IterAlign: Iterative Constitutional Alignment of Large Language Models
arXiv (Cornell University)(2024)
摘要
With the rapid development of large language models (LLMs), aligning LLMswith human values and societal norms to ensure their reliability and safety hasbecome crucial. Reinforcement learning with human feedback (RLHF) andConstitutional AI (CAI) have been proposed for LLM alignment. However, thesemethods require either heavy human annotations or explicitly pre-definedconstitutions, which are labor-intensive and resource-consuming. To overcomethese drawbacks, we study constitution-based LLM alignment and propose adata-driven constitution discovery and self-alignment framework calledIterAlign. IterAlign leverages red teaming to unveil the weaknesses of an LLMand automatically discovers new constitutions using a stronger LLM. Theseconstitutions are then used to guide self-correction of the base LLM. Such aconstitution discovery pipeline can be run iteratively and automatically todiscover new constitutions that specifically target the alignment gaps in thecurrent LLM. Empirical results on several safety benchmark datasets andmultiple base LLMs show that IterAlign successfully improves truthfulness,helpfulness, harmlessness and honesty, improving the LLM alignment by up to13.5% in harmlessness.
更多查看译文
AI 理解论文
溯源树
样例
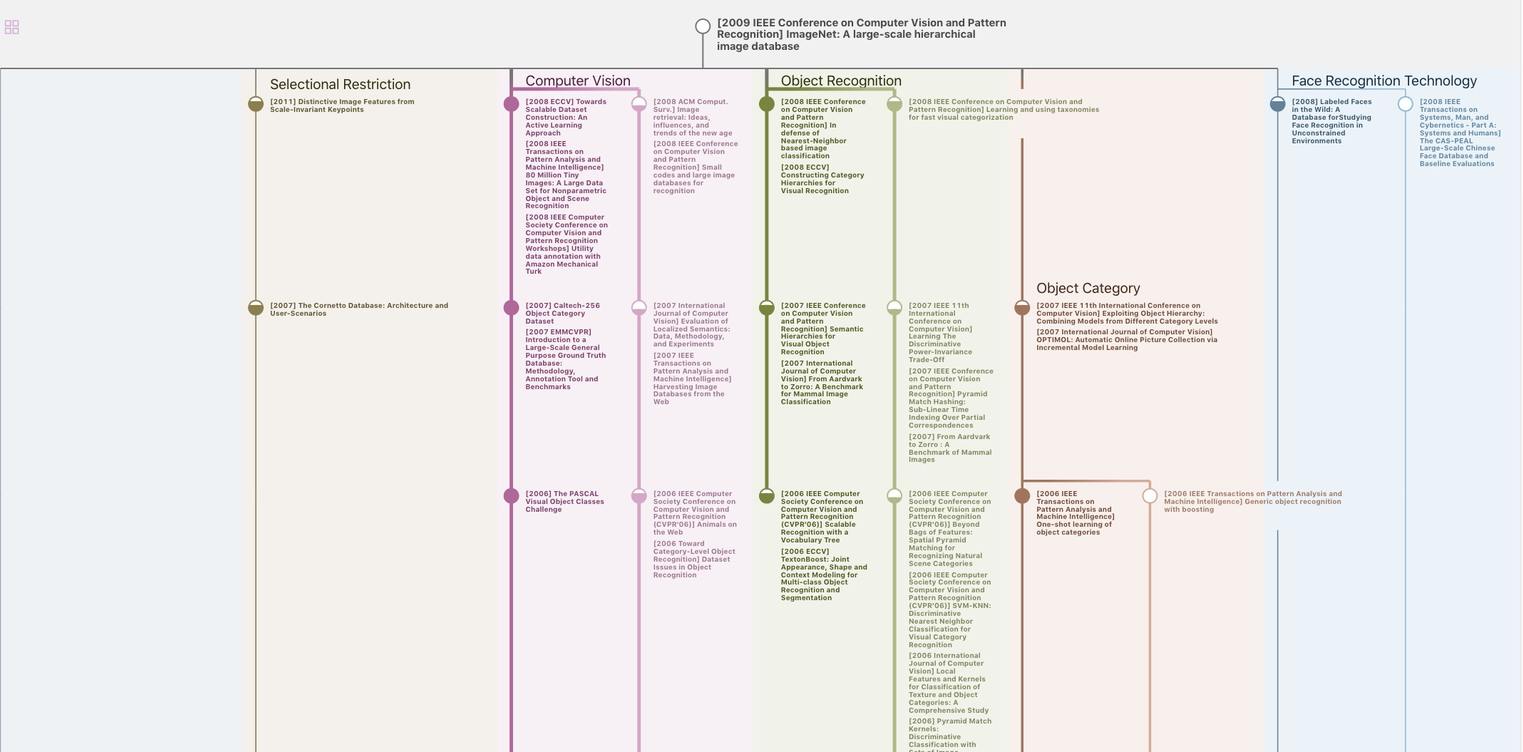
生成溯源树,研究论文发展脉络
Chat Paper
正在生成论文摘要