LWSA: A Learning-Based Workflow Scheduling Algorithm for Energy-Efficient UAV Delivery System.
International Conference on Parallel and Distributed Systems(2023)
摘要
Due to their fast speed and easy deployment, Unmanned Aerial Vehicles (UAVs) have been widely used across various sectors, such as earthquake rescue, medical assistance, and smart agriculture. However, UAVs in delivery networks face significant challenges due to limited battery life and computational capabilities, particularly for tasks that entail intensive computing workflows. In this context, Multi-access Edge Computing (MEC), which provides computing resources in close proximity to mobile terminal devices, has emerged as a promising solution. UAVs can offload computing tasks to MEC resources across diverse Internet of Things (IoT) environments. Although task offloading can enhance their task processing capability, it simultaneously brings additional costs, encompassing data transmission time and energy consumption. To address these issues, this paper proposes a novel workflow scheduling method based on the Proximal Policy Optimization (PPO) algorithm, aimed at optimizing UAV energy consumption within MEC environments. The proposed approach establishes a learning-based workflow scheduling strategy harnessing the adaptability of the PPO algorithm to manage dynamic and intricate scenarios, which facilitates efficient task allocation to optimal computational resources while accounting for flight time constraints. Extensive experiments conducted on various well-known scientific workflow benchmarks in real-world UAV delivery networks validate the effectiveness of our method. Compared with state-of-the-art methods, our approach significantly reduces UAV energy consumption and task completion time, simultaneously increasing UAV’s effective payload capacity.
更多查看译文
关键词
Energy Efficiency,Multi-Access Edge Computing,Reinforcement Learning,Workflow Scheduling
AI 理解论文
溯源树
样例
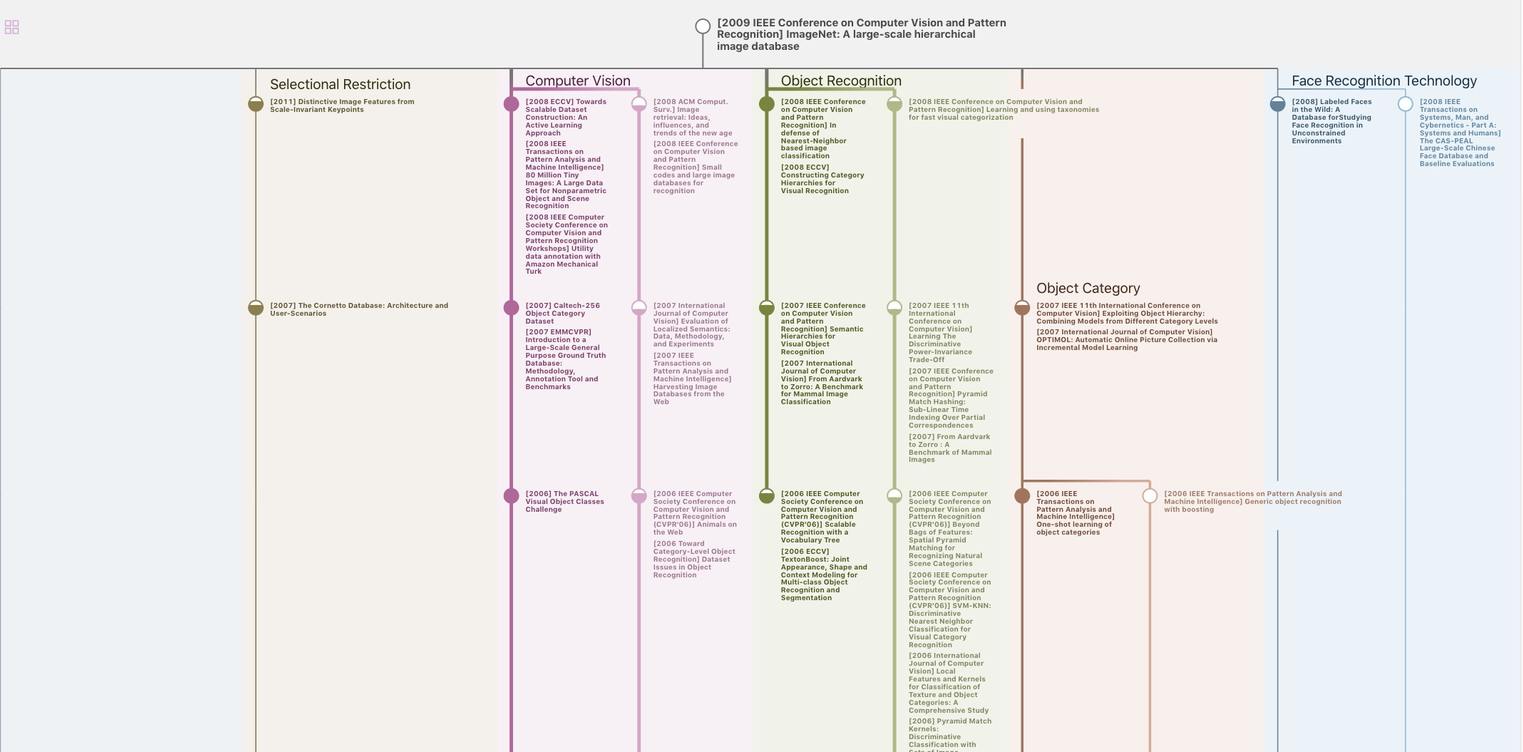
生成溯源树,研究论文发展脉络
Chat Paper
正在生成论文摘要