Privacy-Enhancing Person Re-identification Framework - A Dual-Stage Approach.
IEEE/CVF Winter Conference on Applications of Computer Vision(2024)
摘要
In this work, we show that deep learning-based re-identification (Re-ID) models, albeit trained only with a Re-ID objective (i.e. if two samples belong to the same identity), encode personally identifiable information (PII) in the learned features that may lead to serious privacy concerns. In cognizance of the modern privacy regulations on protecting PII, we propose a novel dual-stage person Re-ID framework that (1) suppresses the PII from the discriminative features, and (2) introduces a controllable privacy mechanism through differential privacy. The former is achieved with a self-supervised de-identification (De-ID) decoder and an adversarial-identity (Adv-ID) module, whereas the latter mechanism leverages a controllable privacy budget to generate a privacy-protected gallery with a Gaussian noise generator. Furthermore, we introduce the notion of a privacy metric to quantify the privacy leakage in Re-ID features which is not explicitly examined in prior work. We demonstrate the feasibility of our approach in achieving a better trade-off between utility and privacy through rigorous experiments on person Re-ID benchmarks.
更多查看译文
关键词
Applications,Social good,Algorithms,Explainable,fair,accountable,privacy-preserving,ethical computer vision,Algorithms,Image recognition and understanding
AI 理解论文
溯源树
样例
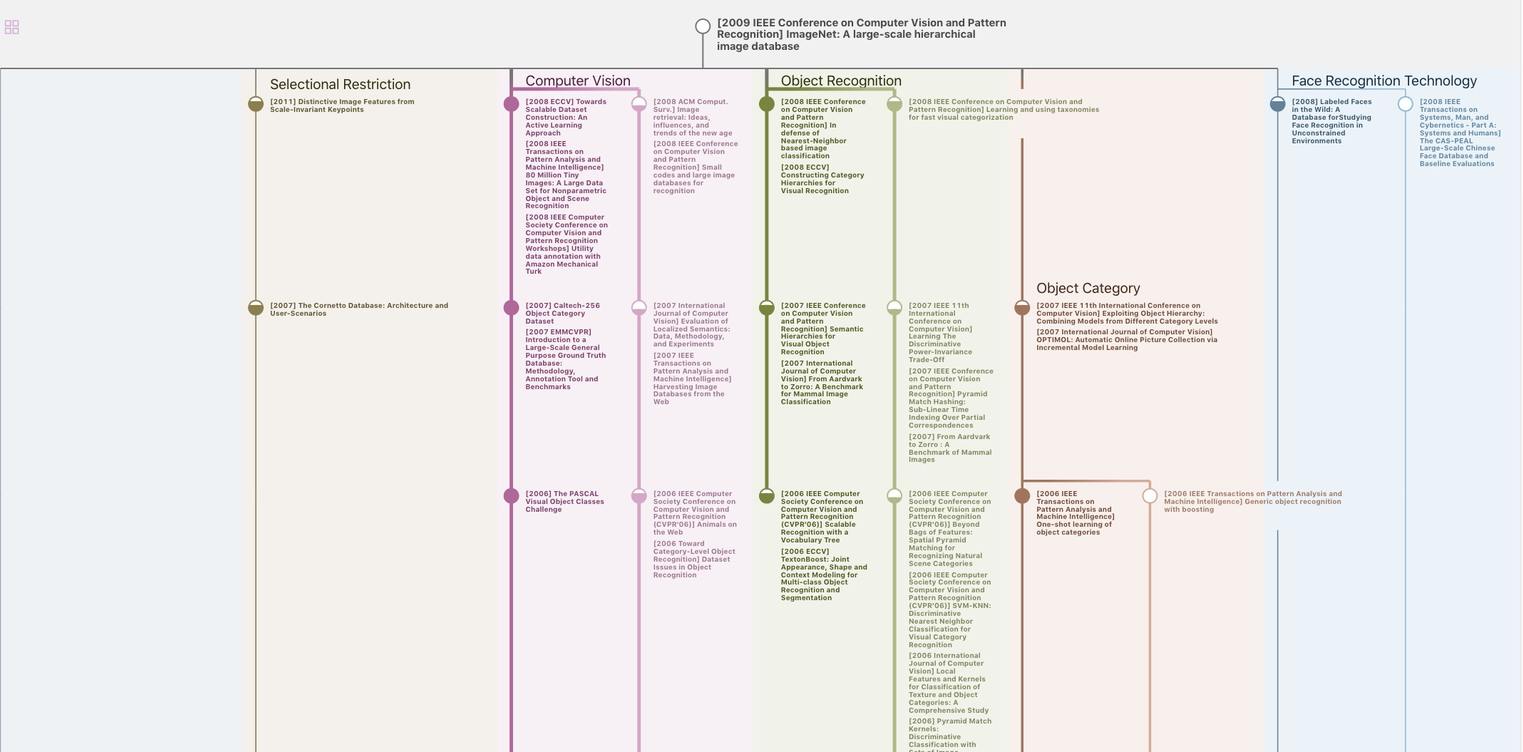
生成溯源树,研究论文发展脉络
Chat Paper
正在生成论文摘要