DR-Label: Label Deconstruction and Reconstruction of GNN Models for Catalysis Systems
AAAI 2024(2024)
摘要
Attaining the equilibrium geometry of a catalyst-adsorbate system is key to fundamentally assessing its effective properties, such as adsorption energy. While machine learning methods with advanced representation or supervision strategies have been applied to boost and guide the relaxation processes of catalysis systems, existing methods that produce linearly aggregated geometry predictions are susceptible to edge representations ambiguity, and are therefore vulnerable to graph variations. In this paper, we present a novel graph neural network (GNN) supervision and prediction strategy DR-Label. Our approach mitigates the multiplicity of solutions in edge representation and encourages model predictions that are independent of graph structural variations. DR-Label first Deconstructs finer-grained equilibrium state information to the model by projecting the node-level supervision signal to each edge. Reversely, the model Reconstructs a more robust equilibrium state prediction by converting edge-level predictions to node-level via a sphere-fitting algorithm. When applied to three fundamentally different models, DR-Label consistently enhanced performance. Leveraging the graph structure invariance of the DR-Label strategy, we further propose DRFormer, which applied explicit intermediate positional update and achieves a new state-of-the-art performance on the Open Catalyst 2020 (OC20) dataset and the Cu-based single-atom alloys CO adsorption (SAA) dataset. We expect our work to highlight vital principles for advancing geometric GNN models for catalysis systems and beyond. Our code is available at https://github.com/bowenwang77/DR-Label
更多查看译文
关键词
ML: Graph-based Machine Learning
AI 理解论文
溯源树
样例
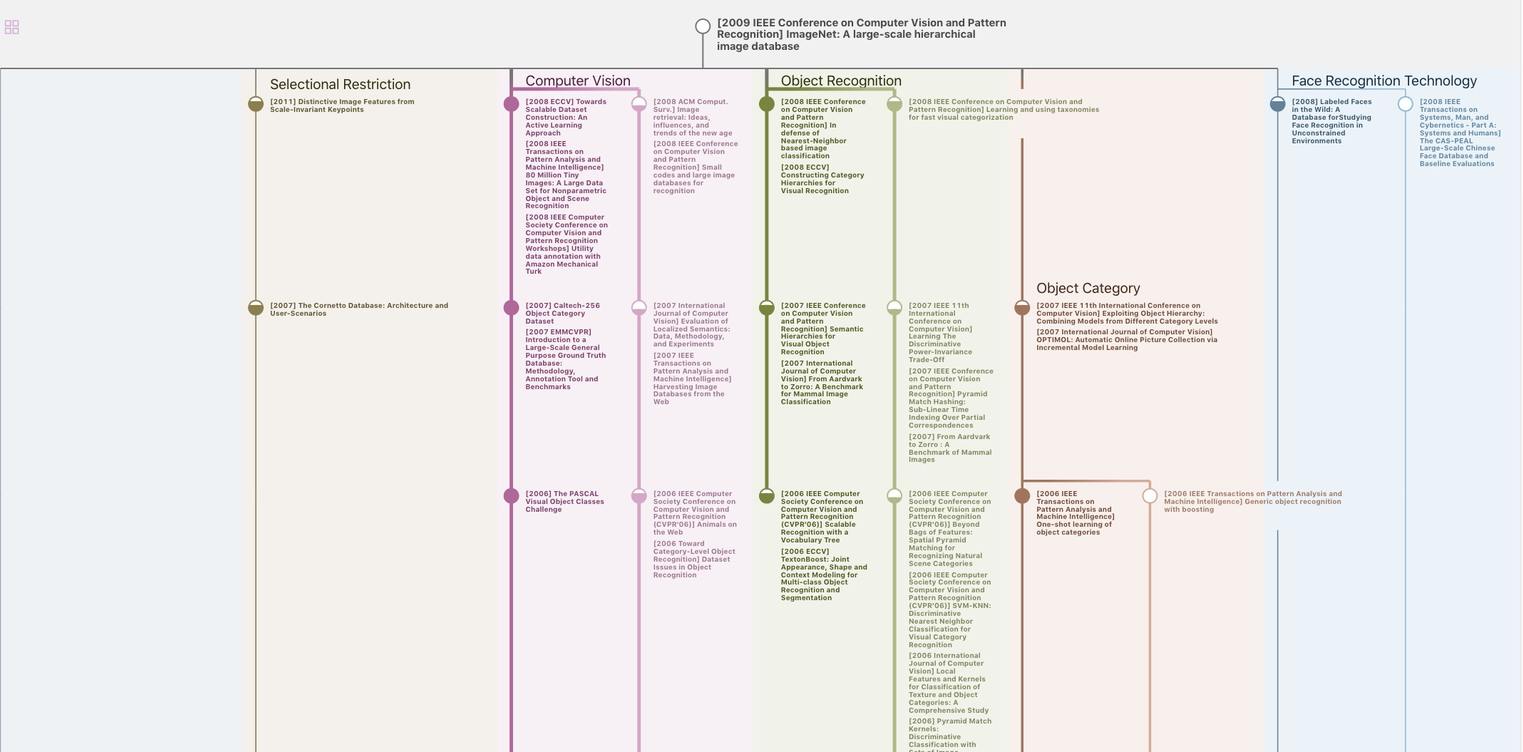
生成溯源树,研究论文发展脉络
Chat Paper
正在生成论文摘要