LaDiC: Are Diffusion Models Really Inferior to Autoregressive Counterparts for Image-to-Text Generation?
NAACL-HLT(2024)
摘要
Diffusion models have exhibited remarkable capabilities in text-to-imagegeneration. However, their performance in image-to-text generation,specifically image captioning, has lagged behind Auto-Regressive (AR) models,casting doubt on their applicability for such tasks. In this work, we revisitdiffusion models, highlighting their capacity for holistic context modeling andparallel decoding. With these benefits, diffusion models can alleviate theinherent limitations of AR methods, including their slow inference speed, errorpropagation, and unidirectional constraints. Furthermore, we identify the priorunderperformance of diffusion models stemming from the absence of an effectivelatent space for image-text alignment, and the discrepancy between continuousdiffusion processes and discrete textual data. In response, we introduce anovel architecture, LaDiC, which utilizes a split BERT to create a dedicatedlatent space for captions and integrates a regularization module to managevarying text lengths. Our framework also includes a diffuser for semanticimage-to-text conversion and a Back Refine technique to enhance tokeninteractivity during inference. LaDiC achieves state-of-the-art performance fordiffusion-based methods on the MS COCO dataset with 38.2 BLEU@4 and 126.2CIDEr, demonstrating exceptional performance without pre-training or ancillarymodules. This indicates strong competitiveness with AR models, revealing thepreviously untapped potential of diffusion models in image-to-text generation.
更多查看译文
AI 理解论文
溯源树
样例
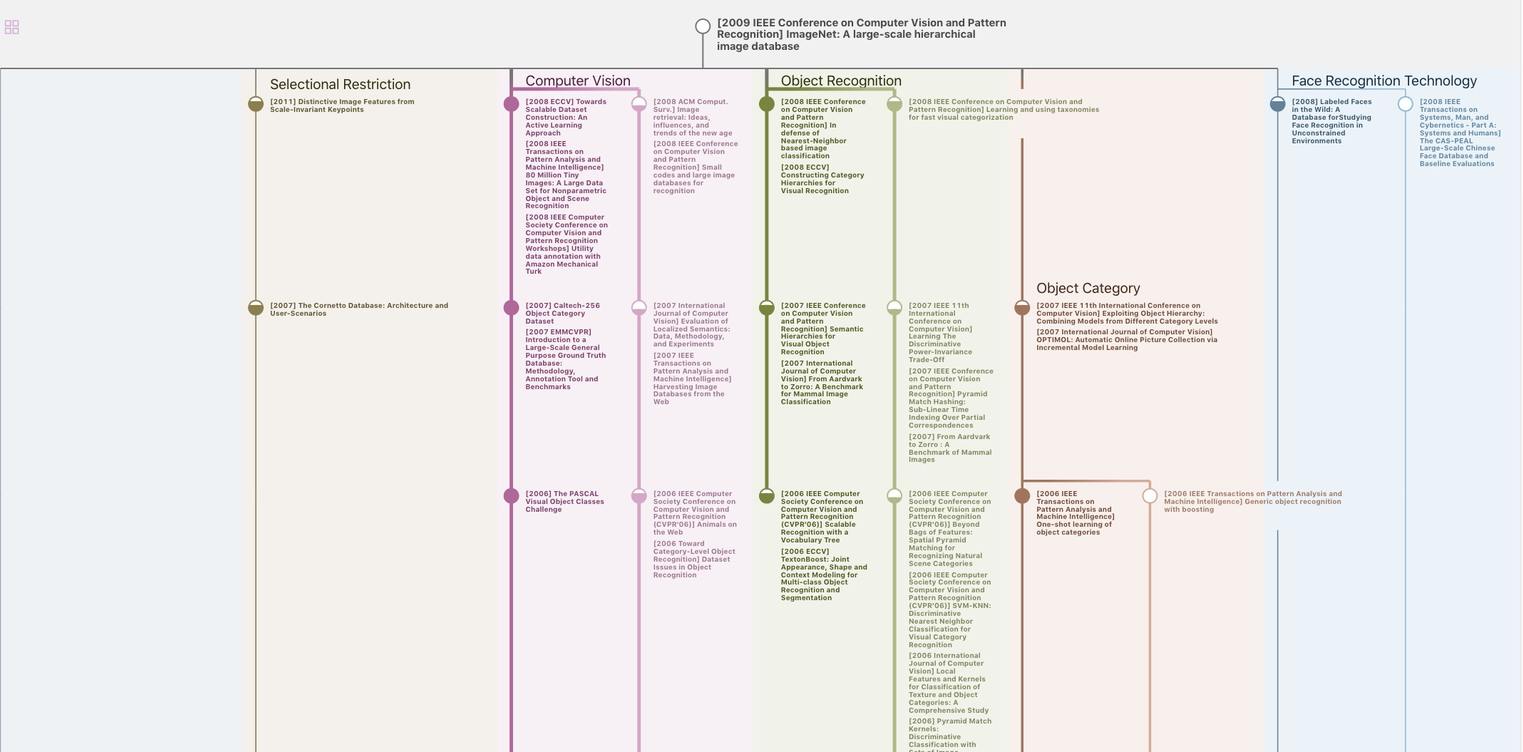
生成溯源树,研究论文发展脉络
Chat Paper
正在生成论文摘要