Granger causal representation learning for groups of time series
Science China Information Sciences(2024)
摘要
Discovering causality from multivariate time series is an important but challenging problem. Most existing methods focus on estimating the Granger causal structures among multivariate time series, while ignoring the prior knowledge of these time series, e.g., the group of the time series. Focusing on discovering the Granger causal structures among groups of time series, we propose a Granger causal representation learning method to solve this problem. First, we use the multiset canonical correlation analysis method to learn the Granger causal representation of each group of time series. Then, we model the Granger causal relationships among the learned Granger causal representations using a recurrent neural network with temporal information. Finally, we formulate the above two stages into one unified optimization problem, which is efficiently solved using the augmented Lagrangian method. We conduct extensive experiments on synthetic and real-world datasets to validate the correctness and effectiveness of the proposed method.
更多查看译文
关键词
Granger causal discovery,Granger causal representation learning,time series data,recurrent neural network,multiset canonical correlation analysis
AI 理解论文
溯源树
样例
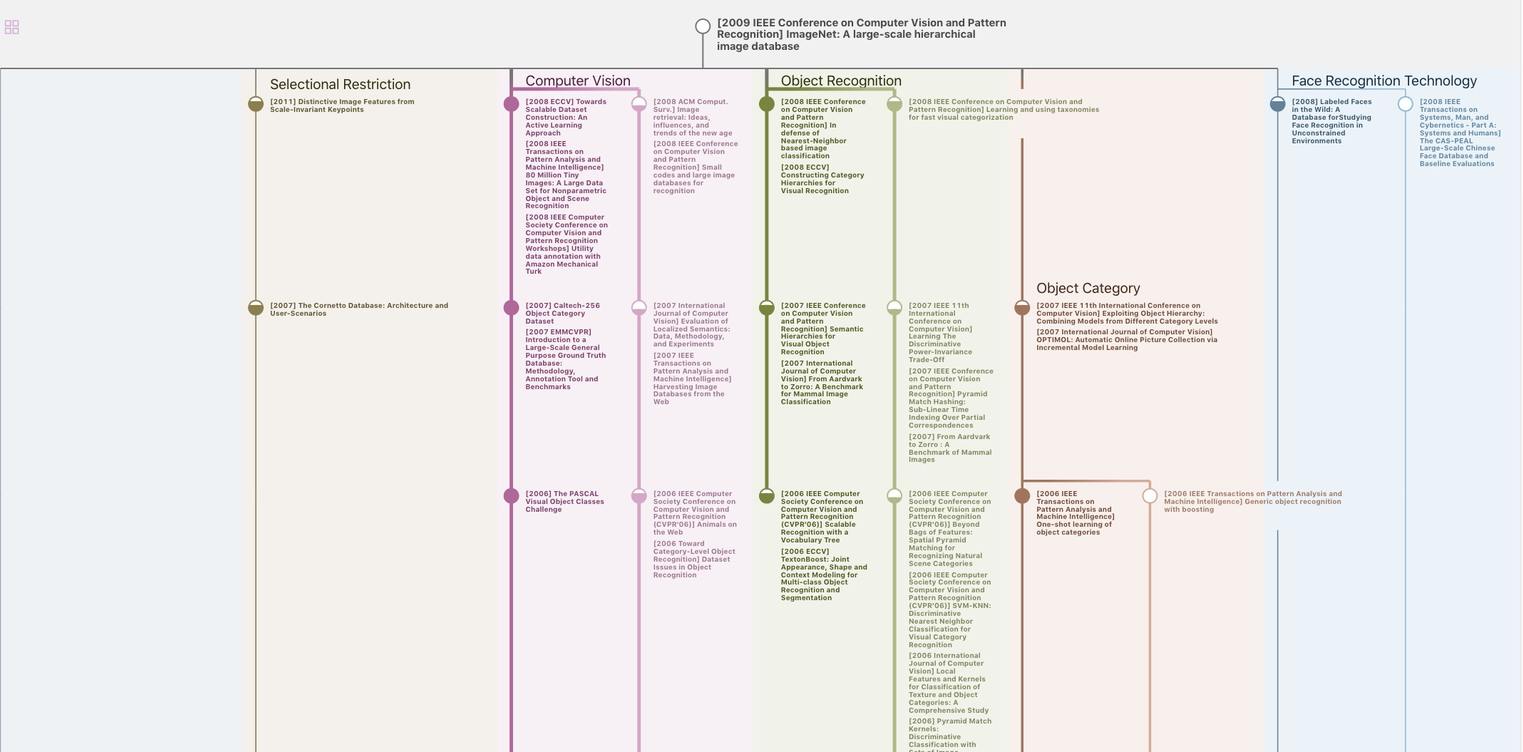
生成溯源树,研究论文发展脉络
Chat Paper
正在生成论文摘要