Fairness with adaptive weight in network attack detection.
International Conference on Parallel and Distributed Systems(2023)
摘要
Network attacks aim to exploit vulnerabilities inherent in network protocols, which is widely used in many real-world applications. In the process of network anomaly detection, most methods train the model by minimizing the average empirical risk of all samples. However, due to the uneven distribution of samples from different protocols, detection models tend to be biased against minority protocols groups. To address this issue, we propose an adaptive weight assignment method for network attack detection, which emphasizes more on error-prone samples in prediction and enhances adequate representation of minority groups for fairness. We conduct experiments on two widely used datasets, KDD and NSLKDD. According to the results, our method achieves better performance than state-of-the-art methods for classification and regression tasks, and is robust to label noise in the test dataset.
更多查看译文
关键词
Attack detection,Fairness,Adaptive weight
AI 理解论文
溯源树
样例
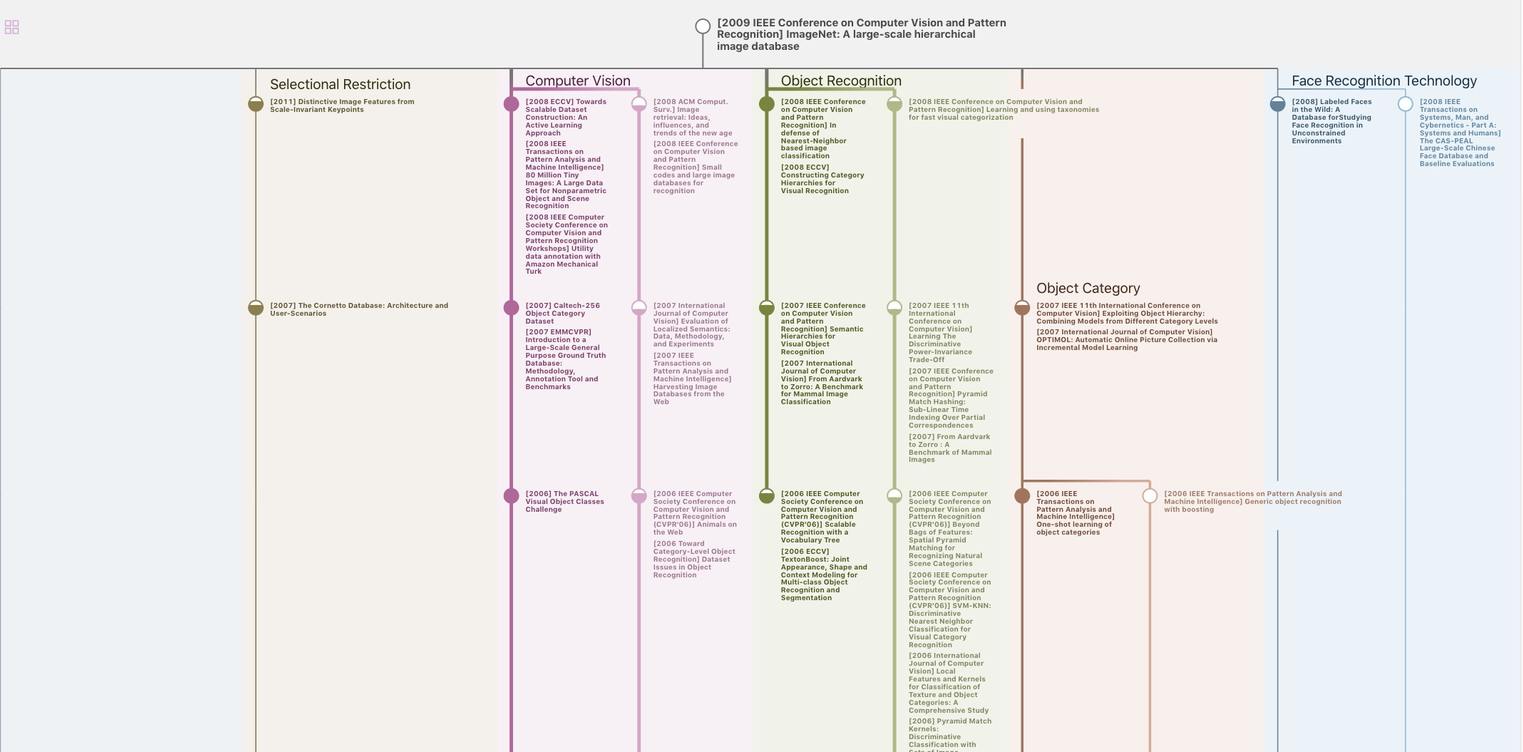
生成溯源树,研究论文发展脉络
Chat Paper
正在生成论文摘要