Discriminative and robust least squares regression for semi-supervised imageclassification
NEUROCOMPUTING(2024)
摘要
Due to the ability to leverage information from both unlabeled and labeled data, semi-supervised classificationhas found extensive applications in various practical scenarios. However, there are two major drawbacks: (1)Traditional graph construction leads to high algorithmic complexity, which limits efficiency. (2) The decisionboundary might be blurred by boundary points in common cases. To cope with these issues, inspired byclassical Least Squares Regression (LSR), we present a novel semi-supervised classification algorithm termedas Discriminative and Robust LSR (DRLSR) for semi-supervised image classification. First of all, a manifoldregularization term is designed and introduced to an LSR-based semi-supervised method to preserve localmanifold structures and smooth structures of the subspace, which strengthens the discrimination ability.Meanwhile, preservation of local manifold structures also contributes to restrain decision boundaries frombeing blurred by boundary points, which strengthens robustness of the algorithm. After that, an efficientalternative optimization method is applied to our algorithm. Evidence of the effectiveness of DRLSR arecompelled by extensive experimental results.
更多查看译文
关键词
Semi-supervised classification,Least Squares Regression,Manifold regularization,Discrimination,Decision boundary,Robustness
AI 理解论文
溯源树
样例
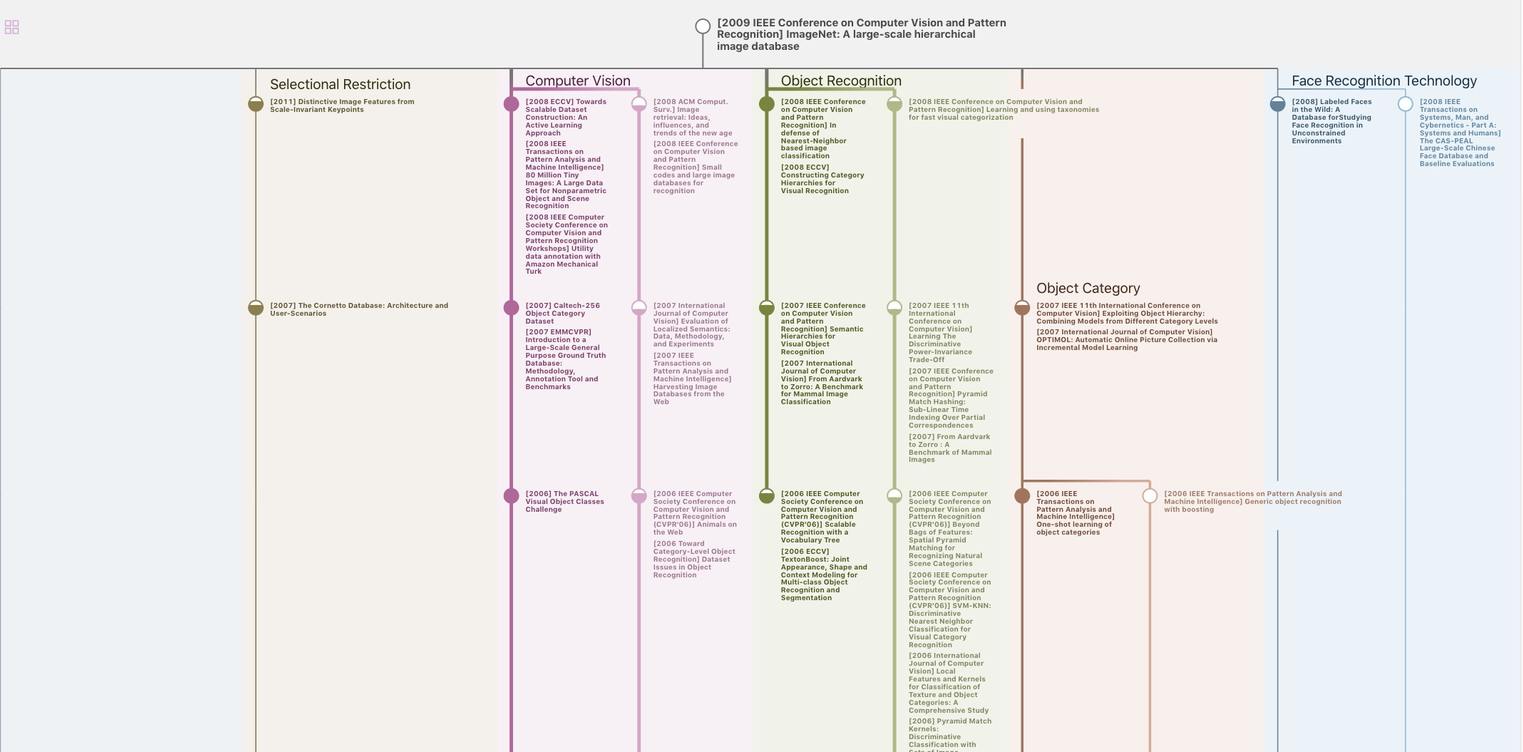
生成溯源树,研究论文发展脉络
Chat Paper
正在生成论文摘要