Exploring Domain Incremental Video Highlights Detection with the LiveFood Benchmark
AAAI 2024(2024)
摘要
Video highlights detection (VHD) is an active research field in computer vision, aiming to locate the most user-appealing clips given raw video inputs. However, most VHD methods are based on the closed world assumption, i.e., a fixed number of highlight categories is defined in advance and all training data are available beforehand. Consequently, existing methods have poor scalability with respect to increasing highlight domains and training data. To address above issues, we propose a novel video highlights detection method named Global Prototype Encoding (GPE) to learn incrementally for adapting to new domains via parameterized prototypes. To facilitate this new research direction, we collect a finely annotated dataset termed LiveFood, including over 5,100 live gourmet videos that consist of four domains: ingredients, cooking, presentation, and eating. To the best of our knowledge, this is the first work to explore video highlights detection in the incremental learning setting, opening up new land to apply VHD for practical scenarios where both the concerned highlight domains and training data increase over time. We demonstrate the effectiveness of GPE through extensive experiments. Notably, GPE surpasses popular domain incremental learning methods on LiveFood, achieving significant mAP improvements on all domains. Concerning the classic datasets, GPE also yields comparable performance as previous arts. The code is available at: https://github.com/ForeverPs/IncrementalVHD_GPE.
更多查看译文
关键词
HAI: Applications,CV: Other Foundations of Computer Vision,CV: Representation Learning for Vision,CV: Video Understanding & Activity Analysis
AI 理解论文
溯源树
样例
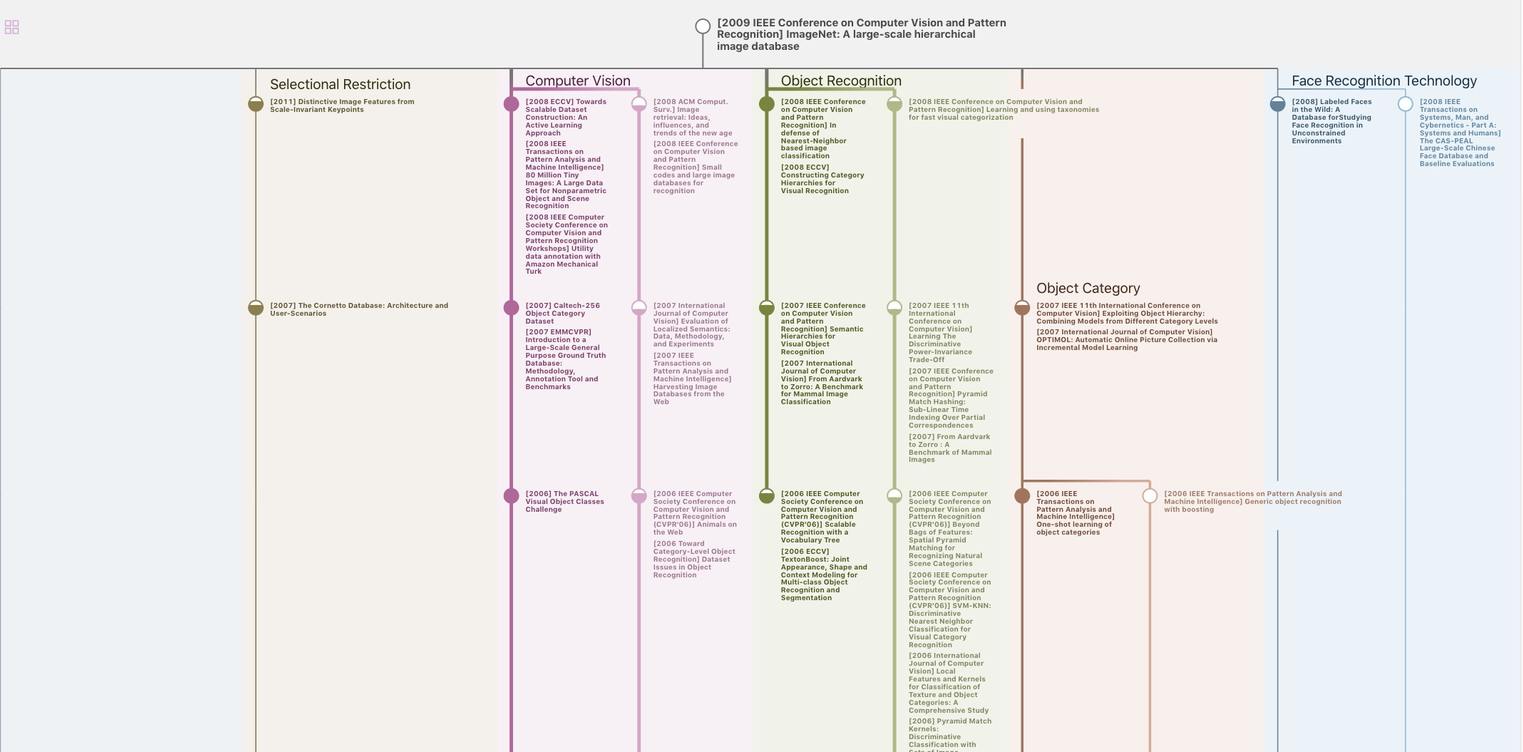
生成溯源树,研究论文发展脉络
Chat Paper
正在生成论文摘要