CatmullRom Splines-Based Regression for Image Forgery Localization
THIRTY-EIGHTH AAAI CONFERENCE ON ARTIFICIAL INTELLIGENCE, VOL 38 NO 7(2024)
摘要
IFL (Image Forgery Location) helps secure digital media forensics. However, many methods suffer from false detections (i.e., FPs) and inaccurate boundaries. In this paper, we proposed the CatmullRom Splines-based Regression Network (CSR-Net), which first rethinks the IFL task from the perspective of regression to deal with this problem. Specifically speaking, we propose an adaptive CutmullRom splines fitting scheme for coarse localization of the tampered regions. Then, for false positive cases, we first develop a novel re-scoring mechanism, which aims to filter out samples that cannot have responses on both the classification branch and the instance branch. Later on, to further restrict the boundaries, we design a learnable texture extraction module, which refines and enhances the contour representation by decoupling the horizontal and vertical forgery features to extract a more robust contour representation, thus suppressing FPs. Compared to segmentation-based methods, our method is simple but effective due to the unnecessity of post-processing. Extensive experiments show the superiority of CSR-Net to existing state-of-the-art methods, not only on standard natural image datasets but also on social media datasets.
更多查看译文
关键词
Image Forgery Detection,Resampling Detection,Splicing Detection,Tampering Localization,Representation Learning
AI 理解论文
溯源树
样例
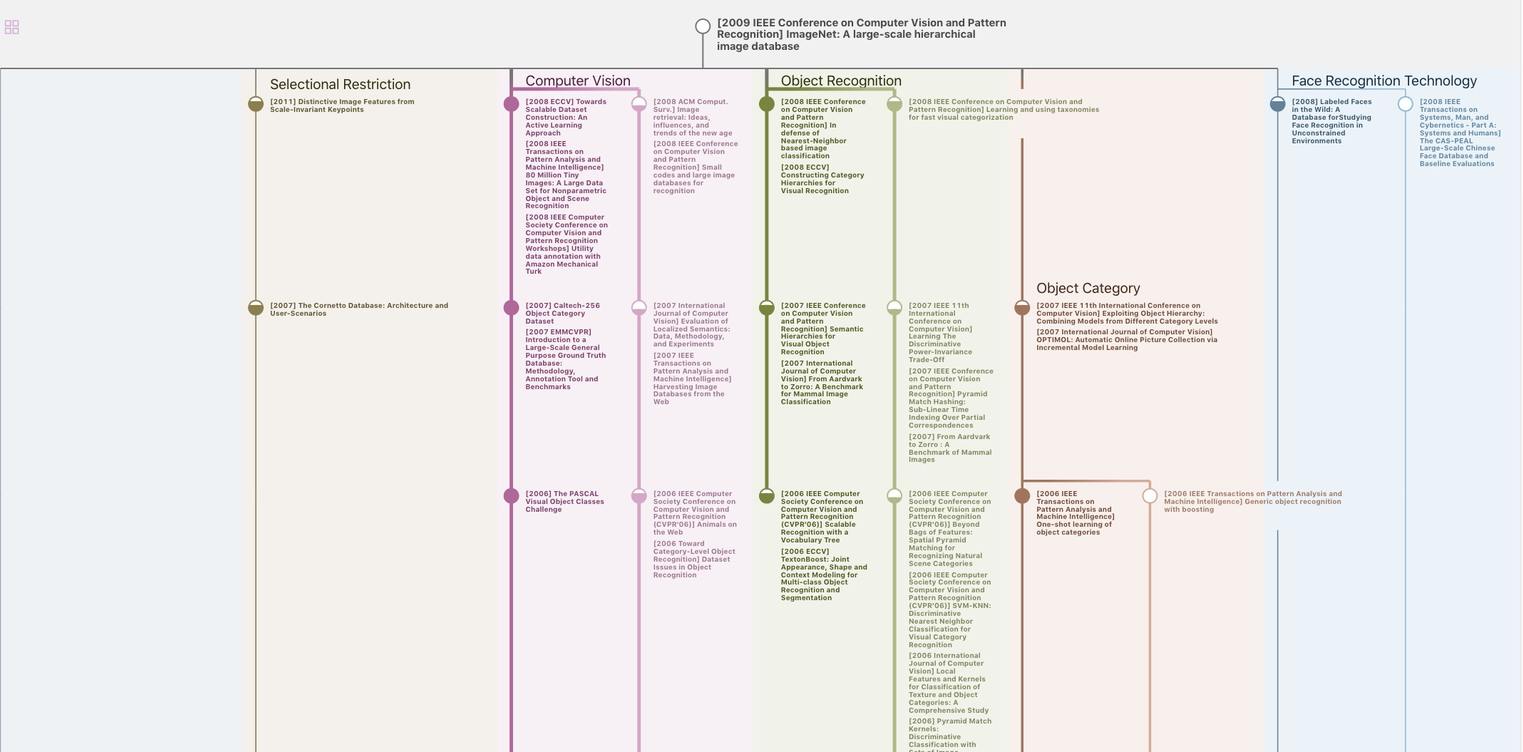
生成溯源树,研究论文发展脉络
Chat Paper
正在生成论文摘要