Self-supervised learning of monocular depth and ego-motion estimation for non-rigid scenes in wireless capsule endoscopy videos
BIOMEDICAL SIGNAL PROCESSING AND CONTROL(2024)
摘要
Background and objective: Gastrointestinal (GI) cancers represent the most widespread type of cancer worldwide. Wireless capsule endoscopy (WCE), an innovative, capsule -sized endoscope, has the potential to revolutionize both the diagnosis and treatment of GI cancers as well as other GI diseases by offering patients a less invasive and more comfortable option. Nonetheless, WCE videos frequently display non -rigid transformations and brightness fluctuations, rendering prior simultaneous localization and mapping (SLAM) approaches unfeasible. The depth can assist in recognizing and monitoring potential obstructions or anomalies when localization is required. Methods: In this paper, we present a self -supervised model, SfMLearner-WCE, specifically designed for estimating depth and ego motion in WCE videos. Our approach incorporates a pose estimation network and a Transformer network with a global self -attention mechanism. To ensure high -quality depth and pose estimation, we propose learnable binary per -pixel masks to eliminate misaligned image regions arising from non -rigid transformations or significant changes in lighting. Additionally, we introduce multi -interval frame sampling to enhance training data diversity, coupled with long-term pose consistency regularization. Results: We present a comprehensive evaluation of the performance of SfMLearner-WCE in comparison with five state-of-the-art self -supervised SLAM methods. Our proposed approach is rigorously assessed on three WCE datasets. The experimental results demonstrate our approach achieves high -quality depth estimation and high -precision ego -motion estimation for non -rigid scenes in WCE videos, outperforming other self -supervised SLAM methods. In the quantitative evaluation of depth estimation using the ColonDepth dataset, an absolute relative error of 0.232 was observed. Additionally, during the quantitative assessment of ego -motion estimation on the ColonSim dataset, a translation drift percentage of 43.176% was achieved at a frame rate of 2 frames per second. Conclusions: The experimental analysis conducted in this study offers evidence of the effectiveness and robustness of our proposed method, SfMLearner-WCE, in non -rigid scenes of WCE videos. SfMLearner-WCE assists in enhancing diagnostic efficiency, enabling physicians to navigate and analyze WCE videos more effectively, benefiting patient outcomes. Our code will be released at https://github.com/fisherliaoc/SfMLearner-WCE.
更多查看译文
关键词
Wireless capsule endoscopy (WCE) images,Monocular depth estimation,Ego-motion estimation,Non-rigid scenes,Transformer
AI 理解论文
溯源树
样例
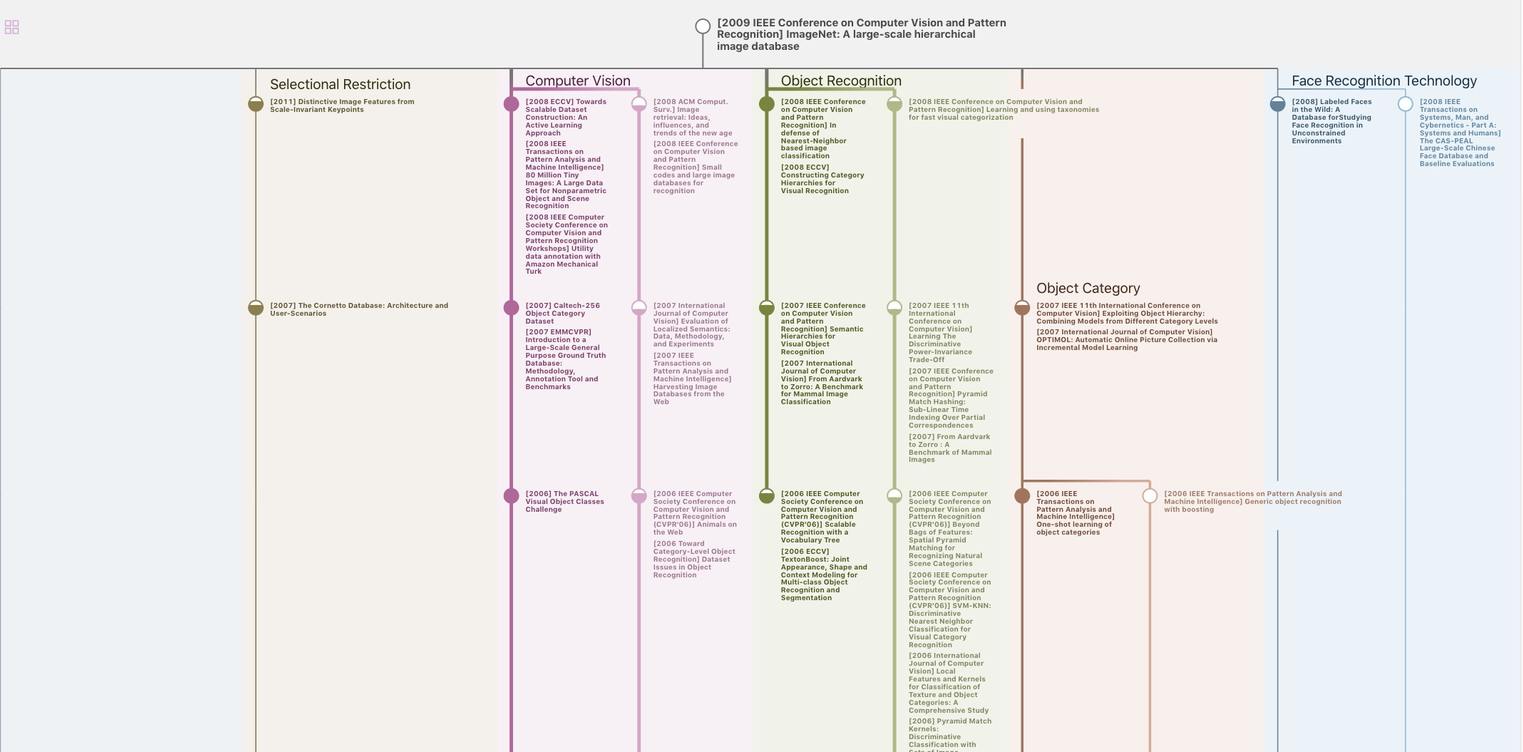
生成溯源树,研究论文发展脉络
Chat Paper
正在生成论文摘要