Negative-Sensitive Framework With Semantic Enhancement for Composed Image Retrieval.
IEEE Trans. Multim.(2024)
摘要
Composed image retrieval is a challenging task in the field of multi-modal learning, aiming at measuring the similarities between target images and query images with modification sentences. Most previous methods either construct feature composition for the query image and modification text or concentrate on extracting cross-modal alignments. However, these methods are prone to neglect the negative impacts of the mismatched correspondences between the hybrid-modal query and target, which could be discriminative when comparing similar instances. Besides, localized textual representations are not fully explored when learning similarities between the query and the target. To overcome the above issues, we propose a Negative-Sensitive Framework with Semantic Enhancement (NSFSE) for mining the adaptive boundaries between matched and mismatched samples with comprehensive consideration of positive and negative correspondences. It can optimize the threshold dynamically based on distributions to explore the intrinsic characteristics of positive and negative correlations, which could further facilitate accurate similarity learning. A text-guided attention mechanism after infusing cross-modal affinities on localized word features is exploited in NSFSE to explore latent semantic-related visual similarity and cross-modal similarity simultaneously. The performance of extensive experiments and comprehensive analysis on three representative datasets CIRR, FashionIQ, and Fashion200 K demonstrate the effectiveness of negative mining of similarity with semantic enhancement in the proposed NSFSE.
更多查看译文
关键词
Composed image retrieval,semantic learning,cross-modal retrieval,distribution learning,attention mechanism
AI 理解论文
溯源树
样例
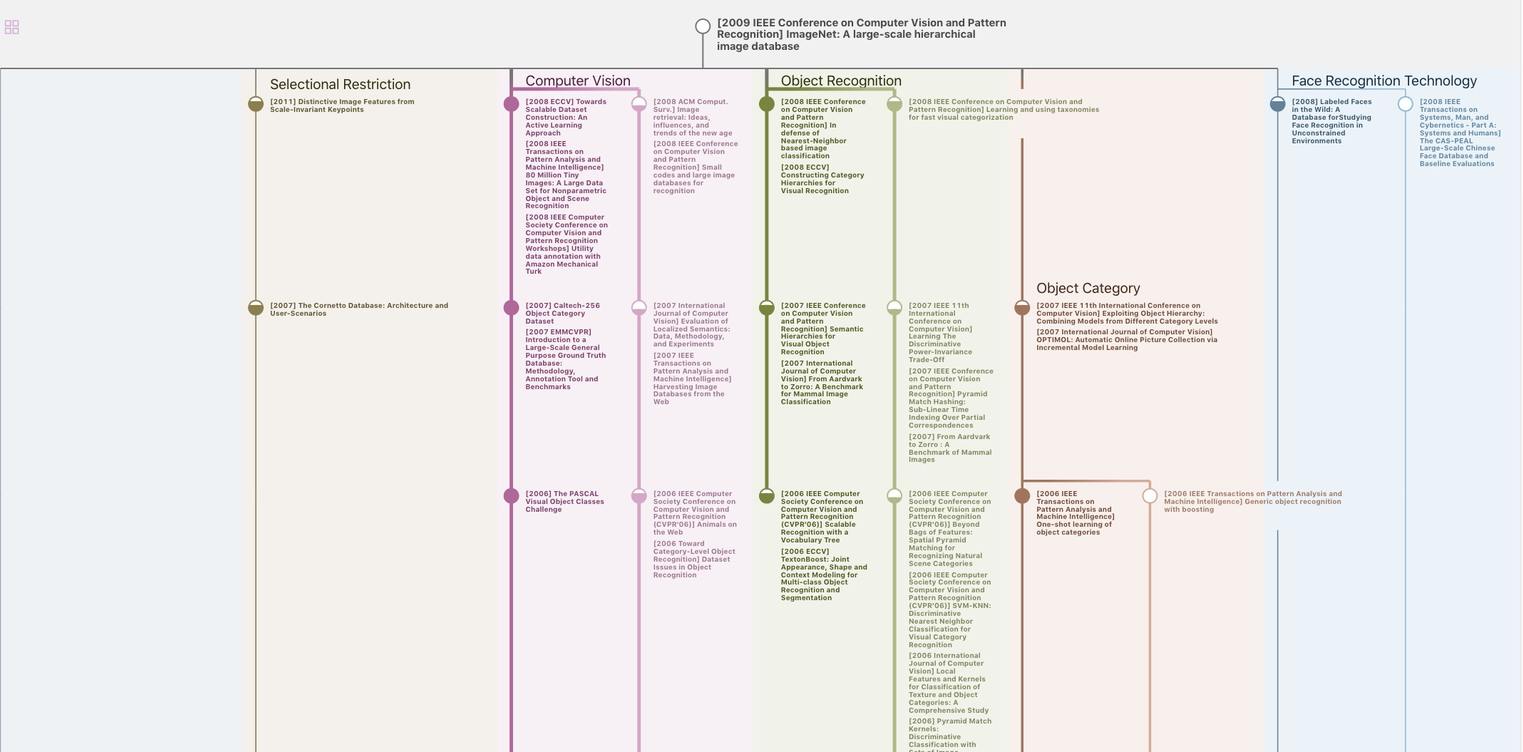
生成溯源树,研究论文发展脉络
Chat Paper
正在生成论文摘要