The electrostatic landscape of MHC-peptide binding revealed using inception networks
Cell Systems(2024)
摘要
Predictive modeling of macromolecular recognition and protein-protein complementarity represents one of the cornerstones of biophysical sciences. However, such models are often hindered by the combinatorial complexity of interactions at the molecular interfaces. Exemplary of this problem is peptide presentation by the highly polymorphic major histocompatibility complex class I (MHC-I) molecule, a principal component of immune recognition. We developed human leukocyte antigen (HLA)-Inception, a deep biophysical convolutional neural network, which integrates molecular electrostatics to capture non-bonded interactions for predicting peptide binding motifs across 5,821 MHC-I alleles. These predictions of generated motifs correlate strongly with experimental peptide binding and presentation data. Beyond molecular interactions, the study demonstrates the application of predicted motifs in analyzing MHC-I allele associations with HIV disease progression and patient response to immune checkpoint inhibitors. A record of this paper’s transparent peer review process is included in the supplemental information.
更多查看译文
关键词
molecular immunology,major histocompatibility complexes,continuum electrostatics,inception learning,convolutional neural network,T cell therapies,multiscale modeling,computational biology,deep learning,immunophysics
AI 理解论文
溯源树
样例
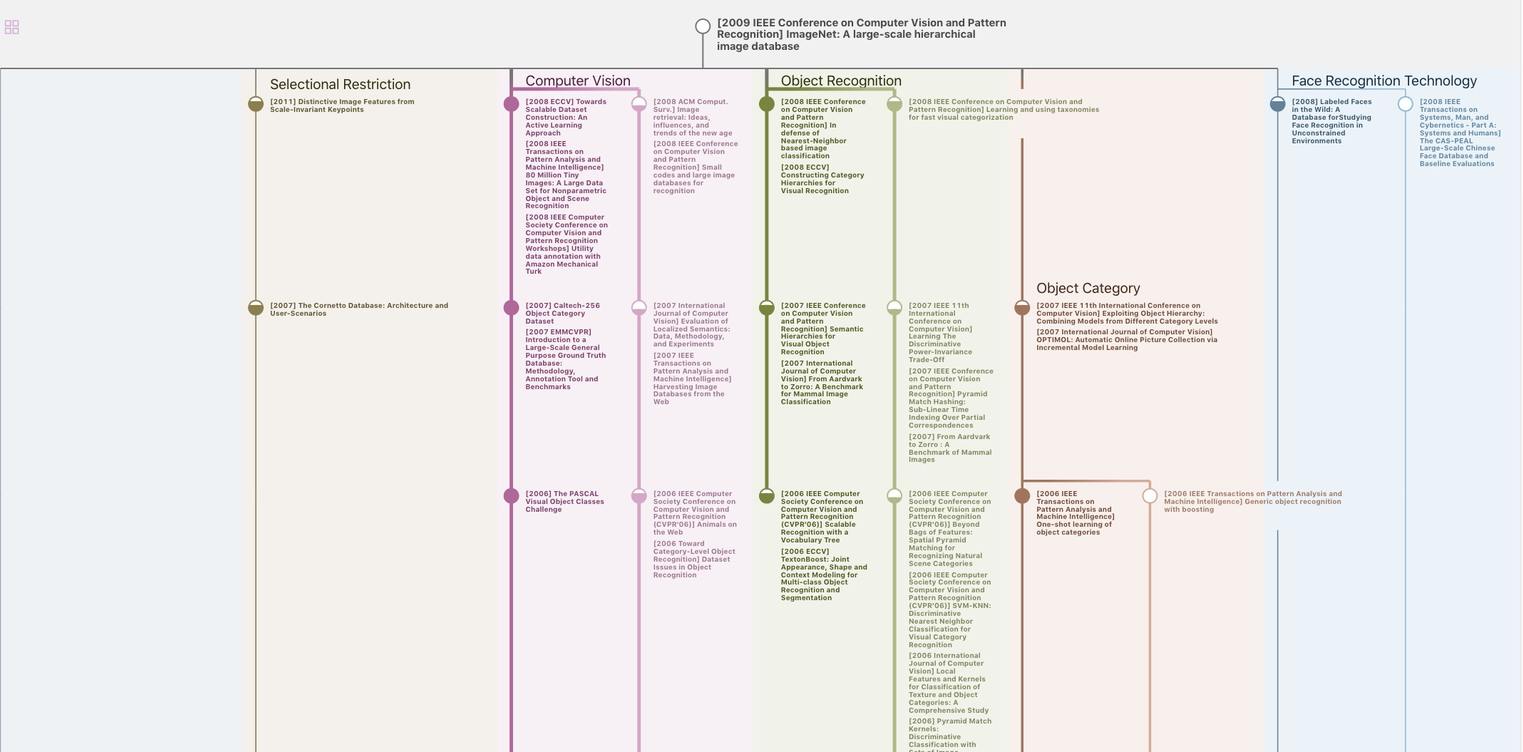
生成溯源树,研究论文发展脉络
Chat Paper
正在生成论文摘要