PD50-01 AI VS. UROLOGISTS: A COMPARATIVE ANALYSIS FOR PROSTATE CANCER DETECTION ON TRANSRECTAL B-MODE ULTRASOUND
JOURNAL OF UROLOGY(2024)
Abstract
You have accessJournal of UrologyProstate Cancer: Detection & Screening V (PD50)1 May 2024PD50-01 AI VS. UROLOGISTS: A COMPARATIVE ANALYSIS FOR PROSTATE CANCER DETECTION ON TRANSRECTAL B-MODE ULTRASOUND Sulaiman Vesal, Indrani Bhattacharya, Hassan Jahanandish, Moonhyung Choi, Steve Ran Zhou, Zachary Kornberg, Elijah Richard Sommer, Richard E. Fan, Mirabela Rusu, and Geoffrey A. Sonn Sulaiman VesalSulaiman Vesal , Indrani BhattacharyaIndrani Bhattacharya , Hassan JahanandishHassan Jahanandish , Moonhyung ChoiMoonhyung Choi , Steve Ran ZhouSteve Ran Zhou , Zachary KornbergZachary Kornberg , Elijah Richard SommerElijah Richard Sommer , Richard E. FanRichard E. Fan , Mirabela RusuMirabela Rusu , and Geoffrey A. SonnGeoffrey A. Sonn View All Author Informationhttps://doi.org/10.1097/01.JU.0001008620.35181.96.01AboutPDF ToolsAdd to favoritesDownload CitationsTrack CitationsPermissionsReprints ShareFacebookLinked InTwitterEmail Abstract INTRODUCTION AND OBJECTIVE: Transrectal ultrasound-guided (TRUS) is widely used to guide prostate biopsy, but its effectiveness is limited due to low sensitivity and signal-to-noise ratio in B-mode TRUS images. While MRI fusion improves prostate biopsy accuracy, only ∼25% of biopsies nationwide are performed following MRI. Instead, most biopsies are still performed using TRUS alone. Better prostate cancer (PCa) detection on B-mode TRUS images could greatly improve biopsy targeting. METHODS: In this study, we developed ProsDectNet, an AI model to detect and localize clinically significant prostate cancer in B-mode TRUS images. ProsDectNet consists of a lesion detection backbone and a false positive reduction component to enhance model performance. We trained and validated ProsDectNet using a cohort of 289 patients (N=110 with a Gleason score ≥7, and N=179 without cancer) who underwent MRI-TRUS fusion targeted biopsy and tested our approach on an independent group of 41 (N=20 with a Gleason score ≥7, and N=21 without cancer) patients. For comparison, we asked three urologist-readers and one radiologist-reader (blinded to MRI or clinical data) to review all test set TRUS images and annotate any areas of suspected cancer with unlimited time. Successful lesion identification was defined as any overlap between AI predictions and ground-truth labels. We computed lesion-level and patient-level sensitivity, specificity, and positive predictive value (PPV) to compare ProsDectNet performance vs. readers. RESULTS: ProsDectNet demonstrated a patient-level sensitivity and specificity of 74.0% and 67.0%, and lesion-level sensitivity and specificity of 66.0% and 90.0%, respectively, surpassing the average urologist's results (50.0% sensitivity, 70.0% specificity, 43.0% lesion-level sensitivity, and 91.0% specificity). Additionally, its patient-level PPV of 67.0% outperformed the average urologists' PPV of 62.0%. CONCLUSIONS: Our study developed a high-performing AI model to identify cancer on TRUS images. The model outperformed urologists in detecting PCa. This emphasizes its potential for improving biopsy guidance with B-mode ultrasound, especially in scenarios where MRI was unavailable. Ongoing research aims to validate and enhance our approach through expanded datasets and real-world clinical evaluations. Download PPT Source of Funding: Departments of Radiology and Urology, Stanford University, National Cancer Institute of the National Institutes of Health (R37CA260346 to M.R), and the generous philanthropic support of our patients (G.S.). The content is solely the responsibility of the authors and does not necessarily represent the official views of the National Institutes of Health © 2024 by American Urological Association Education and Research, Inc.FiguresReferencesRelatedDetails Volume 211Issue 5SMay 2024Page: e1056 Advertisement Copyright & Permissions© 2024 by American Urological Association Education and Research, Inc.Metrics Author Information Sulaiman Vesal More articles by this author Indrani Bhattacharya More articles by this author Hassan Jahanandish More articles by this author Moonhyung Choi More articles by this author Steve Ran Zhou More articles by this author Zachary Kornberg More articles by this author Elijah Richard Sommer More articles by this author Richard E. Fan More articles by this author Mirabela Rusu More articles by this author Geoffrey A. Sonn More articles by this author Expand All Advertisement PDF downloadLoading ...
MoreTranslated text
Key words
Ultrasound Contrast Agents
AI Read Science
Must-Reading Tree
Example
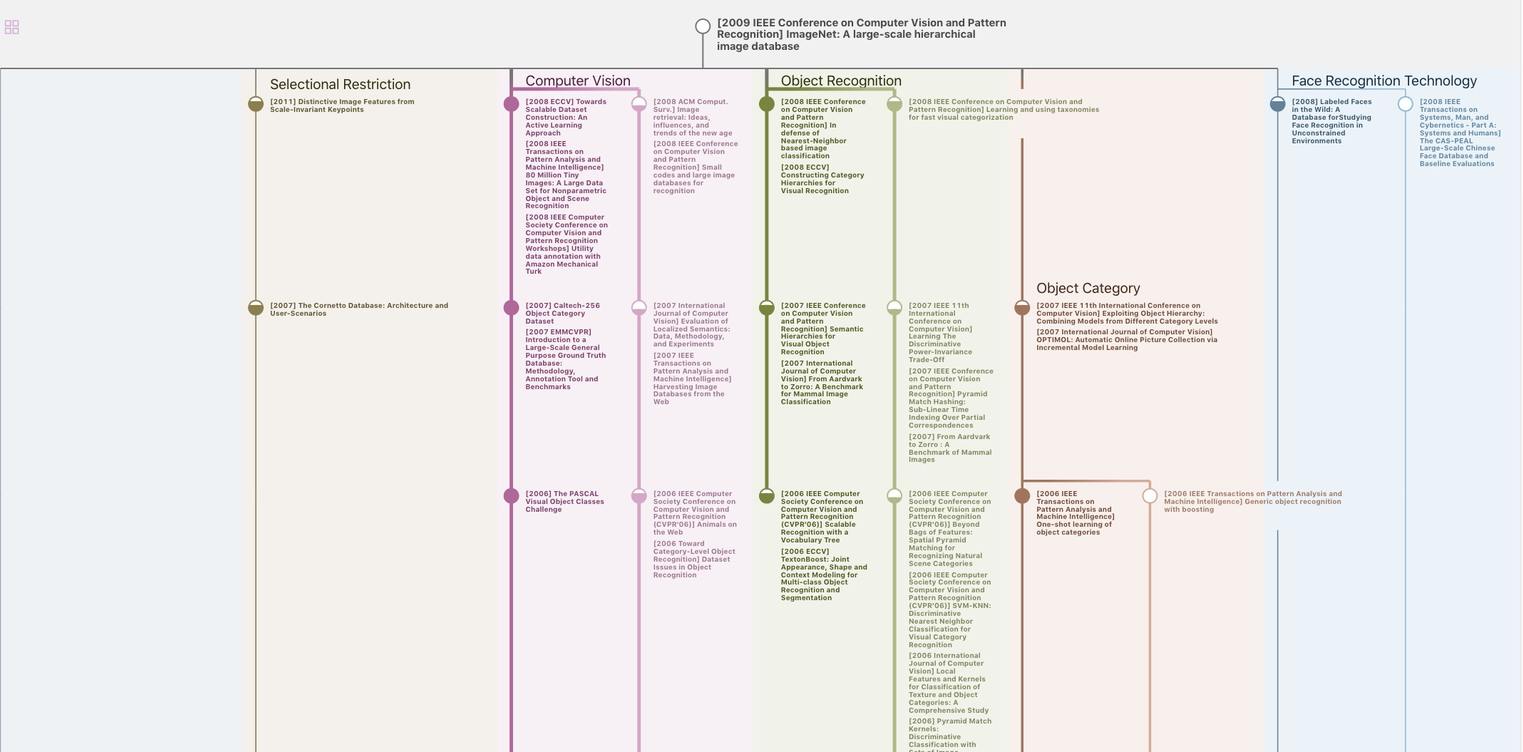
Generate MRT to find the research sequence of this paper
Chat Paper
Summary is being generated by the instructions you defined