π-Light: Programmatic Interpretable Reinforcement Learning for Resource-Limited Traffic Signal Control
AAAI 2024(2024)
摘要
The recent advancements in Deep Reinforcement Learning (DRL) have significantly enhanced the performance of adaptive Traffic Signal Control (TSC). However, DRL policies are typically represented by neural networks, which are over-parameterized black-box models. As a result, the learned policies often lack interpretability, and cannot be deployed directly in the real-world edge hardware due to resource constraints. In addition, the DRL methods often exhibit limited generalization performance, struggling to generalize the learned policy to other geographical regions. These factors limit the practical application of learning-based approaches. To address these issues, we suggest the use of an inherently interpretable program for representing the control policy. We present a new approach, Programmatic Interpretable reinforcement learning for traffic signal control (π-light), designed to autonomously discover non-differentiable programs. Specifically, we define a Domain Specific Language (DSL) and transformation rules for constructing programs, and utilize Monte Carlo Tree Search (MCTS) to find the optimal program in a discrete space. Extensive experiments demonstrate that our method consistently outperforms baseline approaches. Moreover, π-Light exhibits superior generalization capabilities compared to DRL, enabling training and evaluation across intersections from different cities. Finally, we analyze how the learned program policies can directly deploy on edge devices with extremely limited resources.
更多查看译文
关键词
General
AI 理解论文
溯源树
样例
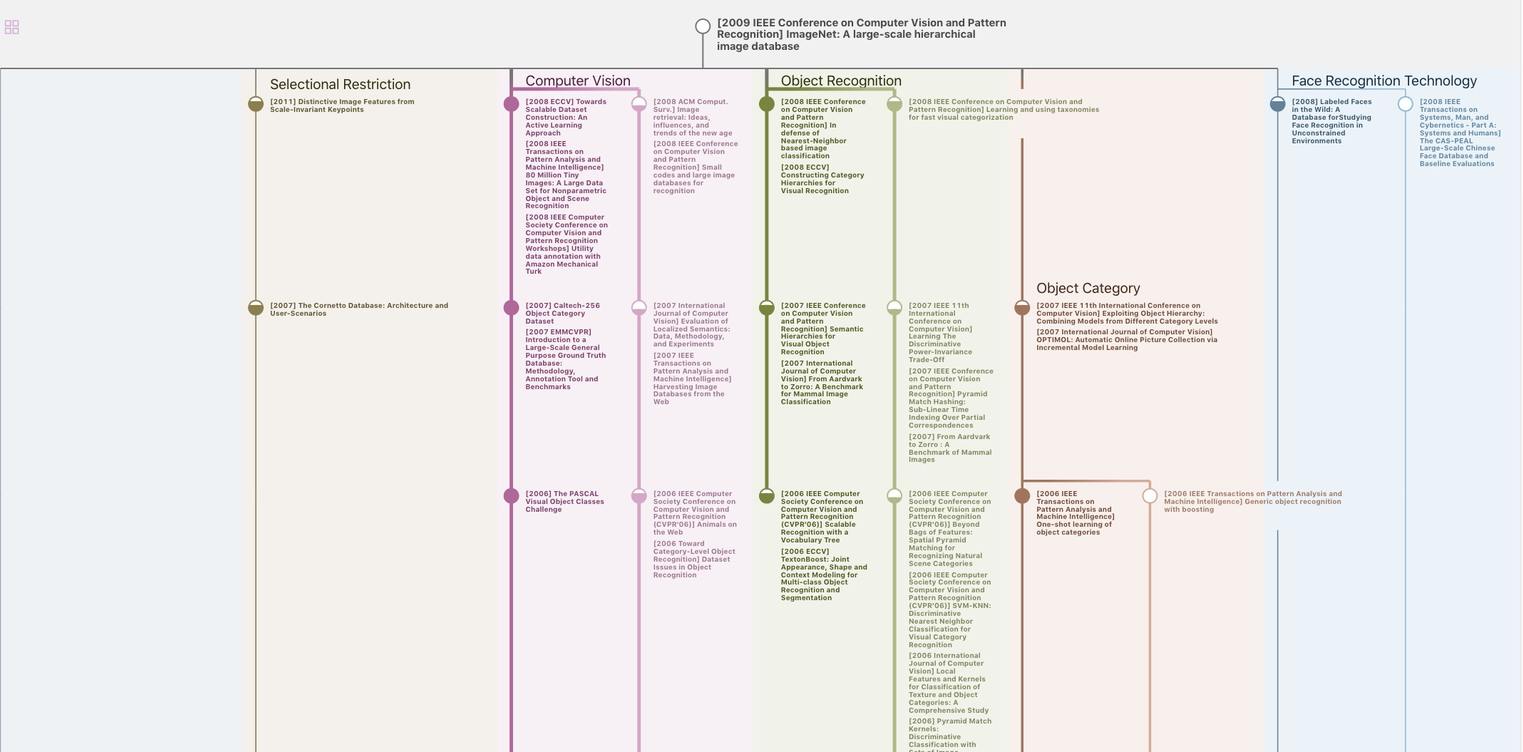
生成溯源树,研究论文发展脉络
Chat Paper
正在生成论文摘要