Multi-Agent Reinforcement Learning for Coordinating Communication and Control
IEEE TRANSACTIONS ON COGNITIVE COMMUNICATIONS AND NETWORKING(2024)
摘要
The automation of factories and manufacturing processes has been accelerating over the past few years, leading to an ever-increasing number of scenarios with networked agents whose coordination requires reliable wireless communication. In this context, goal-oriented communication adapts transmissions to the control task, prioritizing the more relevant information to decide which action to take. Instead, networked control models follow the opposite pathway, optimizing physical actions to address communication impairments. In this work, we propose a joint design that combines goal-oriented communication and networked control into a single optimization model, an extension of a multi-agent Partially Observable Markov Decision Process (POMDP), which we call Cyber-Physical POMDP. The proposed model is flexible enough to represent a large variety of scenarios and we illustrate its potential in two simple use cases with a single agent and a set of supporting sensors. Our results assess that the joint optimization of communication and control tasks radically improves the performance of networked control systems, particularly in the case of constrained resources, leading to implicit coordination of communication actions.
更多查看译文
关键词
Optimization,Networked control systems,Sensors,Quality of service,Process control,Wireless communication,Robot sensing systems,Markov decision processes,networked control systems,goal-oriented communications,multi-agent reinforcement learning
AI 理解论文
溯源树
样例
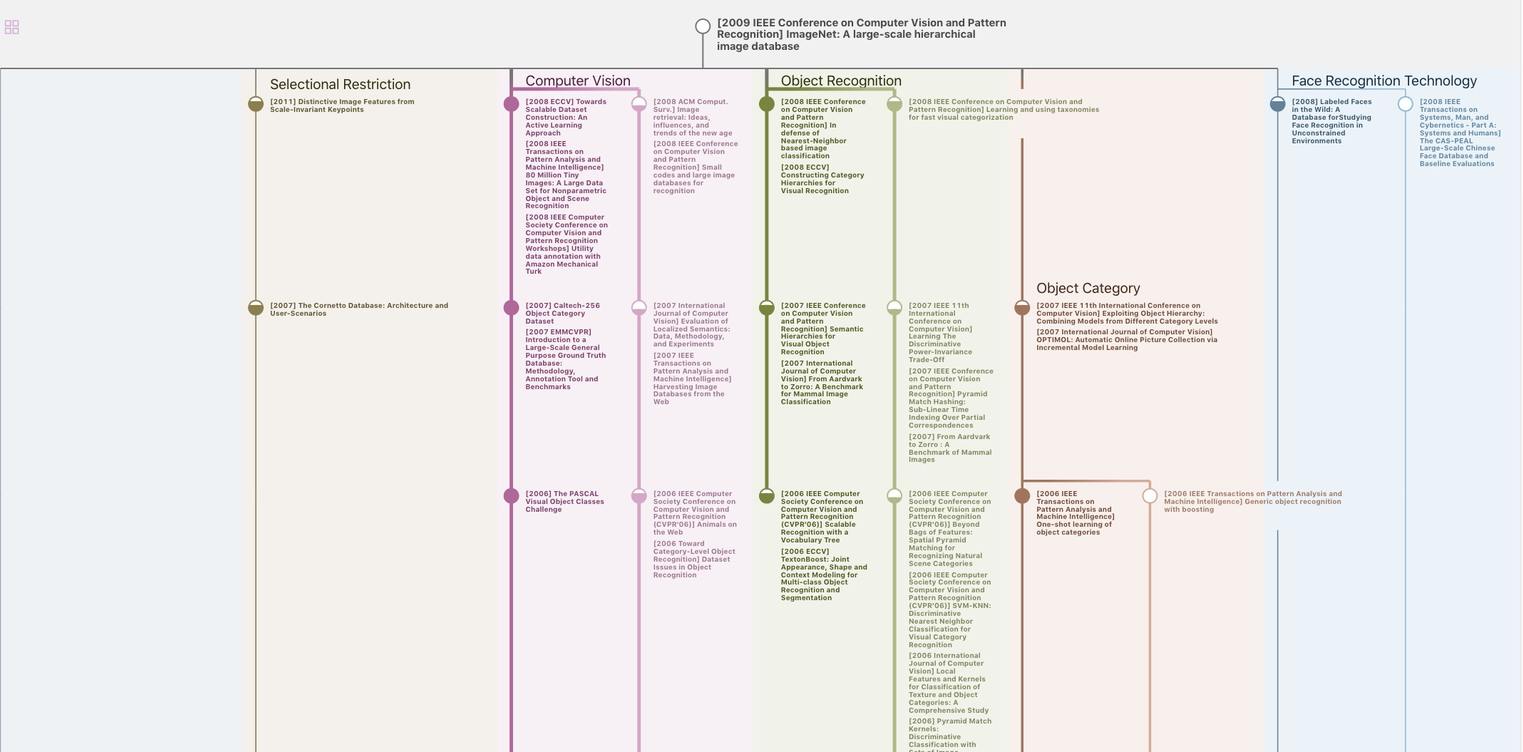
生成溯源树,研究论文发展脉络
Chat Paper
正在生成论文摘要