Zookt: Task-Adaptive Knowledge Transfer of Model Zoo for Few-Shot Learning
Pattern Recognition(2024)
摘要
Few-shot learning (FSL) aims to recognize novel classes with few examples. It is challenging since it suffers from a data scarcity issue. Although existing methods have shown superior performance, they neglect some pretrained model priors from various datasets/tasks, which are usually free or cheap to collect from some open source platforms (e.g., Github). Inspired by this, in this paper, we focus on a new FSL setting, namely, Model-Zoo-based FSL (MZFSL), which transfers knowledge learned from not only base classes but also a zoo of deep models (called Model Zoo) pretrained on various tasks/datasets. To fully exploit the Model Zoo avoiding the risk of overfitting, we propose a novel knowledge transfer framework, called ZooKT, which amalgamates knowledge of model zoo, instead of finetuning the model zoo, to learn to extract transferable visual features for novel classes. Specifically, we first regard the model zoo as a prior of feature extractor. Then a meta weight prediction network is designed to leverage the prior to predict the weights of target feature extractor for novel classes. Finally, we integrate the target feature extractor to existing FSL methods for performing novel class prediction. Experimental results on few-shot classification and detection scenarios show that our ZooKT outperforms not only single-model finetuning methods but also state-of-the-art multiple-model transfer learning methods, with comparable inference time with a single model.
更多查看译文
关键词
Few-shot learning,Meta-learning,Model ZOO
AI 理解论文
溯源树
样例
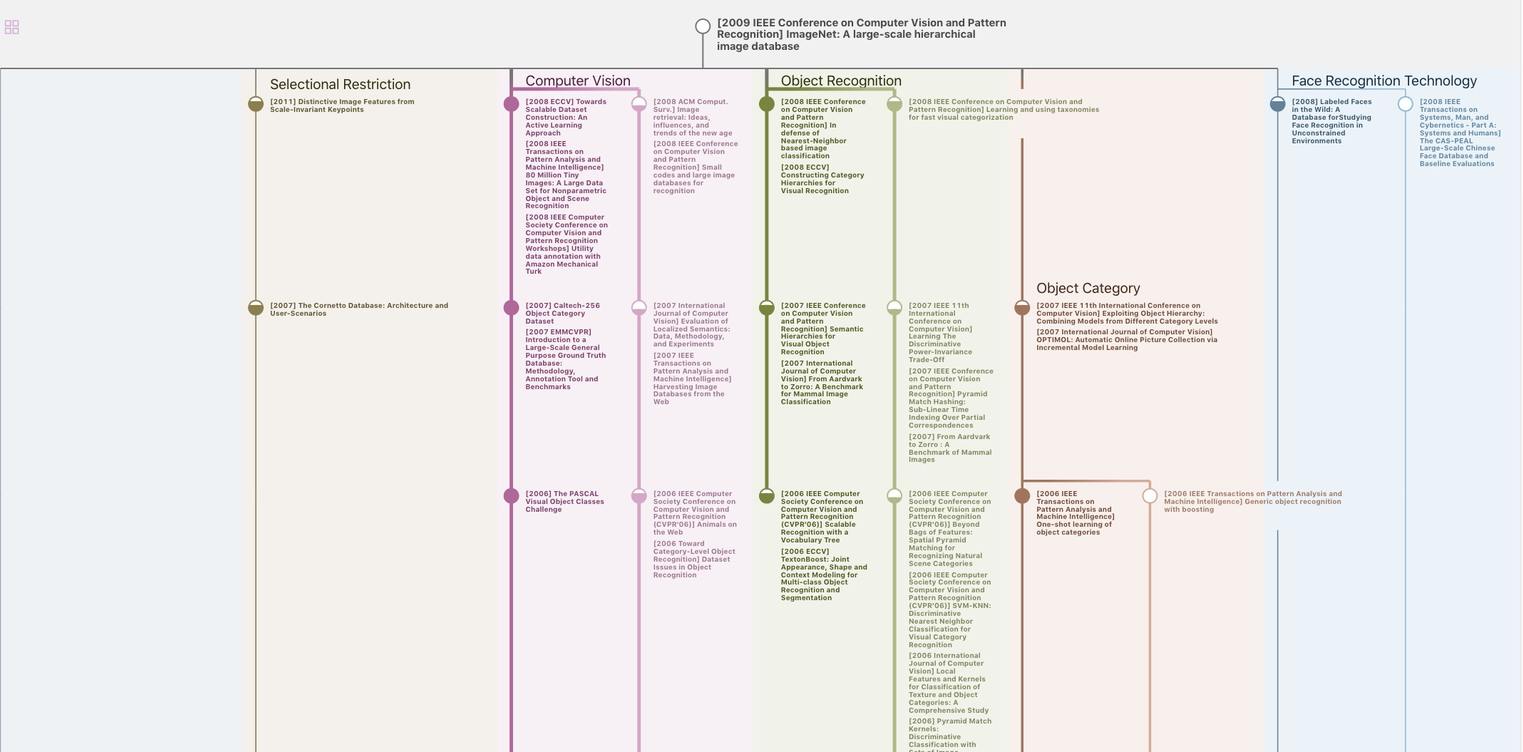
生成溯源树,研究论文发展脉络
Chat Paper
正在生成论文摘要