruction,Implicit Neural Representation,CT Reconstruction
AI 理解论文
溯源树
样例
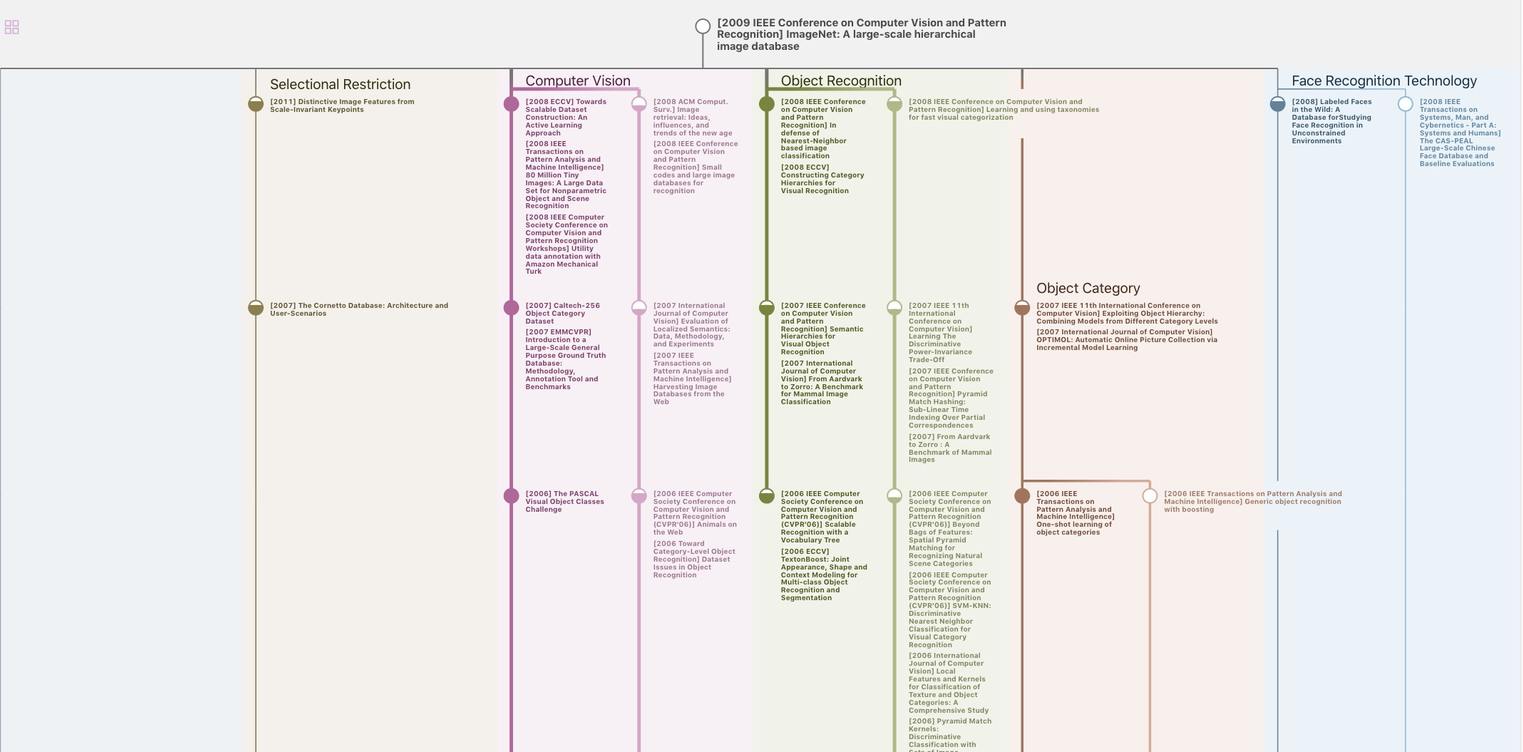
生成溯源树,研究论文发展脉络
Chat Paper
正在生成论文摘要