Machine learning models for predicting the onset of chronic kidney disease after surgery in patients with renal cell carcinoma
BMC Medical Informatics and Decision Making(2024)
摘要
Patients with renal cell carcinoma (RCC) have an elevated risk of chronic kidney disease (CKD) following nephrectomy. Therefore, continuous monitoring and subsequent interventions are necessary. It is recommended to evaluate renal function postoperatively. Therefore, a tool to predict CKD onset is essential for postoperative follow-up and management. We constructed a cohort using data from eight tertiary hospitals from the Korean Renal Cell Carcinoma (KORCC) database. A dataset of 4389 patients with RCC was constructed for analysis from the collected data. Nine machine learning (ML) models were used to classify the occurrence and nonoccurrence of CKD after surgery. The final model was selected based on the area under the receiver operating characteristic (AUROC), and the importance of the variables constituting the model was confirmed using the shapley additive explanation (SHAP) value and Kaplan-Meier survival analyses. The gradient boost algorithm was the most effective among the various ML models tested. The gradient boost model demonstrated superior performance with an AUROC of 0.826. The SHAP value confirmed that preoperative eGFR, albumin level, and tumor size had a significant impact on the occurrence of CKD after surgery. We developed a model to predict CKD onset after surgery in patients with RCC. This predictive model is a quantitative approach to evaluate post-surgical CKD risk in patients with RCC, facilitating improved prognosis through personalized postoperative care.
更多查看译文
关键词
Renal cell carcinoma,Machine learning,Chronic kidney disease,KOrean Renal Cell Carcinoma,Gradient boost
AI 理解论文
溯源树
样例
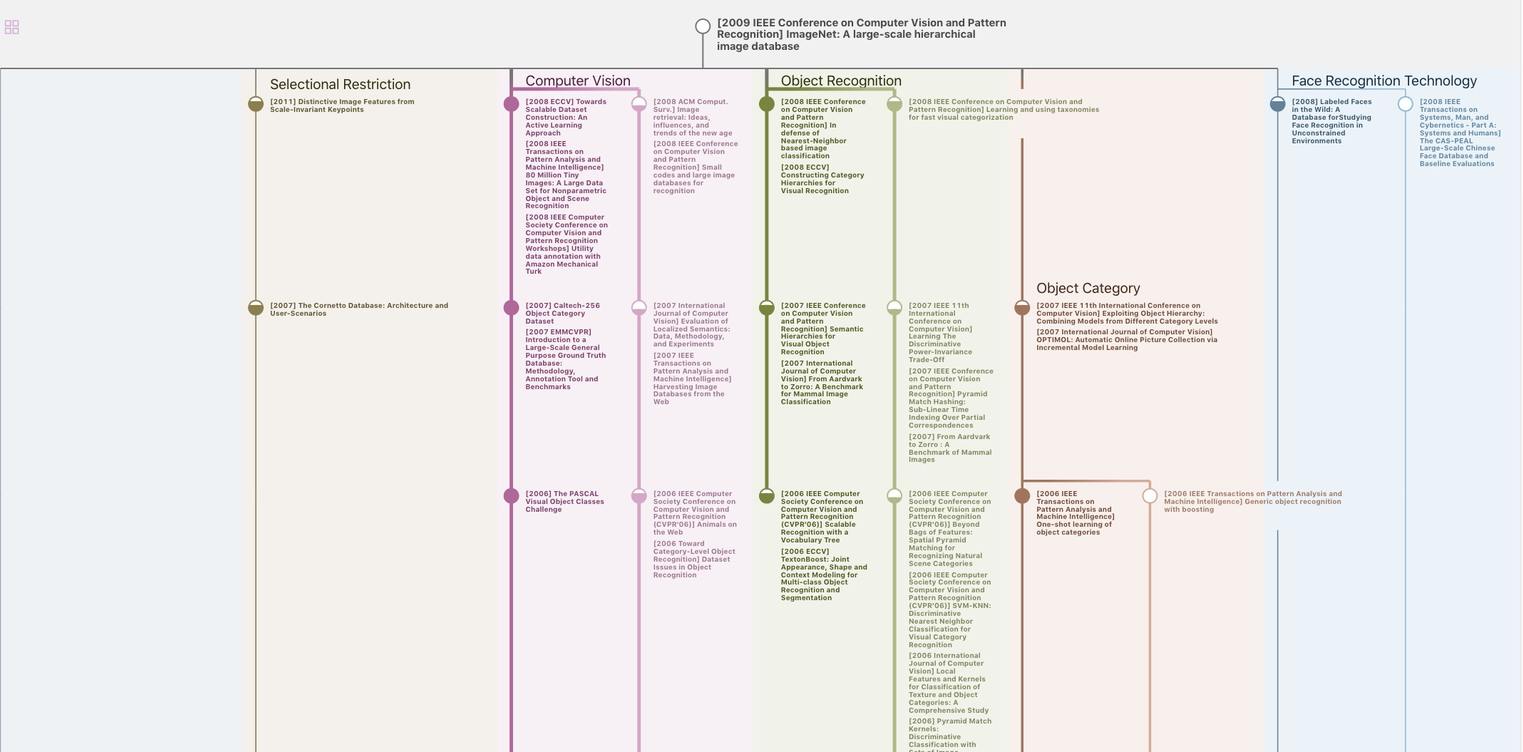
生成溯源树,研究论文发展脉络
Chat Paper
正在生成论文摘要