DPTraj-PM: Differentially Private Trajectory Synthesis Using Prefix Tree and Markov Process
CoRR(2024)
摘要
The increasing use of GPS-enabled devices has generated a large amount of
trajectory data. These data offer us vital insights to understand the movements
of individuals and populations, benefiting a broad range of applications from
transportation planning to epidemic modeling. However, improper release of
trajectory data is increasing concerns on individual privacy. Previous attempts
either lack strong privacy guarantees, or fail to preserve sufficient basic
characteristics of the original data. In this paper, we propose DPTraj-PM, a
method to synthesize trajectory dataset under the differential privacy (DP)
framework while ensures high data utility. Based on the assumption that an
individual's trajectory could be mainly determined by the initial trajectory
segment (which depicts the starting point and the initial direction) and the
next location point, DPTraj-PM discretizes the raw trajectories into
neighboring cells, and models them by combining a prefix tree structure and an
m-order Markov process. After adding noise to the model under differential
privacy, DPTraj-PM generates a synthetic dataset from the noisy model to enable
a wider spectrum of data mining and modeling tasks. The output traces crafted
by DPTraj-PM not only preserves the patterns and variability in individuals'
mobility behaviors, but also protects individual privacy. Experiments on two
real-world datasets demonstrate that DPTraj-PM substantially outperforms the
state-of-the-art techniques in terms of data utility. Our code is available at
https://github.com/wnn5/DP-PrefixTreeMarkov.
更多查看译文
AI 理解论文
溯源树
样例
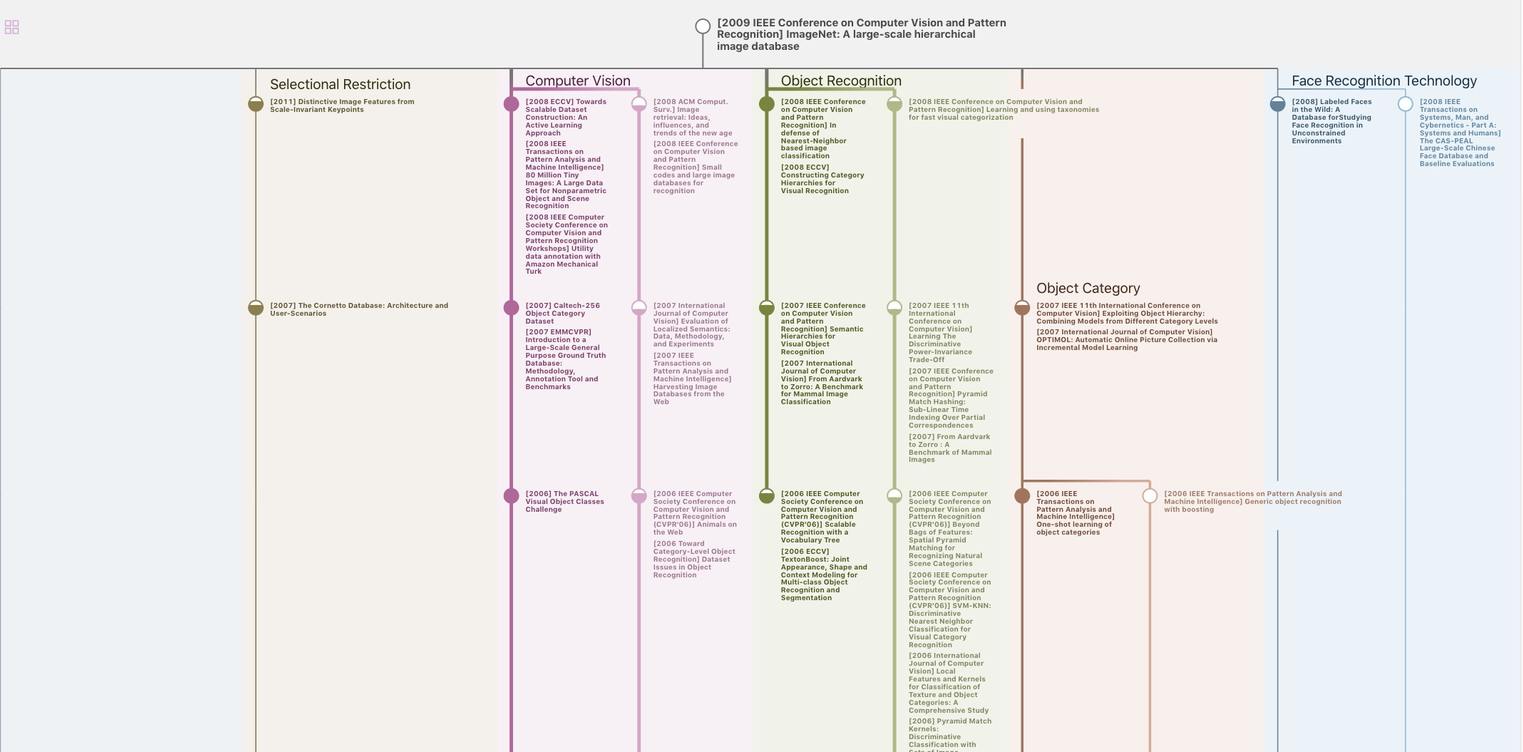
生成溯源树,研究论文发展脉络
Chat Paper
正在生成论文摘要