Contextual MAB Oriented Embedding Denoising for Sequential Recommendation
PROCEEDINGS OF THE 17TH ACM INTERNATIONAL CONFERENCE ON WEB SEARCH AND DATA MINING, WSDM 2024(2024)
摘要
Deep neural networks now have become the de-facto standard for sequential recommendation. In the existing techniques, an embedding vector is assigned for each item, encoding all the characteristics of the latter in latent space. Then, the recommendation is transferred to devising a similarity metric to recommend user's next behavior. Here, we consider each dimension of an embedding vector as a (latent) feature. Though effective, it is unknown which feature carries what semantics toward the item. Actually, in reality, this merit is highly preferable since a specific group of features could induce a particular relation among the items while the others are in vain. Unfortunately, the previous treatment overlooks the feature semantic learning at such a fine-grained level. When each item contains multiple latent aspects, which however is prevalent in real-world, the relations between items are very complex. The existing solutions are easy to fail on better recommendation performance. It is necessary to disentangle the item embeddings and extract credible features in a context-aware manner. To address this issue, in this work, we present a novel Contextual MAB based Embedding Denoising model (Comed for short) to adaptively identify relevant dimension-level features for a better recommendation. Specifically, Comed formulates the embedding denoising task as a Contextual Multi-armed Bandit problem. For each feature of the item embedding, we assign a two-armed neural bandit to determine whether the constituent semantics should be preserved, rendering the whole process as embedding denoising. By aggregating the denoised embeddings as contextual information, a reward function deduced by the similarity between the historical interaction sequence and the target item is further designed to approximate the maximum expected payoffs of bandits for efficient learning. Considering the possible inefficiency of training the serial operating mechanism, we also design a swift learning strategy to accelerate the co-guidance between the renovated sequential embedding and the parallel actions of neural bandits for a better recommendation. Comprehensive trials conducted on four widely recognized benchmarks substantiate the efficiency and efficacy of our framework.
更多查看译文
关键词
Sequential Recommendation,Contextual Multi-Armed Bandit,Representation Denoising
AI 理解论文
溯源树
样例
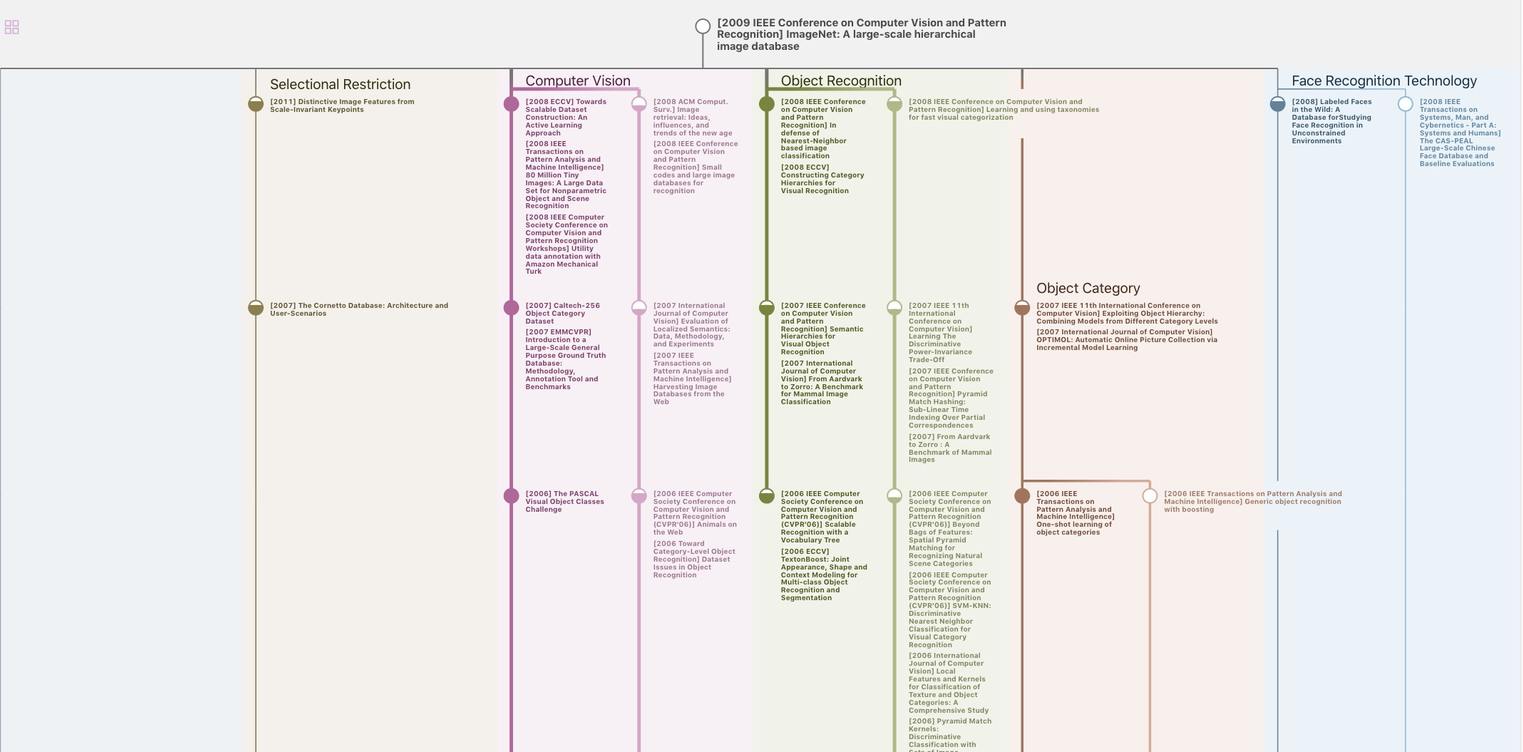
生成溯源树,研究论文发展脉络
Chat Paper
正在生成论文摘要