Improved carbide volume fraction estimation in as-cast HCCI alloys using machine learning techniques
Computational Materials Science(2024)
摘要
An improved approach is presented for the estimation of carbide volume fraction (CVF) in as-cast High Chromium Cast Iron (HCCI) alloys using Machine Learning (ML) techniques. The limitations of existing formulae for CVF estimation in HCCI alloys, which relied on a limited number of alloy compositions, are addressed. A comprehensive dataset comprising 320 distinct alloy compositions from 60 different sources was compiled. ML models trained on this dataset revealed the significant influence of carbon (C), chromium (Cr), and molybdenum (Mo) on CVF determination. By leveraging ML algorithms, a predictive model was developed that offers enhanced accuracy in estimating CVF across a wider range of compositions. This ML-based approach provides researchers with a valuable tool for determining CVF in as-cast HCCI alloys, minimizing the need for resource-intensive and time-consuming experimental procedures. The results obtained demonstrate improved CVF estimation accuracy and broader applicability, thus facilitating more efficient and reliable CVF determination in HCCI alloys.
更多查看译文
关键词
Carbide volume fraction,High chromium cast iron,Machine learning,Metallography,Microstructure,Phase quantification
AI 理解论文
溯源树
样例
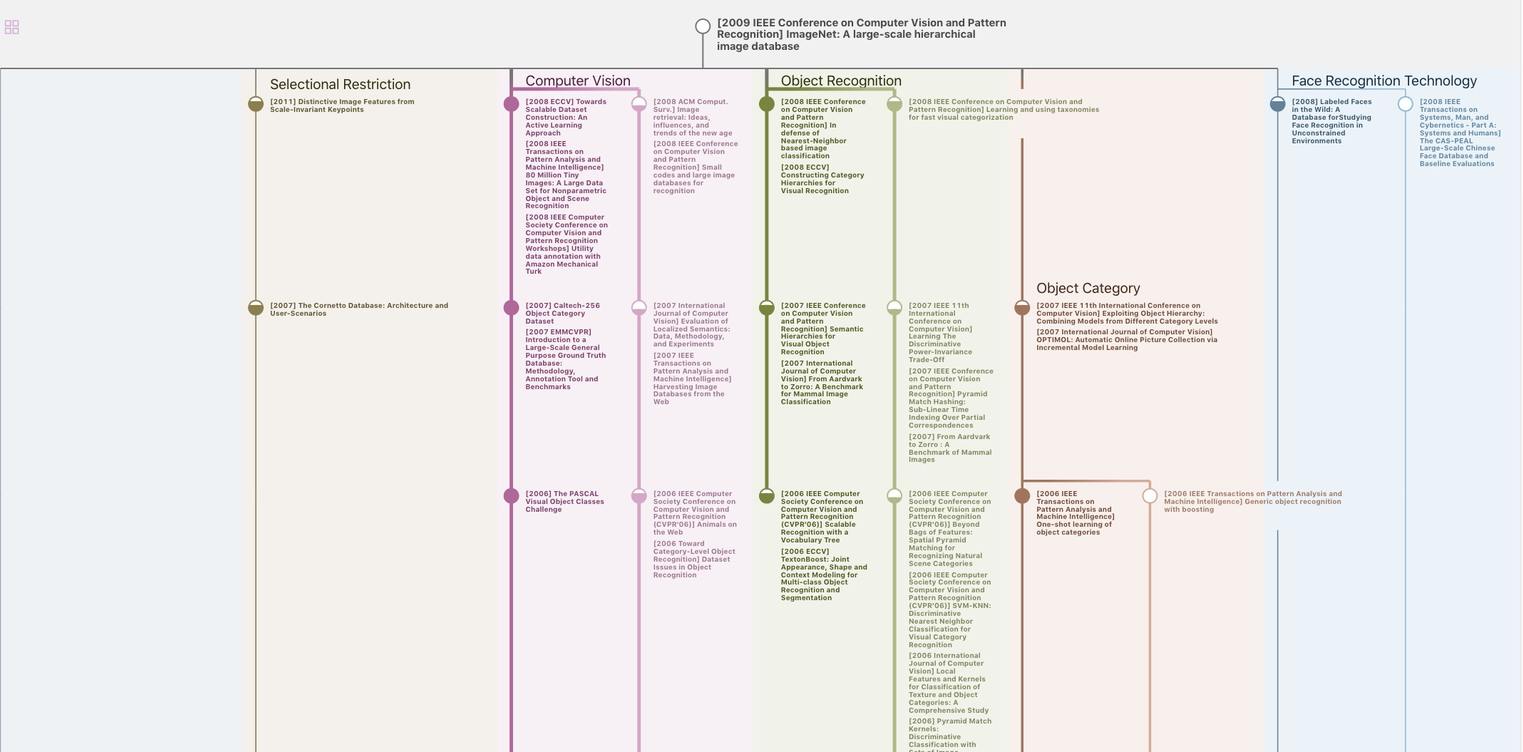
生成溯源树,研究论文发展脉络
Chat Paper
正在生成论文摘要