Decoding Global Preferences: Temporal and Cooperative Dependency Modeling in Multi-Agent Preference-Based Reinforcement Learning
AAAI 2024(2024)
摘要
Designing accurate reward functions for reinforcement learning (RL) has long been challenging. Preference-based RL (PbRL) offers a promising approach by using human preferences
to train agents, eliminating the need for manual reward design. While successful in single-agent tasks, extending PbRL to complex multi-agent scenarios is nontrivial. Existing PbRL methods lack the capacity to comprehensively capture both temporal and cooperative aspects, leading to inadequate reward functions. This work introduces an advanced multi-agent preference learning framework that effectively addresses these limitations. Based on a cascading Transformer architecture, our approach captures both temporal and cooperative dependencies, alleviating issues related to reward uniformity and intricate interactions among agents. Experimental results demonstrate substantial performance improvements in multi-agent cooperative tasks, and the reconstructed reward function closely resembles expert-defined reward functions. The source code is available at https://github.com/catezi/MAPT.
更多查看译文
关键词
ML: Reinforcement Learning,MAS: Multiagent Learning
AI 理解论文
溯源树
样例
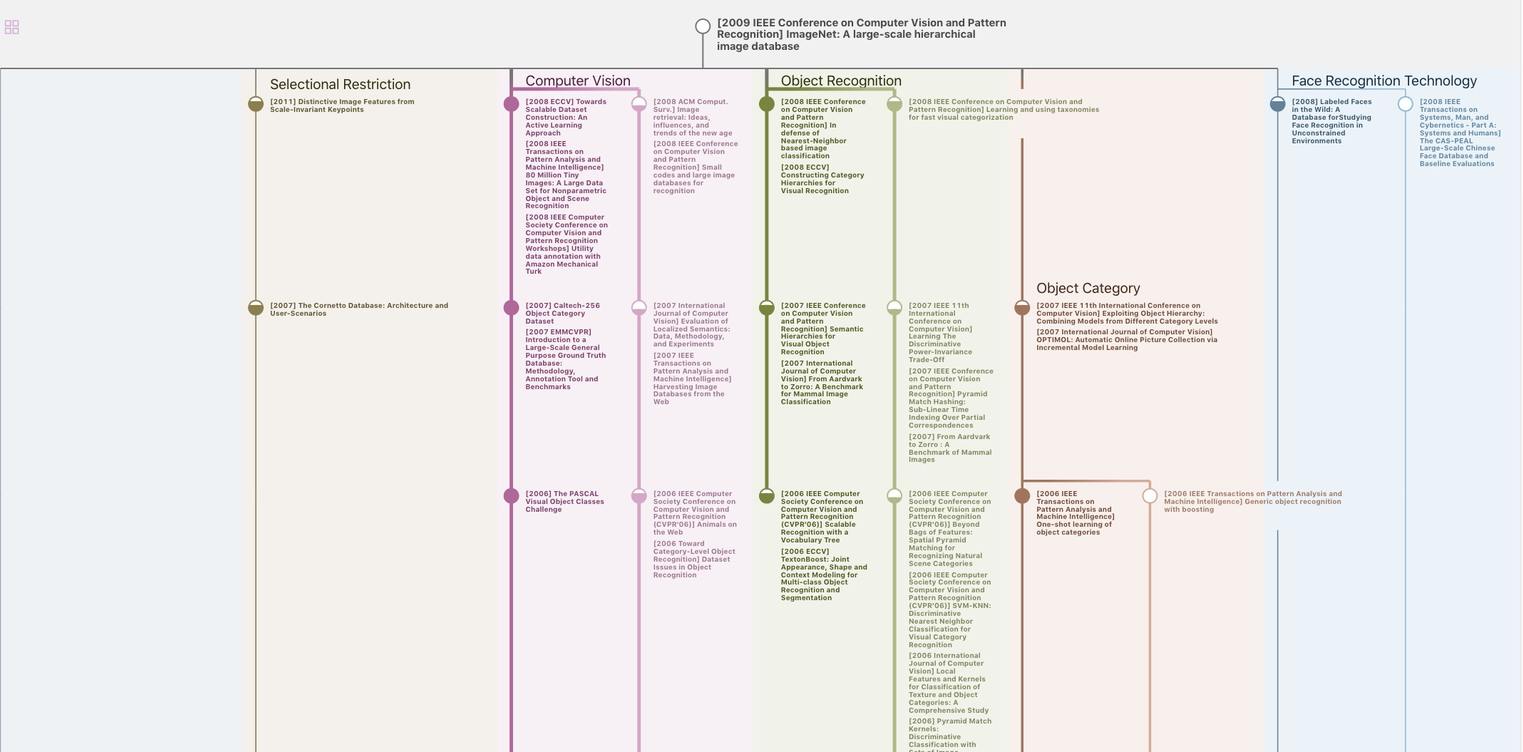
生成溯源树,研究论文发展脉络
Chat Paper
正在生成论文摘要