Hawkes-Enhanced Spatial-Temporal Hypergraph Contrastive Learning Based on Criminal Correlations
AAAI 2024(2024)
摘要
Crime prediction is a crucial yet challenging task within urban computing, which benefits public safety and resource optimization. Over the years, various models have been proposed, and spatial-temporal hypergraph learning models have recently shown outstanding performances. However, three correlations underlying crime are ignored, thus hindering the performance of previous models. Specifically, there are two spatial correlations and one temporal correlation, i.e., (1) co-occurrence of different types of crimes (type spatial correlation), (2) the closer to the crime center, the more dangerous it is around the neighborhood area (neighbor spatial correlation), and (3) the closer between two timestamps, the more relevant events are (hawkes temporal correlation). To this end, we propose Hawkes-enhanced Spatial-Temporal Hypergraph Contrastive Learning framework (HCL), which mines the aforementioned correlations via two specific strategies. Concretely, contrastive learning strategies are designed for two spatial correlations, and hawkes process modeling is adopted for temporal correlations. Extensive experiments demonstrate the promising capacities of HCL from four aspects, i.e., superiority, transferability, effectiveness, and sensitivity.
更多查看译文
关键词
DMKM: Mining of Spatial, Temporal or Spatio-Temporal Data,DMKM: Graph Mining, Social Network Analysis & Community,ML: Applications
AI 理解论文
溯源树
样例
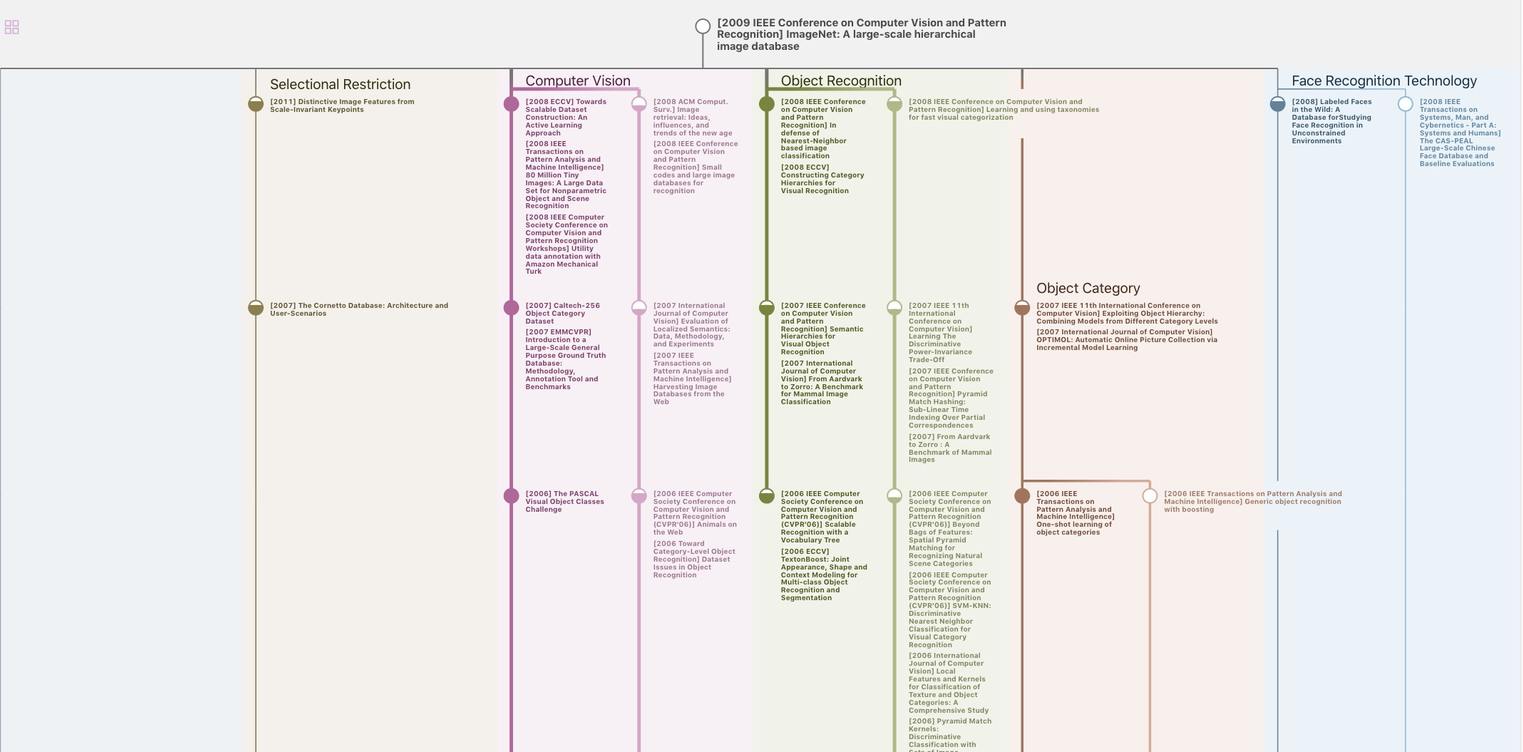
生成溯源树,研究论文发展脉络
Chat Paper
正在生成论文摘要